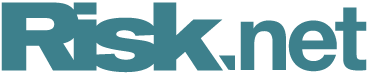
This article was paid for by a contributing third party.More Information.
How small and medium-sized banks can enhance deposits modelling frameworks
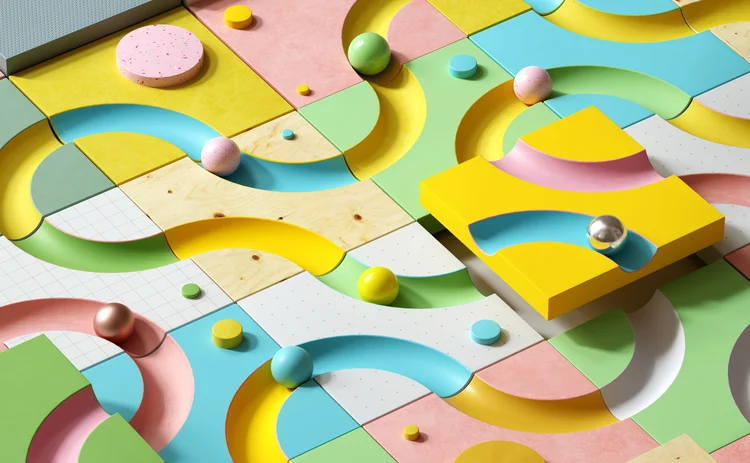
Recent events have called into question the reliability of deposits as a primary source of funding for small and medium-sized banks. Stickiness of deposits that generations of bankers had counted on suddenly seem ephemeral
Typical deposits models at smaller banks use a simple top-down approach with few segments. This traditional deposits modelling framework has proven inadequate to accurately predict the level and the pace of the deposit outflows observed at certain banks. The rapid rise in interest rates, as expected, unleashed systemic deposit outflows. The expeditious outflows at certain banks that ultimately led to their demise, however, was unexpected. Unlike the bank-run of the past, the affected banks did not have any significant credit issues. Many factors – including the rise in money transfer technology, changing depositor preferences, the persistent near-zero interest rate environment of the last decades, excess liquidity from Covid-19 programmes, and historically high inflation rate – contributed to this outcome.
In light of these events, banking regulators are increasingly shining a spotlight on deposits and will likely subject deposits models to higher levels of scrutiny. In anticipation of this heightened scrutiny, this article presents a simple road map of how banks can enhance their deposits modelling frameworks. A bottom-up approach is proposed, using several sources of relevant data for analysis. Specifically, six types of data that can be integrated into modelling frameworks are discussed.
A typical deposits modelling framework
Broadly, typical top-down bank deposits modelling frameworks consist of five primary tasks, a high-level overview of which is presented in figure 1.
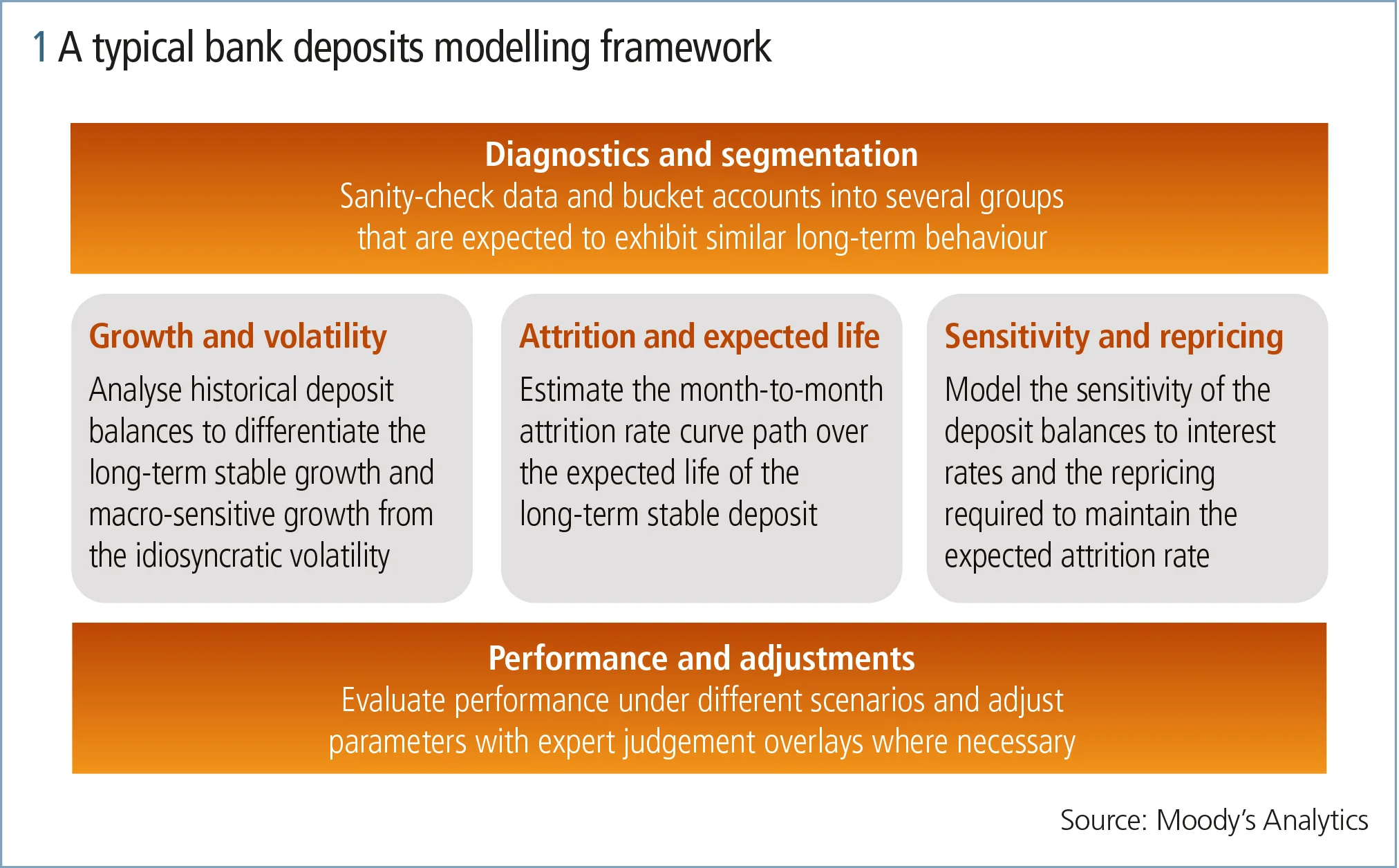
Deposits models currently implemented at many typical small and medium-sized banks leave much to be desired in terms of quantitative rigour, their qualitative ability to provide insights about depositor base trends, and their ability to inform on key gaps and risks. The focus of these modelling exercises is primarily to estimate the required parameters to run the asset-liability management (ALM) analysis.
Figure 2 presents a stylised vision for a second-generation deposits modelling framework, aimed at addressing the shortcomings of the typical top-down modelling approach.
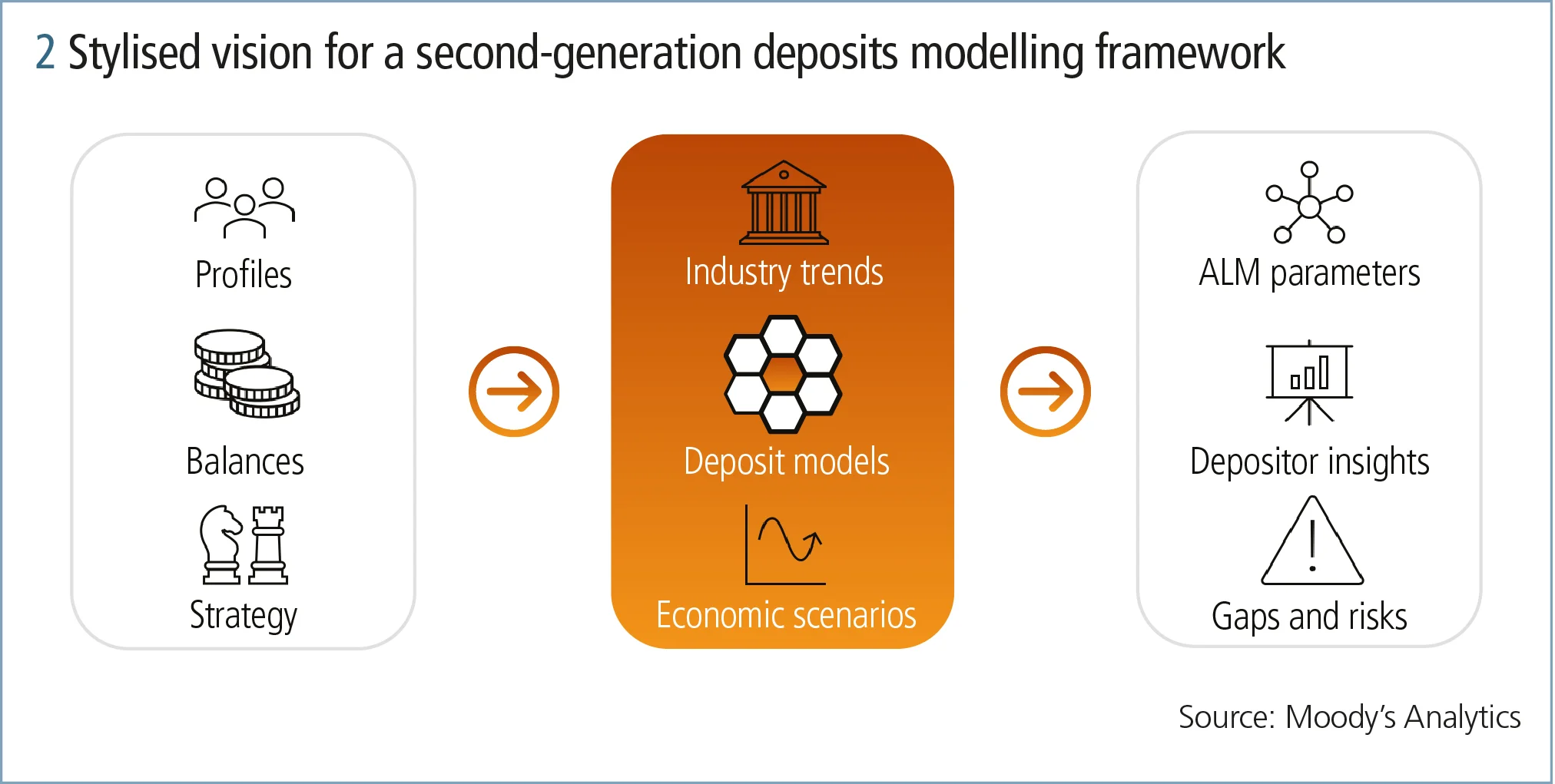
Second-generation deposits modelling frameworks
It is recommended banks approach the enhancement of deposits modelling with two primary objectives: bringing analytical rigour to deposits analysis and enhancing the role of deposits analysis as a strategic tool.
For the first goal, banks are encouraged to consider several enhancements, including switching to a bottom-up approach. This framework is set up to analyse behaviour at the account or relationship level rather than segmenting at the product type or balance cutoffs.
The most critical aspect of this approach is to assess potential sources of relevant data that can be considered for model development. Six types of data are highlighted for this purpose: demographic attributes, behavioural patterns, banking relationships, segment concentration, competitive landscape and macroeconomic forecasts. This list is not exhaustive, and is merely a starting point selected based on Moody’s Analytics experience of what would be achievable given the current state of data management at typical small and medium-sized banking institutions.
Furthermore, this modelling approach seeks to limit reliance on unstructured subjective judgements and one-off manual adjustments as a way of compensating for the factors that are clearly important but not considered in the modelling framework.
Finally, this approach avoids manually changing ALM model parameters across scenarios. This is achieved by integrating economic scenarios within the modelling framework such that key parameters are estimated as a function of relevant economic variables. This enables model parameters to quickly adapt to the changing industry conditions.
Regarding the second goal, deposits modelling is often relegated to a check-box regulatory compliance exercise. However, assumptions about deposits are foundational to risk management, pricing, business growth and other strategic decisions. Different functions within banks have their own siloed views about deposits that may rely on different sources of information and may be internally inconsistent.
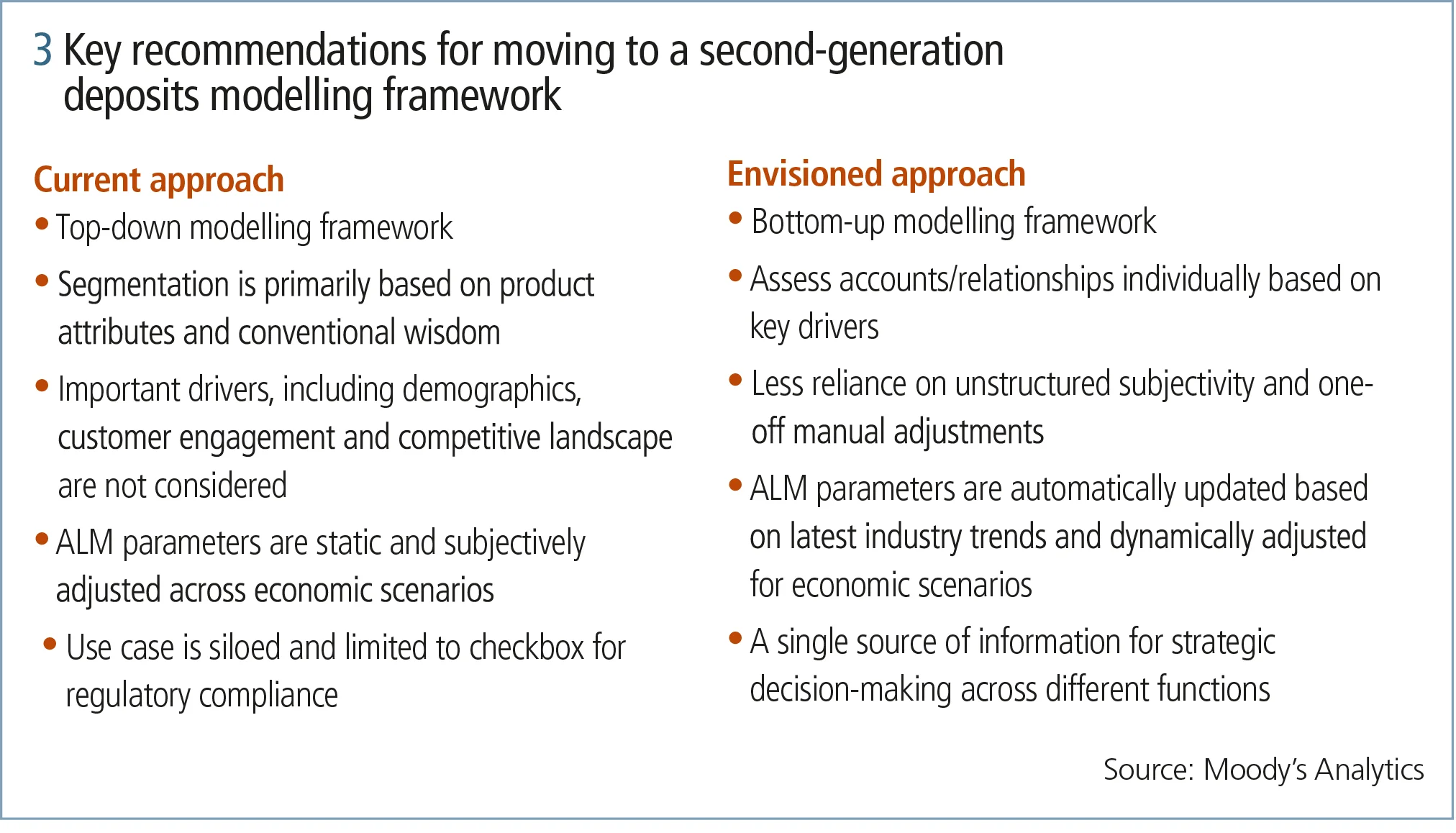
A summary of the recommendations for moving from current practices to an enhanced approach is presented in figure 3.
Integrating siloed data
A key recommendation is the integration of data that is currently siloed into a unified modelling framework that can serve multiple needs of different bank functions. The six different data types for consideration are highlighted in figure 4.
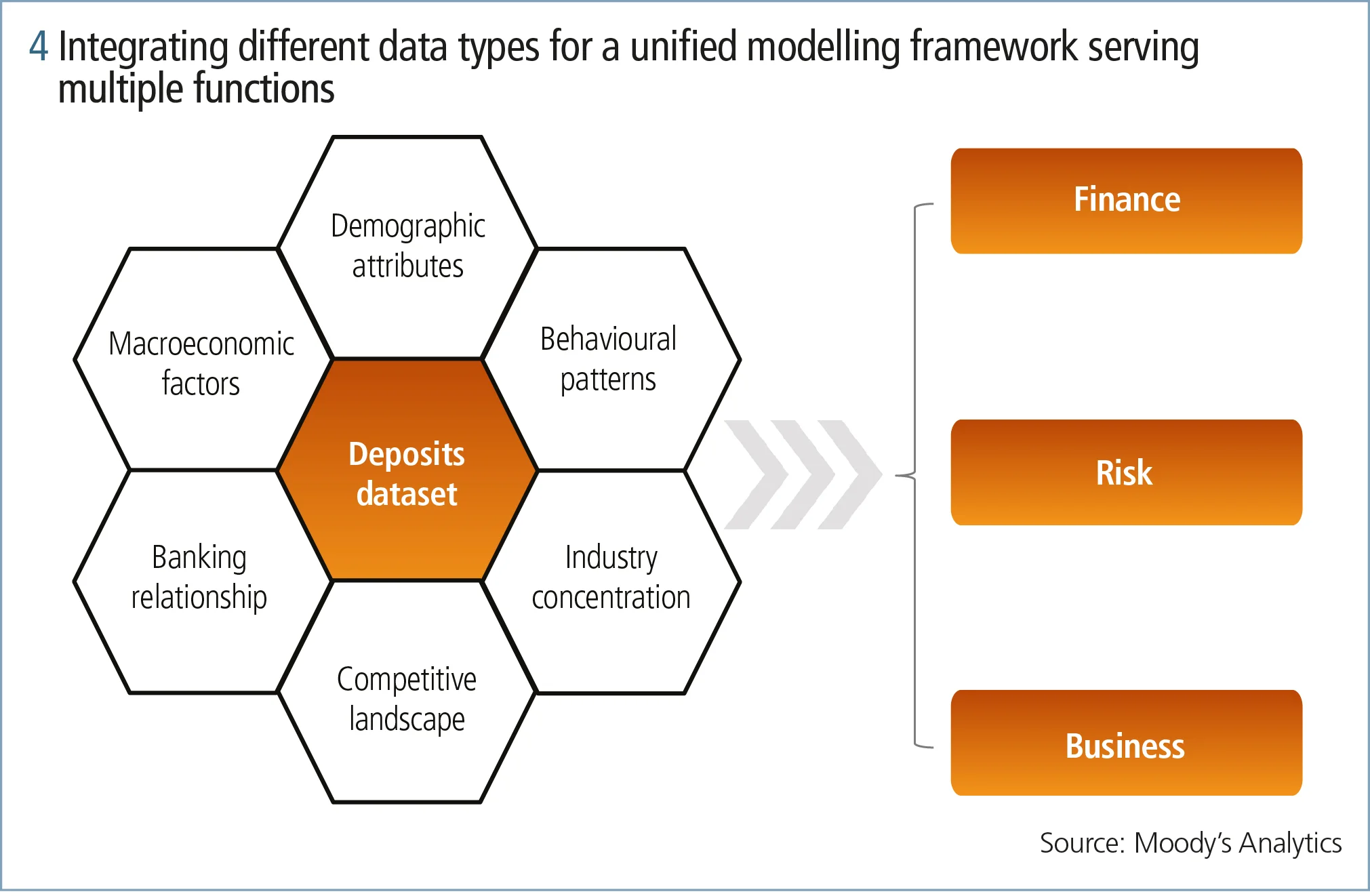
1. Demographic attributes
Basic information that helps create depositor profiles to categorise traits and develop indicators for key life or corporate events that potentially change depositors’ needs and use of cash. This category includes biographical or business profile information collected as a part of the know-your-customer or other information-gathering processes. Additionally, tracking of events – corporate or personal – may prove to be an early signal for changes in cash use patterns.
2. Behavioural patterns
Banking services usage patterns and engagement microdata provide valuable insights into not only how best to grow stable deposits, but also for other business developments and operational efficiency. Mode and frequency of cross-service offerings – including uses of online or mobile banking, frequency and location of ATMs, branch offices and customer services – provide valuable clues regarding how well the bank meets customer needs and likely customer retention.
3. Customer relationships
A deep understanding of the customer relationship and value proposition helps identify long-term beneficial relationships worth pursuing from those that are merely transactional. Channel of customer acquisition, relationship length, referrals, number of products involved, revenue potential and overall value proposition are some of the examples of factors to consider to achieve segmentation of depositors into strategic, tactical and expendable.
4. Industry concentration
Having the depositor base categorised by employer and/or business segment helps identify concentrations that may turn into unexpected vulnerability if the employer/business segment experiences distress. Similarly, a structured way of tracking major litigation/scandal, legislative changes and corporate or industry events may serve as an early indicator of future changes in deposit flows.
5. Competitive landscape
Keeping an eye on the products and services offered by competitors and potential disruptors enables one to put in place a defensive market strategy and focus on customers who could be poached away. Enumerate the services offered by competitors and the conveniences of those to each customer, such as number of ATMs and branch offices near the customer’s home or office.
6. Macroeconomic factors
The impact of macroeconomic or systemic factors on the deposits cannot be avoided but can be better managed with proper understanding and preparation. Stress-testing and what-if analysis on thoughtfully prepared scenarios provide actionable insights.
Achieving this vision for second-generation deposits modelling requires a comprehensive data plan to bring together data elements that are currently siloed and are not generally considered within the context of deposits analysis.
Account-level data is often considered a by-product of doing business and is ignored once its intended one-off purpose is completed. A detailed data plan is needed to bring back to life these ‘filed and forgotten’ data elements for modelling purposes. For a successful implementation, the data plan should include strategies to resolve the common data challenges, including the following:
1. Anonymisation
The envisioned data plan calls for the use of detailed depositor profile information, hence robust tools and techniques must be in place to mask sensitive information such as personally identifiable information to comply with consumer protection regulation.
2. Standardisation
Successfully bringing together data from various parts of the organisation requires common definitions and formats to be used across sources.
3. Preprocessing
Numerous data elements envisioned – such as cash deposits and withdrawals – require aggregation or preprocessing of the raw records to be integrated into the deposits database.
4. Data gaps
Certain kinds of data elements, such as products and services offered by competitors, may not be currently available, and certain data elements may not have full coverage across geographies or historical time periods.
Conclusion
Recent events have put a spotlight on the traditional framework in which deposits are analysed using embedded assumptions borne out of historical experience and convention. Banks are inevitably going to face heightened scrutiny and will likely be expected to enhance their modelling methodologies.
This article has presented ways small and medium-sized banks can embark on the path towards improvement by focusing on integrating data elements into the modelling framework that they already collect or that are easy to collect, but are currently not within the purview of deposits modelling. Beyond technical improvements, enhancing the role of deposits modelling as a strategic tool that serves multiple functions within the bank is also recommended.
About the author
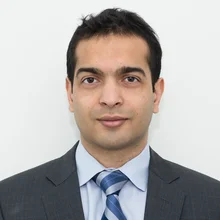
Prem Neupane heads the Custom Solutions for Americas Banking Advisory Services team at Moody’s Analytics. He has served as senior adviser in a variety of modelling engagements for commercial banks and other large financial institutions. Select engagements include developing and/or reviewing probability of default, loss given default and correlation models for commercial and industrial and commercial real estate portfolios for impairment or stress-testing purposes. He has served as external adviser for model risk management teams reviewing internal risk ratings, prepayment, deposit, ALM and liquidity models, and has helped clients address regulatory feedback in those areas.
Sponsored content
Copyright Infopro Digital Limited. All rights reserved.
As outlined in our terms and conditions, https://www.infopro-digital.com/terms-and-conditions/subscriptions/ (point 2.4), printing is limited to a single copy.
If you would like to purchase additional rights please email info@risk.net
Copyright Infopro Digital Limited. All rights reserved.
You may share this content using our article tools. As outlined in our terms and conditions, https://www.infopro-digital.com/terms-and-conditions/subscriptions/ (clause 2.4), an Authorised User may only make one copy of the materials for their own personal use. You must also comply with the restrictions in clause 2.5.
If you would like to purchase additional rights please email info@risk.net