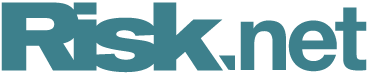
This article was paid for by a contributing third party.More Information.
Revolutionising credit surveillance: part one
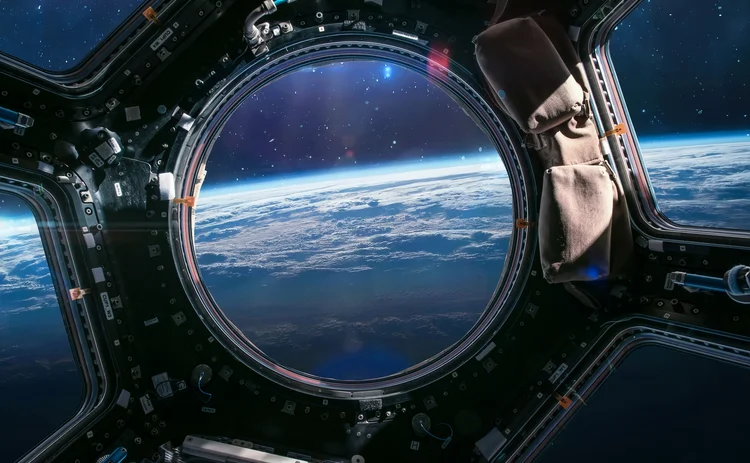
Early warning indicators for credit risk changes are key, especially during high market volatility. Some of these indicators rely on security prices as the primary driver; however, the volatility of market prices may cause the early warnings to be active and inactive in consecutive months. Promptly detecting changes in credit risk profiles, even when defaults do not take place, is essential and can support fixed income investing, market risk monitoring and Basel IV due diligence
The authors
- Michelle Cheong, Head, Credit Solutions Thought Leadership, S&P Global Market Intelligence
- Clemens Thym, Managing director and global head, Credit Solutions, S&P Global Market Intelligence
- Shruthi Nagarajan, Senior research analyst, Credit Solutions, S&P Global Market Intelligence
The authors acknowledge research partners Paige Tan, director, Pricing Valuations and Reference Data, and Min Jiang, director, Analytical, Innovation and Development Group, Credit & Risk Solutions, for the creation of this material.
Market problems and use cases

Recent cases of credit distress reveal the importance of promptly detecting changes in credit risk profiles even if defaults do not take place. This can support fixed income investing, market risk monitoring and Basel IV due diligence, among others.
No one-size-fits-all early warning signals
Early warning signals for credit risk changes have been available for some time. This article examines several flavours of these signals:
- Purely based on indicators from market prices
- Using credit risk fundamentals
- Derived from natural language processing (NLP) on digitised research and news.
The double-edged sword of early warning signals based on market prices

Many early warning indicators of credit risk changes rely on security prices as the primary driver. Credit default swaps mapped to the same scale as credit ratings have often signalled ratings changes. However, the volatility of market prices may cause the early warnings to be active and inactive in consecutive months.
Fundamentals as early warning indicators
Early warning indicators on credit risk can also be rooted in fundamental analysis. Two examples are the business risk profile and the financial risk profile, which build up to corporate credit ratings.

The business risk profile is the risk and return potential for a company, its industry and country risks, and the company’s competitive position. The financial risk profile is the outcome of decisions that management makes in the context of its business risk profile and its financial risk tolerances. S&P Global Ratings maps these to a numerical score, from 1 (best) to 6 (worst), which enables their use for benchmarking. Entities with the same ratings category can have very different business and financial risk profiles. Figure 1 provides an example of the distribution for BBB+ and BBB- rated entities.

Note: For BBB+ to BBB- rated entities, the average business risk profile was 3 (satisfactory) and financial risk profile 3 (intermediate). The yellow-shaded area is where a worse-than-average financial risk (business risk) profile was equally offset by a better business risk (financial risk) profile. We classify entities in the upper-left diagonal as those with above-average business plus financial risk profile, shaded green; and in the lower right diagonal as those with below-average business plus financial risk profile, shaded red. These classifications are proxies computed by Credit Solutions Thought Leadership from S&P Global Market Intelligence, which is independent of S&P Global Ratings.
In our sample of 6,842 rated corporates, from 2014 to 2024, entities with better business plus financial risk profiles experienced net ratings upgrades over 24 months, while those worse than their peers had net ratings downgrades over the same period (figure 2).

Some observations from past cases are presented in figure 3 (better/worse relative to similarly rated peers).
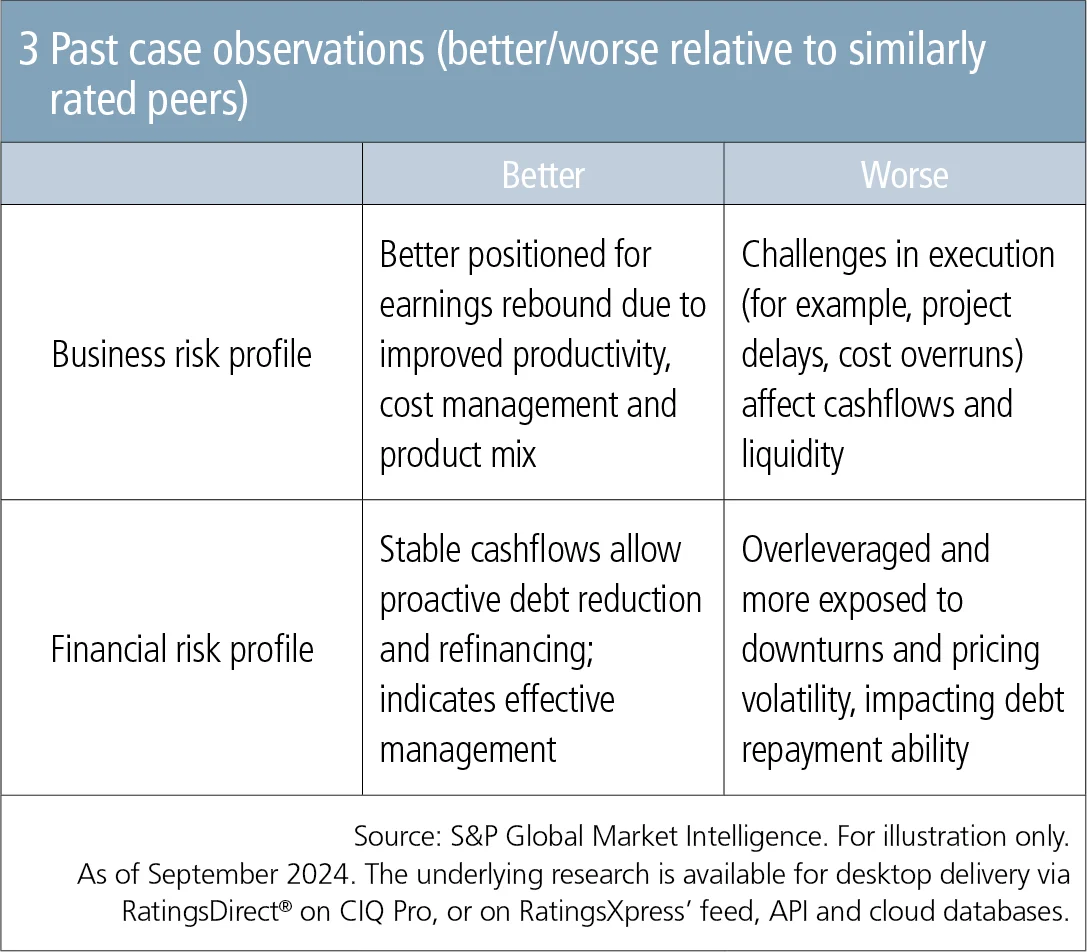
Furthermore, companies that relied heavily on parent/sovereign support to attain credit ratings levels above their own fundamentals could see credit ratings downgrades if the parent’s/sovereign’s credit quality deteriorated.
In an event study using yield curve spreads over the zero-coupon treasury curve (Z-spreads), we found that bonds had not fully priced in the information from business and financial risk profiles. The Z-spreads of entities with better‑than-average business plus financial risk profiles narrowed over time, while those with worse-than-average profiles widened (figure 4).

Potential added edge from NLP on credit ratings research
Text sentiment, using NLP techniques on digitised text of research reports, can provide an additional gauge of credit fundamental trends. To illustrate, we ran a simple ‘bag of words’ analysis on specific sections of entity research articles using an open-source dictionary.
When the text sentiment of S&P Global Ratings’ Full Analysis and Research Update reports was significantly below (or above)1 that of peers with the same credit rating, there were more credit ratings downgrades (or upgrades) over the subsequent 24 months (figure 5). Empirical tests show this effect is incremental to CreditWatch and Ratings Outlook.

This material has been created by S&P Global Market Intelligence. S&P Global Market Intelligence’s opinions, quotes and credit-related and other analyses are statements of opinion by S&P Global Market Intelligence as of the date they are expressed and not statements of fact or recommendations to purchase, hold or sell any securities or to make any investment decisions, and do not address the suitability of any security. This material does not represent the views of S&P Global Ratings, nor did S&P Global Ratings participate in the creation of this material.
Disclosures for this article from S&P Global Market Intelligence
1Research from S&P Global Market Intelligence Thought Leadership defined significantly below- (or above-) average text sentiment as those where sentiment scores were less (or more) than one standard deviation below (or above) the average of the peer group sharing the same credit rating. See Michelle Cheong and Shruthi Nagarajan, The informativeness of research reports – is all text content created equal?, S&P Global blog, January 2024.
Read more
Revolutionising credit surveillance: part two
Does GenAI live up to the hype?
Sponsored content
Copyright Infopro Digital Limited. All rights reserved.
As outlined in our terms and conditions, https://www.infopro-digital.com/terms-and-conditions/subscriptions/ (point 2.4), printing is limited to a single copy.
If you would like to purchase additional rights please email info@risk.net
Copyright Infopro Digital Limited. All rights reserved.
You may share this content using our article tools. As outlined in our terms and conditions, https://www.infopro-digital.com/terms-and-conditions/subscriptions/ (clause 2.4), an Authorised User may only make one copy of the materials for their own personal use. You must also comply with the restrictions in clause 2.5.
If you would like to purchase additional rights please email info@risk.net