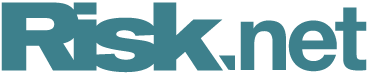
This article was paid for by a contributing third party.More Information.
The fine line with LLMs: financial institutions’ cautious embrace of AI
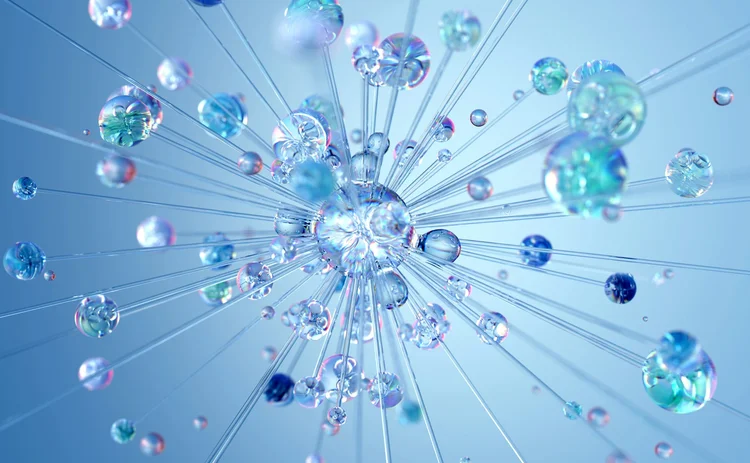
The integration of large language models (LLMs) has emerged as a pivotal force reshaping industry practices, while redefining how banks and asset managers operate in an increasingly complex environment. Here, industry experts discuss the multifaceted roles LLMs play in enhancing efficiency, mitigating risks and revolutionising decision‑making processes
The panel
- Alexander Sokol, Founder and executive chairman, CompatibL
- Andrew Chin, Head of investment solutions and sciences, Alliance Bernstein
- Christian Hull, Former head of innovation, Emea, BNY Mellon
How are banks and asset managers leveraging LLMs in their day-to-day operations and decision-making processes?
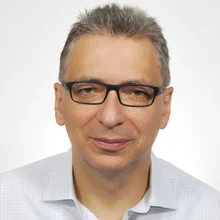
Alexander Sokol, CompatibL: Artificial intelligence (AI) has taken the world by storm, and the banking and asset management industry is no exception. After a very short period of hesitation, most banks, asset managers and hedge funds embraced AI and have been exploring its use in many different applications across their businesses. It was perhaps inevitable that some of these efforts ran too far ahead of the current stage in AI model evolution.
There is no doubt that AI will become increasingly competent over time and will be able to perform more and increasingly complex and critical tasks with less supervision. However, the current state of the art for these models falls short, especially when they are used out of the box without being fine-tuned on financial industry documents. These documents use highly specialised vocabulary and complex concepts that are not well represented in the public datasets used to develop the LLMs for training purposes.
It is very important to recognise that even today’s best LLMs are not capable of generating a complex regulatory filing or an investor prospectus without human supervision. And, without human supervision, today’s LLMs will inevitably produce documents riddled with errors that may have far-reaching negative consequences, including regulatory action or investor lawsuits. This is no surprise – after all, a reputable organisation would never consider delegating the drafting and filing of these documents to an intern or a junior employee without senior supervision.
Successful applications of AI in banking and asset management require not only fine-tuning LLMs on the financial vocabulary, but also using them in a co-pilot capacity, ensuring employees with the appropriate level of skill retain firm control. This is the approach CompatibL took for its first AI product – the AI Co-Pilot.
Andrew Chin, Alliance Bernstein: Asset managers are leveraging LLMs across their organisations – from investments to distribution to back-office functions. They are looking at LLMs to become more effective in synthesising insights faster, while also being more efficient at handling larger volumes of data.
In a data-rich environment, LLMs are valuable – particularly in handling diverse data formats such as text, audio and video. Much of this data can be transcribed to text, presenting enormous opportunities for natural language processing (NLP). As a branch of AI focused on understanding and interpreting textual and spoken data, NLP is the lowest-hanging fruit in this arena. These techniques allow us to ingest very large quantities of incoming data and synthesise it to suggest actions. LLMs, in turn, provide the tools and building blocks for us to leverage NLP. Investment professionals facing the challenge of discerning themes and extracting actionable insights from millions of data points can benefit from NLP-based tools that efficiently analyse data, minimise errors and operate continuously.
Christian Hull: Compared to the much more mature field of machine learning, LLMs are very new, and capital markets firms are naturally cautious. They are all talking about LLMs, but closer examination often reveals that many are still in the proof-of-concept stage. If firms have any LLMs already in production, they are probably limited to internal use cases or carry very little client value or risk. It may sound dismissive, but this cautious approach is rooted in the financial services industry’s mature understanding of risk.
What are the most promising LLM applications and use cases for capital markets firms, and how will this change?
Alexander Sokol: Model governance is uniquely suited for the application of LLMs. It is a critical bank function with multiple stakeholders, including the bank’s auditors and regulators. Its objectives are to exercise control over the model development process and lifecycle, ensure that all model changes are authorised and properly implemented, document the models for the management and regulators, keep complete and up-to-date regulatory and internal model documentation, and publish accurate and comprehensive model release notes.
Model governance requires a tremendous amount of time and effort and can only be performed by a highly qualified team that consists of quants and risk experts. Each regulatory submission or internal model approval requires tens of documents that often reach 300–500 pages. Other than the effort required, the sheer amount of information in these documents increases the risk of inadvertent omissions and errors. Among other business functions in banking, model governance involves dealing with extraordinarily large volumes of natural language text (model documents) and source code (model libraries).
CompatibL recognised the unique potential of LLMs to assist in and partially automate model governance in early 2023. This led to the development of CompatibL’s AI Co-Pilot for model governance.
Before the introduction of this product, model governance solutions provided a limited degree of automation based on rigid templates, and were unable to comprehend free‑form documents or source code, or reconcile multiple sources of information.
What makes CompatibL’s AI Co-Pilot so successful is the unique way it uses LLMs to process and integrate information from model specifications, model test results, model revision history messages, existing documents and regulatory guidelines.
The AI Co-Pilot for model governance performs labour-intensive and time-consuming work that would be unfeasible for even a large human team to accomplish without AI assistance. It can look at every line of source code and every version control log message, perform an in-depth analysis of the prior documentation and release notes, integrating all of this data in a nuanced and sophisticated manner. It can also cross-reference the resulting documents with the specific lines of source code – which is tremendously helpful to the bank examiners and internal risk control function, but is rarely done because of the time and effort involved.
In addition to generating drafts of model governance documents, CompatibL’s AI Co-Pilot can also flag areas of concern inside the source code, including potential bugs, discrepancies between the source code of the model and how the model is described in the documentation, or the use of numerical methods and modelling techniques that were not intended or approved. For example, during a recent routine production use by a client, our software was able to detect a model code change that involved the unapproved use of an approximate numerical method. This was not reflected in the documentation or signed off by risk control, so the discrepancy was flagged for further investigation and remediation. Without the use of the AI Co-Pilot, the discrepancy may have been missed or discovered after the model went into production.
The way AI is used by CompatibL perfectly fits the capabilities of today’s LLMs. Each call to an LLM is limited to a specific, well-defined task over a limited set of data. We are not asking LLMs to write the entire model governance document or propose a model test framework – doing so would go beyond the current LLM capabilities and would not produce acceptable results today.
Only when the LLMs evolve and improve further will we give them a broader set of tasks over larger datasets. We believe the key to successfully evolving AI solutions within the banking industry is to follow evolving LLM capabilities, without getting ahead of them, and to always keep humans in control.

Andrew Chin: In today’s capital markets, LLMs find promise in various applications. First, they excel in interpreting text and efficiently performing tasks such as entity recognition and sentiment analysis. For instance, LLMs can objectively determine the positivity or negativity of a document, offering consistency. In addition, LLMs can be fine-tuned to provide responses that are more consistent with our thought processes.
Another key application is summarisation and topic modelling. LLMs simplify workflows by summarising lengthy documents such as company/regulatory filings or conference calls, providing quick insights into trends.
Chatbots leverage LLMs by enabling users to access information seamlessly, freeing resources in areas such as customer service or compliance queries. Content creation is also a notable use case, where LLMs, once fine-tuned, generate market or client commentaries, serving as valuable starting points for human writers. Finally, in search and question answering, LLMs save time for analysts by efficiently retrieving specific information from extensive documents. An example is searching for environmental, social and governance metrics within a company to track and monitor performance. In all of these cases, fine-tuning and prompt-engineering are important to ensure tasks are performed appropriately.
Christian Hull: Given their focus on processing text data, LLMs show significant potential in fields such as investment analysis and customer service within financial services. In these areas, language serves as a key dataset, unlike operations or payments, for example. Well-trained LLMs will be able to deliver consistent analysis on a large scale, and can offer timely and accurate feedback to clients.
How are banks and asset managers exploring different delivery models for implementing LLMs, and what factors influence these decisions?
Alexander Sokol: The main consideration for banks in choosing a delivery model is the need for data security. The optimal delivery model looks quite different for sensitive data (such as the bank’s portfolio), versus non-sensitive public data (such as quarterly filings).
Most firms will not consider sending their most sensitive data to LLM developers such as OpenAI until these firms can demonstrate a track record of security. While enterprise subscription is now available from OpenAI for its highly capable generative pre-trained transformer (GPT) model family, most banks will prefer to use traditional cloud partners such as Azure or Amazon Web Services, or run LLMs on-premises using their own graphics processing units.
The partnership between Microsoft and OpenAI provides the ability to run GPT LLMs within Azure, without any data being sent to OpenAI servers. This is the preferred deployment option for firms that use GPT models. For banks and asset managers that use the Llama 2 model family, even more options for working with sensitive data are available. Because these models are open source, they can run inside the bank’s own cloud for any cloud vendor, or on-premises.
Many applications of LLMs do not require sensitive data. For example, a bank may use LLMs to analyse regulatory guidelines, quarterly filings and other publicly available information. For non-sensitive data, a cloud deployment model provides the ability to scale the performance – namely, submit many LLM requests at the same time and have them run in parallel. This is something that would otherwise require a massive investment in on-premises hardware, which would remain idle most of the time.
Andrew Chin: Many firms have explored and piloted LLM applications over the past year. As these firms look to implement and scale these applications across their organisations, they may need new ways to deploy these models.
For example, continuously improving LLMs involves capturing real-time feedback from users as they interact with the models. This approach requires users to provide explicit feedback and recommendations during their usage, generating valuable labels and annotations for fine-tuning.
Another influencing factor is the dynamic landscape of LLMs in the marketplace. While OpenAI currently holds an advantage, the rapid evolution – especially among large tech firms – underscores the need for modular pipelines and code. Ensuring upfront flexibility in design enables us to adapt to evolving LLM options in the future.
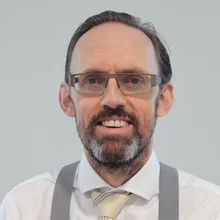
Christian Hull: Many agile firms are building minimum viable products with LLMs to prove their use cases. The degree of application finesse they wrap around the LLM will depend on the end-user, whether internal or external. In many cases, firms aim to seamlessly integrate LLMs into their services without making clients explicitly aware of their use. This may involve obfuscating the role of LLMs in the background. Over time, as the AI hype curve normalises, the acceptance of AI services is expected to increase. However, firms are still proceeding with caution.
What challenges have you encountered in the adoption and implementation of LLMs, and how are you addressing them?
Alexander Sokol: As with any new technology, challenges and growing pains will exist until the LLM technology matures. CompatibL has been able to gain experience with the most powerful commercial LLMs through its early adoption of this technology, to help our clients navigate these challenges.
One of the prerequisites of making LLMs part of enterprise software is the ability to perform quality assurance of LLM-based solutions. Regression-testing is an essential part of software quality assurance, which involves running known use cases through each new version of the software to ensure either the results have not changed, or that they have improved.
The machine learning algorithms inside LLMs make regression-testing challenging due to the variation of the responses that occur from one query to the next, even if the inputs are identical. There are two sources of this variation. One of them is the random seed used by the models to generate responses. Previously, the seed was internally generated within GPT models, which made it very difficult to test enterprise solutions. The recent announcement from OpenAI that it will allow users to provide the seed in the new version of the OpenAI application programming interface goes a long way towards addressing this important issue. For Llama 2 models, users have always been able to pass their own seed, as these models are open source.
Even with user-supplied seeds, LLMs exhibit a natural variation of responses to the same query. This variation is caused by numerical round-off error within the neural network powering the model. The test suite for CompatibL’s AI Co-Pilot uses the advanced test methodologies we developed, which makes it possible to perform reliable testing even in the presence of such variability.
Andrew Chin: Adopting LLMs presents challenges – particularly in addressing ‘hallucinations’ to ensure user trust. Alliance Bernstein employs fine‑tuning, prompt-engineering and retrieval-augmented generation (RAG) to mitigate this issue. Fine-tuning involves gathering real responses from analysts, enhancing model training for specific tasks. Effective prompts and providing context alongside examples significantly improve LLM response quality. RAG restricts LLMs to predefined search areas, reducing the risk of fabricated answers. Model explainability enhances trust by clarifying the origin of answers.
We also believe LLMs should not be used blindly. While decision-making can be improved with LLMs, our analysts and employees must ultimately be accountable for the final decisions. The Marvel character Iron Man can serve as inspiration here – we want to integrate a technologically proficient human expert in a specific field, such as financial analysis, with an AI-powered assistant that can take in a massive amount of input, analyse it and then suggest actions. This setup allows human overrides based on expertise, emphasising human ownership of final decisions, with LLMs providing analysis and context.
Christian Hull: I see the lack of explainability as a significant challenge in the adoption of LLMs. However, this is a human issue – not a technological one. Risk and compliance teams will need to decide where they can relax their risk appetites, and where these must remain steadfast. Demand for explainability in human action lacks a verifiable necessity, suggesting it is unlikely to remain a requirement for LLMs in the long term. The question revolves around how long it will take to make that adjustment to risk perception and policies.
What governance frameworks is your organisation putting in place to ensure the responsible and ethical use of LLMs – particularly in the context of sensitive financial data?
Alexander Sokol: Leaking sensitive financial data – especially personally identifiable information – can lead to serious reputational, regulatory and legal consequences for banks and asset managers using AI. The ability of hackers to get LLMs to disclose the sensitive information fed into them is well known. Until there is a comprehensive solution to this problem, no client-facing LLM solutions or chatbots should have access to sensitive data. Another concern is the ability of bad actors to reprogram the model by carefully crafted prompts, where the model forgets its guardrails and starts producing objectionable, or biased, information.
Most, if not all, of these issues are related to giving banks’ retail clients and the general public the ability to interact with LLMs directly. This is not the mode of operation used by CompatibL’s AI Co-Pilot.
Our AI Co-Pilot software is designed to be used by bank employees in a closely supervised manner, with a full audit log of all interactions with the model. The bank’s retail clients, the general public or any outside users do not have access to the LLMs within CompatibL’s AI Co-Pilot. This deployment approach is an important part of ensuring responsible and ethical use of our software. In addition to having sophisticated guardrails that cannot be breached easily, any attempt to bypass them will be highlighted for immediate investigation with all relevant data available in the audit log.
Andrew Chin: In navigating the risks tied to LLMs – especially those concerning sensitive financial data – Alliance Bernstein is committed to a responsible and ethical approach. Various challenges, such as data security, privacy, hallucinations, biases and automation risks, come into play, impacting the ethical use of LLMs. To ensure responsible use, we have set up several committees. The firm-wide AI Committee oversees our AI usage and associated data. The Model Risk Committee ensures the proper use of models, while the Vendor Oversight Committee manages relationships with external partners. Addressing data privacy concerns falls under the purview of the Data Privacy Committee.
These committees mitigate risks associated with the usage of LLMs and the underlying data. Clear policies and guidelines are also important as a part of the overall governance programme.
Christian Hull: Regulated firms already maintain robust governance measures that should cover model, data and technology risks. I recommend carefully reviewing existing processes with AI in mind, and incorporating additional checks where necessary. The real challenge lies in making governance processes fast and effective. Dealing with complex models can pose challenges in the risk approval process, potentially hindering a firm’s competitive edge. To address this, I suggest looking to tech-native firms as examples and learning how they manage risk with agility and efficiency.
To what extent will regulatory restrictions limit the adoption and deployment of LLMs in the financial sector?
Alexander Sokol: Classic quant models generate prices and risk – something humans cannot calculate or validate directly. This is why model validation for classic quant models, on the one hand, involves validating the code that produces these numbers and analysing test results on sample data.
On the other, LLMs consume and generate natural language documents. Here the situation is the exact opposite: humans are not able to validate the complex machine learning code inside LLMs that produces these results, but are able to validate the model output – the natural language documents and data it produces, for example. The ability of humans to perform validation and sign-off on LLM outputs is what makes the use of this technology acceptable to regulators.
Consensus regarding the use of LLM-based tools such as CompatibL’s AI Co-Pilot is that LLM-generated and human-validated documents should be treated the same as documents drafted by a human from start to finish, as long the human has ultimate responsibility for signing off on the result.
In CompatibL’s AI Co-Pilot, the requirement that a human analyst must validate each LLM output is built into the system. This feature of AI Co-Pilot’s design guarantees that no output can be provided to downstream systems without an audit log record of human validation and sign-off.
Andrew Chin: Regulatory restrictions and guidance will always inform how we operate and the types of tools we use. As more regulators worldwide release frameworks and expectations in the coming years, we may need to adjust how we are using LLMs.
Christian Hull: Regulators are showing an encouraging amount of global cohesion in addressing AI concerns. Initiatives such as the European Union’s AI Act, or US president Joe Biden’s Executive Order on the Safe, Secure, and Trustworthy Development and Use of AI, underscore a shared focus on fundamental risks. Most firms tend to align with regulatory objectives. While complying with AI regulations introduces additional reporting burdens, it isn’t a material obstacle for large financial service firms. However, the costs may pose limitations – particularly for smaller firms or specific business cases.
The panellists’ responses to our questionnaire were made in a personal capacity, and the views expressed herein do not necessarily reflect or represent the views of their employing institutions.
Sponsored content
Copyright Infopro Digital Limited. All rights reserved.
You may share this content using our article tools. Printing this content is for the sole use of the Authorised User (named subscriber), as outlined in our terms and conditions - https://www.infopro-insight.com/terms-conditions/insight-subscriptions/
If you would like to purchase additional rights please email info@risk.net
Copyright Infopro Digital Limited. All rights reserved.
You may share this content using our article tools. Copying this content is for the sole use of the Authorised User (named subscriber), as outlined in our terms and conditions - https://www.infopro-insight.com/terms-conditions/insight-subscriptions/
If you would like to purchase additional rights please email info@risk.net