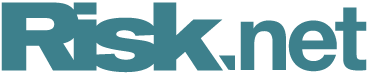
This article was paid for by a contributing third party.More Information.
Only human: the secret of reliable LLM workflows
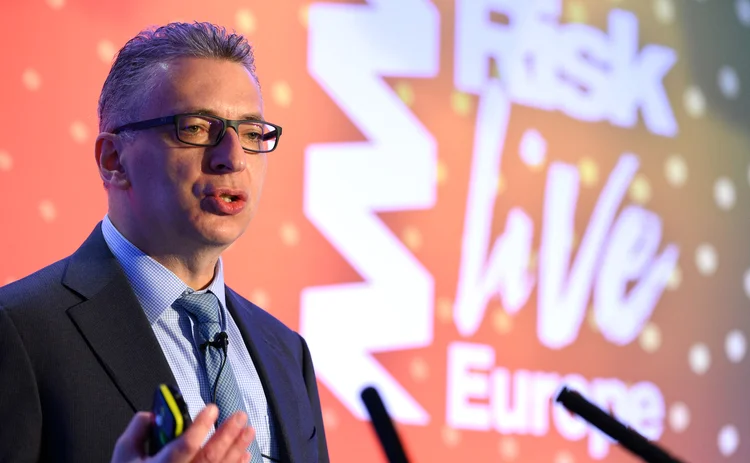
Financial firms are experimenting with artificial intelligence (AI) and large language models (LLMs) in their quest to drive efficiencies, improve decision-making and manage risk more effectively. This article explores research that informed a Risk Live Europe session, which was run by Alexander Sokol, executive chairman and head of quant research at CompatibL. It examines the strengths and limitations of LLMs and offers insights on how to effectively integrate them into business workflows
Capital markets potential

Sokol outlined the huge potential for LLMs within the capital markets sector: “The industry is fairly unique in that there are vast amounts of both data and unstructured natural language text that need to be analysed and understood. This makes capital markets a perfect sector for LLMs, given their ability to process natural language and act as a bridge between text and data.”
Audience polls conducted during the session revealed that the industry largely shares this positivity: 86% of the audience are already seeing the tangible benefits of using AI within their firms, or expect to within the next two years, while more than three-quarters would trust AI for specific business purposes such as generating code, documents or data.
However, Sokol did not shy away from some of the problems encountered, such as ‘hallucinations’ and seemingly simple mistakes, which have impacted confidence in LLM abilities. “People have experienced LLMs failing with tasks such as data extraction and have struggled to solve these issues, which perhaps explains the relatively low proportion of the audience [28%] that would trust AI to generate data,” he noted.
Understanding the human-like traits of LLMs
To tackle these issues, Sokol outlined the findings of CompatibL’s research, which has been used to design and build its suite of AI-based products and can be applied to a wide range of LLM use cases. “When building LLM-based workflows, the critical concept to understand is that they have more in common with the human brain than with traditional computer programs. When LLMs fail, they fail like humans,” explained Sokol.
Just like humans, LLMs have a remarkable ability to process unstructured text, sound and images. They are resilient to changes in input formats and can use logic to follow imprecise instructions.
However, LLMs have also inherited some of the limitations of human cognition. Sokol underlined that, while some of these weaknesses will diminish as LLMs develop, they will never completely disappear. Understanding how to overcome these challenges is therefore crucial for developing effective and accurate LLM-based workflows.
Tackling LLM limitations
Limited recall
One key example is the inability of LLMs to accurately memorise and recall long texts. “The process of converting text to meaning means that the precise retention of inputs is not guaranteed and becomes less likely as the amount of text increases,” explained Sokol. “Like humans, LLMs are capable of remembering the general meaning of an entire 300-page book, but would have difficulties reciting the exact words of half a page.”
Limited recall means that extracting data from large documents and inserting it into outputs can carry a significant risk of errors.
To use LLMs successfully in business applications, Sokol advised against setting tasks that humans cannot perform. “For example, rather than requesting them to memorise extensive term sheets and enter the details of trades ‘from memory’, LLMs perform better when allowed to refer back to documents during a task,” he said.
Sokol also suggested strategies such as markup – asking LLMs to identify the location of data elements in a document and then using conventional code for extraction – and checklists, keeping track of captured data fields to ensure all necessary data is included.
Response variability
Another inherent characteristic LLMs share with human decision-making is variability of response. “An employee would probably not draft exactly the same document at 10am as they would at 10:01am, and two equally qualified employees would certainly not produce exactly the same version word for word,” noted Sokol.
Similar variability in LLM responses can pose challenges in business workflows that require consistency. “To manage these challenges, we need to take the same measures we would when dealing with variability and errors by humans,” he argued. “This involves assigning tasks that are within LLM capabilities, recognising their fundamental limitations, and ensuring they work under continuous human supervision or validation workflows.
Cognitive bias
Other limitations affecting LLMs and humans include cognitive biases, such as distinction bias, and the potential negative impacts of an ‘eagerness to please’. “It is vital to learn how to avoid harmful cognitive biases,” said Sokol. “For instance, in relevance-ranking tasks, asking an LLM to assign an absolute score to a document in isolation will lead to unreliable and variable results. However, asking the model to compare and rank documents relative to each other can yield far more accurate and consistent outcomes.”
“To tackle the hallucinations that can emerge if LLMs feel that users want a particular answer, the key is to find a way to get the information without letting on what you are looking for,” he continued.
Increasing confidence in LLMs
Sokol believes that industry confidence in the ability of LLMs to tackle tasks such as data extraction will rapidly increase as more companies like CompatibL start publicising their solutions and awareness of LLM capabilities grows.
“CompatibL has focused on the areas where it sees the most successful use cases, namely data extraction (converting natural language unstructured text to rigorously formatted data) and validation,” explained Sokol. “Data extraction is where the most rapid progress can be made and where LLMs can work in a highly reliable manner. There are rigorous criteria for success or failure and clear goalposts for accuracy. In addition, it is an area in which users really appreciate LLM involvement. The majority of data extraction and validation cases are those that users find boring, repetitive and unrewarding.”
As an example, Sokol highlighted CompatibL’s Security Prospectus Analyzer solution, which looks at whether a prospectus is compliant with certain regulations or meets certain business criteria. “We believe that’s an amazing opportunity for LLMs, where a tremendous amount of very repetitive and boring work goes on,” he said.
Summary
“The key to building reliable and successful LLM-based workflows lies in understanding their limitations and recognising where they might fail,” Sokol concluded. “Like humans, LLMs produce variable results and sometimes make mistakes. This does not stop us from relying on humans, and it should not stop us relying on LLMs. Instead, we need to set LLMs up in ways that would help humans succeed at the same task. This crucial insight should form the fundamental building block for effective LLM use in the capital markets sector.”
Sponsored content
Copyright Infopro Digital Limited. All rights reserved.
As outlined in our terms and conditions, https://www.infopro-digital.com/terms-and-conditions/subscriptions/ (point 2.4), printing is limited to a single copy.
If you would like to purchase additional rights please email info@risk.net
Copyright Infopro Digital Limited. All rights reserved.
You may share this content using our article tools. As outlined in our terms and conditions, https://www.infopro-digital.com/terms-and-conditions/subscriptions/ (clause 2.4), an Authorised User may only make one copy of the materials for their own personal use. You must also comply with the restrictions in clause 2.5.
If you would like to purchase additional rights please email info@risk.net