Journal of Computational Finance
ISSN:
1460-1559 (print)
1755-2850 (online)
Editor-in-chief: Christoph Reisinger
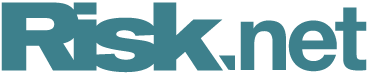
Robust product Markovian quantization
Ralph Rudd, Thomas A. McWalter, Jörg Kienitz and Eckhard Platen
Need to know
- We reformulate recursive Marginal quantization and product Markovian quantization as standard vector quantization.
- This allows the specification of an adaptive, robust algorithm in terms of the Newton–Raphson method and the accelerated Lloyd's algorithm.
- We propose more accurate higher-order updates for stochastic volatility/variance models.
- We apply the robust algorithm to various models and perform a calibration to American options on a stock.
Abstract
Recursive marginal quantization (RMQ) allows the construction of optimal discrete grids for approximating solutions to stochastic differential equations in d dimensions. Product Markovian quantization (PMQ) reduces this problem to d one-dimensional quantization problems by recursively constructing product quantizers, as opposed to a truly optimal quantizer. However, the standard Newton–Raphson method used in the PMQ algorithm suffers from numerical instabilities, inhibiting widespread adoption, especially for use in calibration. By directly specifying the random variable to be quantized at each time step, we show that PMQ, and RMQ in one dimension, can be expressed as standard vector quantization. This reformulation allows the application of the accelerated Lloyd algorithm in an adaptive and robust procedure. Further, in the case of stochastic volatility models, we extend the PMQ algorithm by using higher-order updates for the volatility or variance process. We illustrate the technique for European options using the Heston model, and more exotic products using the stochastic alpha–beta–rho (SABR) model.
Copyright Infopro Digital Limited. All rights reserved.
You may share this content using our article tools. Printing this content is for the sole use of the Authorised User (named subscriber), as outlined in our terms and conditions - https://www.infopro-insight.com/terms-conditions/insight-subscriptions/
If you would like to purchase additional rights please email info@risk.net
Copyright Infopro Digital Limited. All rights reserved.
You may share this content using our article tools. Copying this content is for the sole use of the Authorised User (named subscriber), as outlined in our terms and conditions - https://www.infopro-insight.com/terms-conditions/insight-subscriptions/
If you would like to purchase additional rights please email info@risk.net