Journal of Risk Model Validation
ISSN:
1753-9579 (print)
1753-9587 (online)
Editor-in-chief: Steve Satchell
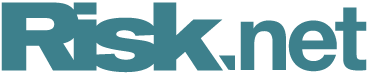
A model combining Optuna and the light gradient-boosting machine algorithm for credit default forecasting
Xinyong Lu, Yuchong Li, Jiaxin Wang, Xuewei Liu and Jiahui Wei
Need to know
- The study introduces a new credit default prediction model called Opt_LightGBM to tackle the difficulties associated with analyzing real-world bank loan data.
- The paper employs the Borderline-SMOTE sampling technique to address data imbalance, helping improve the performance of the prediction model.
- The Opt_LightGBM model utilizes the Optuna parameter tuning tool to enhance the efficiency of parameter optimization and improve the predictive accuracy of the LightGBM model.
- Results show that the model achieves an accuracy of 88%, an AUC of 0.9, and both recall rate and F1 score exceeding 0.88, demonstrating its effectiveness in predicting credit default and aiding risk management.
Copyright Infopro Digital Limited. All rights reserved.
As outlined in our terms and conditions, https://www.infopro-digital.com/terms-and-conditions/subscriptions/ (point 2.4), printing is limited to a single copy.
If you would like to purchase additional rights please email info@risk.net
Copyright Infopro Digital Limited. All rights reserved.
You may share this content using our article tools. As outlined in our terms and conditions, https://www.infopro-digital.com/terms-and-conditions/subscriptions/ (clause 2.4), an Authorised User may only make one copy of the materials for their own personal use. You must also comply with the restrictions in clause 2.5.
If you would like to purchase additional rights please email info@risk.net