Journal of Energy Markets
ISSN:
1756-3607 (print)
1756-3615 (online)
Editor-in-chief: Derek W. Bunn
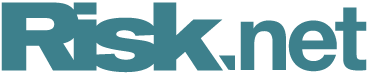
Neural network middle-term probabilistic forecasting of daily power consumption
Need to know
- Middle-term horizon (months to a year) power consumption prediction.
- A new modelling approach that incorporates trend, seasonality and weather conditions, as explicative variables in a shallow neural network with an autoregressive feature.
- Excellent results for density forecast on the one-year test set applying it to the daily power consumption in New England, US.
Abstract
Middle-term horizon (months to a year) power consumption prediction is a major challenge in the energy sector, particularly when probabilistic forecasting is considered. We propose a new modeling approach that incorporates trend, seasonality and weather conditions as explicative variables in a shallow neural network with an autoregressive feature. Applying it to the daily power consumption in New England, we obtain excellent results for the density forecast on the one-year test set. We verified the quality of the power consumption probabilistic forecasting achieved not only by comparing the results with other standard models for density forecasting but also by considering measures that are frequently used in the energy sector, such as the pinball loss function and confidence interval backtesting.
Copyright Infopro Digital Limited. All rights reserved.
As outlined in our terms and conditions, https://www.infopro-digital.com/terms-and-conditions/subscriptions/ (point 2.4), printing is limited to a single copy.
If you would like to purchase additional rights please email info@risk.net
Copyright Infopro Digital Limited. All rights reserved.
You may share this content using our article tools. As outlined in our terms and conditions, https://www.infopro-digital.com/terms-and-conditions/subscriptions/ (clause 2.4), an Authorised User may only make one copy of the materials for their own personal use. You must also comply with the restrictions in clause 2.5.
If you would like to purchase additional rights please email info@risk.net