Journal of Computational Finance
ISSN:
1460-1559 (print)
1755-2850 (online)
Editor-in-chief: Christoph Reisinger
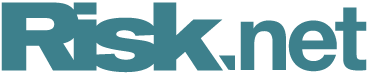
An artificial neural network representation of the SABR stochastic volatility model
Need to know
- The paper demonstrates the effectiveness of training an artificial neural network (ANN) to represent different implementations of the SABR stochastic volatility model – from the widely used SABR approximation to a full two-factor PDE implementation.
- With respect to representing the two-factor PDE implementation the approach demonstrates significant performance acceleration whilst retaining a high degree of accuracy.
- The method allows for richer models, which are currently too computationally expensive for practical purposes, to have ANN representations and be used effectively.
Abstract
In this paper, the universal approximation theorem of artificial neural networks (ANNs) is applied to the stochastic alpha beta rho (SABR) stochastic volatility model in order to construct highly efficient representations. Initially, the SABR approximation of Hagan et al is considered, followed by the more accurate integration scheme of McGhee as well as a two-factor finite-difference scheme. The resulting ANN calculates 10 000 times faster than the finite-difference scheme while maintaining a high degree of accuracy. As a result, the ANN dispenses with the need for the commonly used SABR approximation.
Copyright Infopro Digital Limited. All rights reserved.
As outlined in our terms and conditions, https://www.infopro-digital.com/terms-and-conditions/subscriptions/ (point 2.4), printing is limited to a single copy.
If you would like to purchase additional rights please email info@risk.net
Copyright Infopro Digital Limited. All rights reserved.
You may share this content using our article tools. As outlined in our terms and conditions, https://www.infopro-digital.com/terms-and-conditions/subscriptions/ (clause 2.4), an Authorised User may only make one copy of the materials for their own personal use. You must also comply with the restrictions in clause 2.5.
If you would like to purchase additional rights please email info@risk.net