Journal of Computational Finance
ISSN:
1460-1559 (print)
1755-2850 (online)
Editor-in-chief: Christoph Reisinger
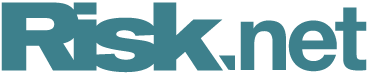
Need to know
- Propose a tail-based normal approximation for ES estimation.
- Develop an adjusted tail-based normal ES estimation approach for heavy-tailed distributions.
- The approach is easy to implement and robust for various heavy-tailed distributions.
- The approach is substantially accurate and works well for small samples.
Abstract
In risk management, estimating expected shortfall, though important and indispensable, is difficult when the sample size is small. This paper suggests a recipe for meeting such a challenge. A tail-based normal approximation with explicit formulas is derived by matching a specific quantile and the mean excess square of the sample observations. To enhance the estimation accuracy, we propose an adjusted tail-based normal approximation based on the sample's tail weight. The adjusted expected shortfall estimator is robust and efficient in the sense that it can be applied to various heavy-tailed distributions, such as Student t, lognormal, Gamma and Weibull, and the errors are all small. Moreover, compared with two common expected shortfall estimators -- the arithmetic average of excessive losses and extreme value theory estimator -- the proposed estimator achieves smaller mean squared errors for small samples, especially at high confidence levels. The properties of linear transformations on the expected shortfall estimator are also investigated to ensure its practicality.
Copyright Infopro Digital Limited. All rights reserved.
As outlined in our terms and conditions, https://www.infopro-digital.com/terms-and-conditions/subscriptions/ (point 2.4), printing is limited to a single copy.
If you would like to purchase additional rights please email info@risk.net
Copyright Infopro Digital Limited. All rights reserved.
You may share this content using our article tools. As outlined in our terms and conditions, https://www.infopro-digital.com/terms-and-conditions/subscriptions/ (clause 2.4), an Authorised User may only make one copy of the materials for their own personal use. You must also comply with the restrictions in clause 2.5.
If you would like to purchase additional rights please email info@risk.net