Journal of Risk Model Validation
ISSN:
1753-9579 (print)
1753-9587 (online)
Editor-in-chief: Steve Satchell
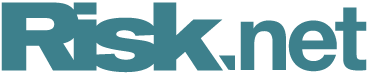
An advanced hybrid classification technique for credit risk evaluation
Chong Wu, Dekun Gao, Qianqun Ma, Qi Wang and Yu Lu
Need to know
- We find a new hybrid classifier based in DBN to credit evaluation.
- Our research finds the new model is better than some traditional base classifiers with a German and Australian credit dataset.
- The model has a better performance in the imbalanced German dataset than balanced Australian dataset.
Abstract
Due to the important role that credit risk evaluation (CRE) plays in banks, it is crucial that we improve the accuracy of the CRE model. In this paper, we employ a hybrid approach to design a practical and effective CRE model based on a deep belief network (DBN) and the K-means method. First, using the K-means method, we preprocess the original data set and reclassify the unrepresentative data into high grades. Second, based on the results of our K-means method analysis, a DBN is used to construct a CRE model. We randomly select data in fixed proportions from the samples that have been assigned to new classes in order to train and test the DBN model. Finally, German and Australian credit data sets are applied to the proposed model. Our results show that the hybrid classifier we propose is effective in CRE and performs significantly better than classical CRE models.
Copyright Infopro Digital Limited. All rights reserved.
As outlined in our terms and conditions, https://www.infopro-digital.com/terms-and-conditions/subscriptions/ (point 2.4), printing is limited to a single copy.
If you would like to purchase additional rights please email info@risk.net
Copyright Infopro Digital Limited. All rights reserved.
You may share this content using our article tools. As outlined in our terms and conditions, https://www.infopro-digital.com/terms-and-conditions/subscriptions/ (clause 2.4), an Authorised User may only make one copy of the materials for their own personal use. You must also comply with the restrictions in clause 2.5.
If you would like to purchase additional rights please email info@risk.net