Journal of Operational Risk
ISSN:
1744-6740 (print)
1755-2710 (online)
Editor-in-chief: Marcelo Cruz
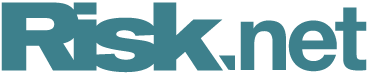
Need to know
- We investigate stability of operational risk capital models by varying the severity distribution, sample-size and heaviness of loss data.
- Fitting moderate loss data to 100 losses above 100k EUR leads to relative OpVaR standard deviations of 30% (Weibull) to and 3437% (generalized beta of the second kind), depending on the severity distribution.
- Clear stability winners are the Weibull and lognormal distributions, while the exibility of the generalized beta distribution of the second kind comes at a high price for stability.
- Heavier loss data do in general require larger sample-sizes for comparable stability, but the trend is not strictly monotonic.
Abstract
The challenge of using small sample sizes for operational risk capital models fitted via maximum likelihood estimation is well recognized, yet the literature generally provides warning examples rather than a systematic approach. We present a general framework for analyzing maximum likelihood estimation error on operational value- at-risk as a function of sample size for five severity distributions commonly used in operational risk capital models. More specifically, we study the estimation error along three dimensions: the choice of severity distribution, the sample size and the heaviness of the underlying losses. We apply these results to model selection and explore implications for operational risk modeling.
Copyright Infopro Digital Limited. All rights reserved.
As outlined in our terms and conditions, https://www.infopro-digital.com/terms-and-conditions/subscriptions/ (point 2.4), printing is limited to a single copy.
If you would like to purchase additional rights please email info@risk.net
Copyright Infopro Digital Limited. All rights reserved.
You may share this content using our article tools. As outlined in our terms and conditions, https://www.infopro-digital.com/terms-and-conditions/subscriptions/ (clause 2.4), an Authorised User may only make one copy of the materials for their own personal use. You must also comply with the restrictions in clause 2.5.
If you would like to purchase additional rights please email info@risk.net