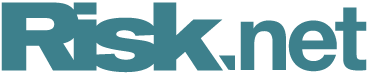
Sponsored statement: Capitects

A key element of finding the optimal portfolio is the assessment of the probability distribution of portfolio returns at individual time steps using multi-period, multivariate Monte Carlo simulations. In modern financial markets – especially in the wake of the financial crisis – it becomes crucial to model the time evolution of complex structured products individually before putting them in the context of broader asset allocations. In this way, financial advisers can choose the appropriate level of transparency, enhancing the intuitive element of the investment decision-making – as it is strongly advocated by both the final investors and the international regulators – and revealing the added-value contribution of the wealth management industry.
Motivation
Building an optimal portfolio allocation is one of the main drivers of the added-value proposition in financial advisory. According to the 1950s Markowitz proposition, this relates to finding the portfolio allocation that promises the highest return at a given risk level, or vice versa. While this approach has been broadly used for decades, one can now ask whether it is still appropriate:
1. Modern investment opportunities contain a large proportion of structured products, the asymmetric distribution of returns of which is not captured by the first two moments.
2. Investors’ characteristics and preferences are brought to centre stage of the optimisation process, bringing modern asset and liability principles into the context of private banking advisory.
Modern investors define targets and constraints not only at a single point in time but as a set of constraints at different time horizons. Therefore, optimisation algorithms will be able to evaluate constraints and optimisation criteria on the whole grid of future time steps by imposing a free adjustable-weighting schema. For example, an investor may be willing to take more risk in the short to medium term to profit from existing market opportunities, while maintaining capital protection in the long term.
The added value of wealth management propositions is tremendously increased by including individual investor guidelines and by discussing fees, transaction costs and taxes in a transparent manner. Only the adoption of simulation over time frameworks enables the definition of an optimal portfolio that combines all of these elements. This effort contributes to enhancing market efficiency by bringing about more transparent and rational decision-making.
Course of action
The following describes the process of an investor searching for the optimal asset allocation in this sense. First, the investor – or the financial adviser on his behalf – defines the products or asset classes in which he might be willing to invest.
Second, the investor – with the aid of the financial adviser – indicates his level of risk acceptance and anticipates his requirements in terms of future in and out cashflows, to be incorporated into the optimisation. The investor’s constraints can easily be transformed into time-varying statements imposed on the distribution of product and portfolio total returns.
The analysis of the probability distribution over time allows the portfolio risk and return potential to be consistently gauged within the investor’s constraints.
Case study
The following investment opportunities are available to a European investor:
- Money market fund, invested in European bonds with six months’ average duration.
- German government bonds with five- or 10-year maturity.
- Fixed income fund, invested in Spanish corporate bonds with five years’ average duration.
- Multi-market fund, invested in European markets and interest rates.
- Equity funds, invested in EUR- or USD-denominated stocks.
- Equity funds, invested in EUR- or USD-denominated stocks, wrapped in products bearing a two-year capital guarantee.
- EUR or USD cash.
The approach presented here allows for the direct modelling of the risk/return characteristics of real products, without reverting to simplified asset classes. This is a fundamental element, enabling a unique view on the strategic asset allocation as a driver of the operational asset allocation.
A Monte Carlo multivariate simulation is performed for a series of time steps into the future. An example is the modelling of the investment into a fund granting a two-year capital guarantee: after the first two-year period, the payoff is reinvested into a similar instrument featuring again a two-year guarantee. Figure 1 illustrates the impact of the capital guarantee: every two years the probability of losing money vanishes. Only if the fund is evaluated between two-yearly reset points, corresponding to a disinvestment prior to expiry of the guarantee, the investor can suffer a loss.
In the present case, at each step, 1,000 stochastic scenarios are generated, bringing the total of revaluation points to 22,000 up to the final time step at six years. In each scenario, a full revaluation of each product is performed and combined with the impact of in and out cashflows, performance fees, upfronts and, potentially, taxes. The set of all scenario returns at a common time step defines the probability distribution of total returns at this point in time.
Here, individual assets can contribute to the initial asset allocation at a multiple of 10% of the total portfolio value. Furthermore, a concentration limit is imposed to prevent any asset contributing more than 30% of the initial portfolio value. From the set of the permitted circa 45,000 allocations, the optimisation algorithm will carve out the allocation that presents a maximum in terms of probability of reaching the target, while complying with all of the risk limits and time-horizon constraints set by the investor. Here, the investor requires his allocation to be verified and optimised at every yearly time step within the six-year time horizon.
The investor’s risk profile in figure 2 (lambda profile) defines the level below which the 5% quantile of a potential portfolio is not allowed to fall. Here the investor is willing to take some risk in the short term, but wants to limit the downside more strongly in the medium and long terms. One can easily choose among alternative measures such as shortfall probability.
The investor also requires a target return that increases with time (zeta profile). The target return quantifies the desired minimum profits the investor is trying to achieve and the optimisation algorithm aims to maximise the probability to reach that level.
The step function in figure 2 visualises the risk/return profile of the investor, the six time points (bars) considered by the optimisation algorithm and the evolution of the 5% and 95% quantile of the identified optimal portfolio allocation. One can easily verify that the risk limit is fulfilled at every time step. For comparison, the base scenario is also shown: this is the expected total return of the portfolio growing according to the forward structure of the risk-free rate.
The portfolio asset allocation is required to be evaluated at several points in time. Here, the criterion for the maximisation is defined as the simple average of the probabilities of reaching the target at each individual time step. One can certainly apply a different weighting scheme to yield more relevance to the short against the long term.
As a result of this case study, the investor’s risk/return ambitions are best served if 30% of the available investment sum is allocated to the 10-year German bond, 30% to the money market fund EUR-denominated, 30% to the Spanish corporate fund with five years’ average duration and 10% to the equity fund wrapper on EUR-denominated stocks with a two-year capital guarantee.
The overall average probability to reach the target profile over time is about 37%. The same exercise, with two observations at two years and six years, would define an optimal portfolio with probability of reaching the target circa 70%. Blending the investor’s ambitions and the time horizons, the set of investment opportunities allow defining the optimal asset allocation in a transparent and fairly customised way.
Click here to view the article in PDF format
Sponsored content
Copyright Infopro Digital Limited. All rights reserved.
As outlined in our terms and conditions, https://www.infopro-digital.com/terms-and-conditions/subscriptions/ (point 2.4), printing is limited to a single copy.
If you would like to purchase additional rights please email info@risk.net
Copyright Infopro Digital Limited. All rights reserved.
You may share this content using our article tools. As outlined in our terms and conditions, https://www.infopro-digital.com/terms-and-conditions/subscriptions/ (clause 2.4), an Authorised User may only make one copy of the materials for their own personal use. You must also comply with the restrictions in clause 2.5.
If you would like to purchase additional rights please email info@risk.net