Journal of Credit Risk
ISSN:
1744-6619 (print)
1755-9723 (online)
Editor-in-chief: Linda Allen and Jens Hilscher
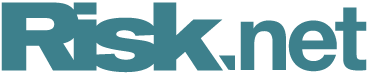
Markov chain Monte Carlo estimation of default and recovery: dependent via the latent systematic factor
Xiaolin Luo and Pavel V. Shevchenko
Abstract
ABSTRACT
It is a well-known fact that recovery rates tend to decrease when the number of defaults increases during economic downturns. We demonstrate how the loss given default model with the default and recovery dependent via the latent systematic risk factor can be estimated using Bayesian inference methodology and the Markov chain Monte Carlo method. This approach is very convenient for joint estimation of all model parameters and latent systematic factors. Moreover, all relevant uncertainties are easily quantified. Typically available data is the annual averages of defaults and recoveries and thus the data sets are small and parameter uncertainty is significant. In this case, the Bayesian approach is superior to the maximum likelihood method, which relies on a large-sample limit Gaussian approximation for the parameter uncertainty. As an example, we consider a homogeneous portfolio with one latent factor. However, the approach can be easily extended to deal with nonhomogenous portfolios and several latent factors.
Copyright Infopro Digital Limited. All rights reserved.
As outlined in our terms and conditions, https://www.infopro-digital.com/terms-and-conditions/subscriptions/ (point 2.4), printing is limited to a single copy.
If you would like to purchase additional rights please email info@risk.net
Copyright Infopro Digital Limited. All rights reserved.
You may share this content using our article tools. As outlined in our terms and conditions, https://www.infopro-digital.com/terms-and-conditions/subscriptions/ (clause 2.4), an Authorised User may only make one copy of the materials for their own personal use. You must also comply with the restrictions in clause 2.5.
If you would like to purchase additional rights please email info@risk.net