Journal of Risk Model Validation
ISSN:
1753-9579 (print)
1753-9587 (online)
Editor-in-chief: Steve Satchell
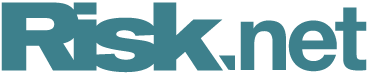
Backtesting of a probability of default model in the point-in-time–through-the-cycle context
Need to know
- We claim PD model correctness is equivalent to unbiasedness; given unbiasedness, calibration accuracy – as an attribute reflecting the magnitude of estimation errors – determines model acceptability. Hence, the back-testing scope includes (i) calibration testing aimed at establishing unbiasedness; and (ii) measuring calibration accuracy.
- Unbiasedness in a PIT–TTC-calibrated PD model can take three different forms. The traditional tests, such as Binomial and chi-squared, look at its strictest form, which ignores the presence of estimation errors. We propose alternative tests and explain how PIT-based results can be used to draw conclusions about TTC and LTA PDs.
- We argue calibration accuracy is equivalent to discriminatory power, ie, the rating function’s ability to rank-order obligors according to their true PDs. Referring to the existing literature, we show how the traditional measures of discriminatory power are unfit for that purpose, and propose a modification that turns the classic AUC into a true measure of calibration accuracy.
Abstract
This paper presents a backtesting framework for a probability of default (PD) model, assuming that the latter is calibrated to both point-in-time (PIT) and through-the-cycle (TTC) levels. We claim that the backtesting scope includes both calibration testing, to establish the unbiasedness of the PIT PD estimator, and measuring calibration accuracy, which, according to our definition, reflects the magnitude of the PD estimation error. We argue that model correctness is equivalent to unbiasedness, while accuracy, being a measure of estimation efficiency, determines model acceptability. We explain how the PIT-based test results may be used to draw conclusions about the associated TTC PDs. We discover that unbiasedness in the PIT–TTC context can take three different forms, and show how the popular binomial and chi-squared tests focus on its strictest form, which does not allow for estimation errors. We offer alternative tests and confirm their usefulness in Monte Carlo simulations. Further, we argue that accuracy is tightly connected to the ranking ability of the underlying rating function and that these two properties can be characterized by a single measure. After considering today’s measures of risk differentiation, which claim to describe the ranking ability, we dismiss them and conclude that they are unfit for purpose. We then propose a modification of one traditional risk differentiation measure, namely, the area under the receiving-operator-characteristic curve (AUC), that makes the result a measure of calibration accuracy, and hence also of the ranking ability.
Copyright Infopro Digital Limited. All rights reserved.
As outlined in our terms and conditions, https://www.infopro-digital.com/terms-and-conditions/subscriptions/ (point 2.4), printing is limited to a single copy.
If you would like to purchase additional rights please email info@risk.net
Copyright Infopro Digital Limited. All rights reserved.
You may share this content using our article tools. As outlined in our terms and conditions, https://www.infopro-digital.com/terms-and-conditions/subscriptions/ (clause 2.4), an Authorised User may only make one copy of the materials for their own personal use. You must also comply with the restrictions in clause 2.5.
If you would like to purchase additional rights please email info@risk.net