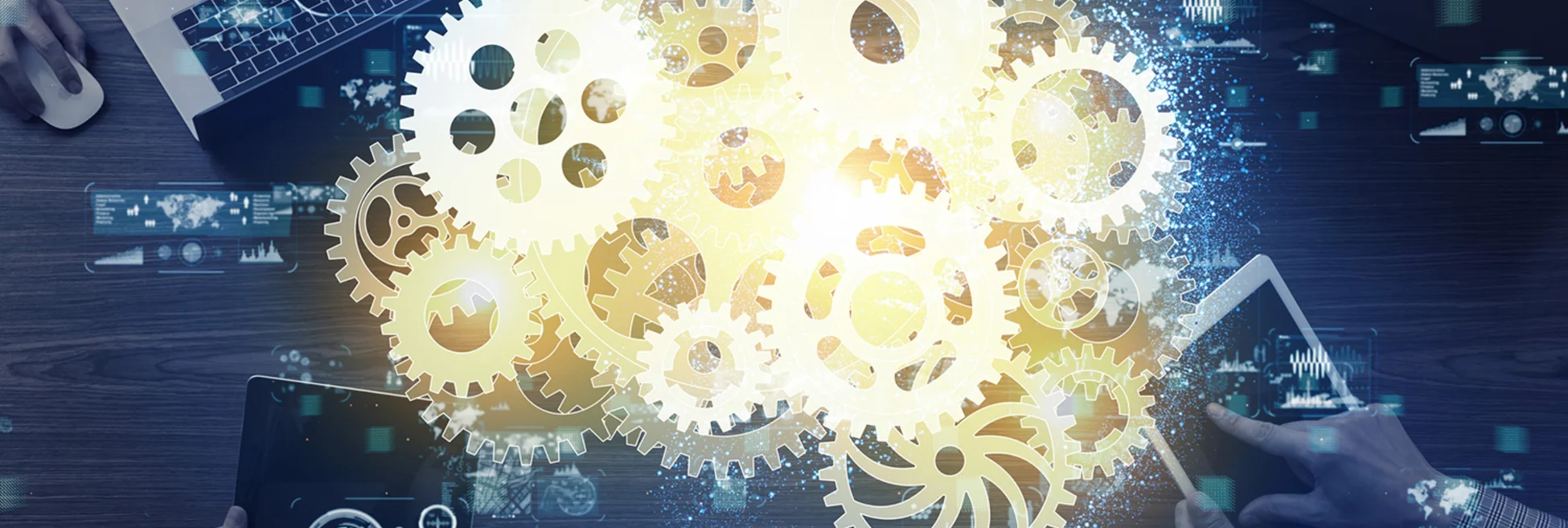
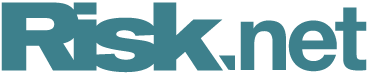
Banks use machine learning to ‘augment’ corporate sales
Big banks are embarking on massive projects to tie up machine learning and big data to sell better to clients
Need to know
- Large dealers have started ambitious projects to apply machine learning techniques to previously unexplored data sources to improve how they sell to corporate clients.
- Both external market data as well as internal data on clients will be used to develop risk advisory robots that offer advanced insights into client needs.
- The techniques being explored will help banks predict client behaviour, identify market opportunities, extract information from news and websites, and alert sales based on market triggers.
- Some banks are also exploring the use of natural language processing to extract information from emails and speeches in the future.
Under pressure to cut costs, banks are all facing the same dilemma: how to do more with less. Many have embarked on transformation projects, leveraging big data to identify potential client interest. Now, some are going a step further by augmenting sales teams with machine learning algorithms that can comb through large datasets to flag up corporate risk management needs.
This is the world of risk advisory robots, which aim to put relationship managers on the front foot by absorbing information that might otherwise be missed and delivering analysis to drive new sales opportunities. All in the time it takes to make a cup of coffee.
“If you were to have analysts covering 75 assets and asking them to provide outputs every day, you would need a team of reasonable size. Whereas with this process we can have all that analysis done within minutes,” says Michael Sneyd, the global head of quantitative and derivative strategy at BNP Paribas in London.
BNP Paribas, HSBC, Nomura, Standard Chartered and Societe Generale all have live projects exploring the capability of machine learning and artificial intelligence in helping predict corporate sales opportunities.
Projects currently afoot are diverse. One aims to assess a corporate’s hedging behaviour against an average of its peers. Another monitors historical trigger points such as a shift in a corporate’s bond curve that have prompted the client to trade a swap in the past. A third aims to identify mispriced assets – and potential strategic opportunities – by matching a variety of pricing models to prevailing market prices.
The development is part of a wider digital transformation taking place across the front office as banks find new ways to make the salespeople and traders quicker, smarter and cheaper. Big data projects have been underway since 2017, initially embraced by market-making businesses in an effort to identify customers for a specific trade idea or an axe. New technology, which combines pools of data from across the bank with external information to determine the best course of action for specific clients, is bringing that revolution closer to the world of risk solutions and advisory.
Depending on the bank, the information collected could range from external market data from public databases and vendors, to internal data on existing positions with clients and customer relationship management (CRM) systems. In the future some banks see this list expanding to include information from email communications, public news and speeches extracted using natural language processing techniques (NLP) (see box: AI tools of the trade).
“We started to build a few initiatives on the [market risk advisory] side – where we work closely with our clients in looking at and understanding their intrinsic exposures related to market risk – to predict behaviour, to enlarge databases, to make some interpretations of actions that are taken by corporates to be able to deduce them quicker,” says Pascale Moreau, the global head of corporates fixed income and currencies sales at Societe Generale Corporate & Investment Banking (SG CIB) in Paris. “Until now most of the work was done from a human point of view… now what we want is to build a machine that will be replicating that system and then giving the information to all the sales,” she says.
Societe Generale went live with its machine learning tool for corporate sales in September 2018.
If you were to have analysts covering 75 assets and asking them to provide outputs every day, you would need a team of reasonable size. Whereas with this process we can have all that analysis done within minutes
Michael Sneyd, BNP Paribas
Nomura is at the proof-of-concept stage with a project that channels external information including market data into the bank’s CRM systems, which house data relating to a banker’s interaction with the client.
“This will all be built into our CRM platform as we have the foundational layer. I don’t want 17 dashboards delivering lots of different content to bankers. I want to try and bring that together as much as we can,” says Mark Chapman, head of technology in the investment banking arm of Nomura in London.
The investment bank is using a solutions vendor to provide the so-called augmented intelligence (AI).
Others are building the analytics themselves. BNP Paribas has established a quant analytics team, which applies machine learning tools to analyse market data and inform multiple teams within the bank – including both corporate and institutional sales – on the market factors that are most relevant at any point in time, and which ones are correlated.
‘Cross-pollination’
“What’s useful about these quant processes is they can absorb information that otherwise would be missed, particularly if you think in a qualitative setup. If an analyst is, say, focused on covering G10 forex they won’t have the bandwidth to also follow what’s happening in rates and equities. However, by having a quant process it’s easy to get the cross-pollination of ideas and correlations between different asset classes,” says BNPP’s Sneyd.
This should change the nature of the relationship between sales and clients. Previously banks’ siloed structures meant a sales person would not necessarily have access to the relevant analytics tools available to match clients’ needs in an evolving market environment.
For example, certain market events may give rise to very specific hedging needs across different asset classes. This information would not be readily available to sales.
Using AI to provide these analytics tools can open up opportunities for improved quality and relevance of service, according to Kasper Christofferson, global head of electronic market solutions at Standard Chartered in London.
In addition, client coverage may get split between low-touch and high-touch segments in the future. Servicing the former is already being automated. To deal with high-touch clients, sales may be armed with advanced analytics to increase the relevance of their service.
For the higher-touch segments, sales will consume various forms of advanced data analytics to enhance outcomes for clients further up the value chain
Kasper Christofferson, Standard Chartered
“For the higher-touch segments, sales will consume various forms of advanced data analytics to enhance outcomes for clients further up the value chain. I think we will see this manifest itself across the industry more explicitly,” adds Christofferson.
HSBC has created a new client intelligence unit that aims to apply machine learning to a 10-petabyte pool of internal data, allowing salespeople to respond to client needs and identify opportunities that may typically have been missed.
Data vendors are also tapping into the action, Refinitiv – formerly the financial and risk business of Thomson Reuters – offers tools that combine a bank’s own customer data with data Refinitiv has on these firms in a commingled environment. The firm has partnered with AI vendor Squirro to provide machine learning-based analytics to many major banks based on its market data, which is integrated into banks’ own CRM systems.
“If something new happens, [such as] a new corporate event in the market that affects a company, [we can] alert that to the banker who is looking at this company… the system learns by being told when it does well and being told when it doesn’t do so well, sending those alerts and those reasons to call,” says Leon Saunders Calvert, head of M&A and capital-raising propositions at Refinitiv in London.
The sales team can communicate feedback on which information provided by the tool was most relevant for them, helping to improve the overall effectiveness of alerts.
High alert
The key goal behind the use of machine learning for sales is to provide the most relevant information in a quick and efficient manner and inform salespeople how and when to approach clients.
For instance, SG CIB’s tool can alert a salesperson on certain behavioural patterns of the client, such as trading a specific product less, for instance. If the volume is decreasing, sales could call up the client to find out why and whether they can serve the client differently.
For example, a client may be trialling an alternative fintech provider, causing it to reduce the amount of flow it is putting through bank systems. Alternatively, a change in corporate structure may have caused a client to reduce certain exposures it would normally hedge.
“It opens a dialogue, and we can propose to the client something which is more adapted, for instance… it allows us to arrive at the right time,” says SG CIB’s Moreau.
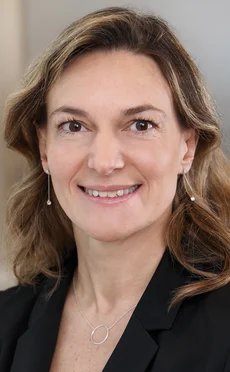
The bank rolled out its machine learning-based alerting tool in September last year, beginning with trade finance data. Details of a specific trade and the corporate involved, such as its importance to the bank and the firm’s overall size, are delivered to relevant sales desks.
Alerting can work in other ways, for example by sending relevant news flow about a particular corporate, ultimately cutting down the valuable time it takes to read information from a vast array of sources. Refinitiv’s tool integrates this into the CRM system, while the machine-learning algorithm developed by Squirro decides what type of information is relevant.
“You don’t have to be in some way an expert on looking at various different sources and interrogating different databases. You only need to know what’s important to you, whether it’s the markets a certain company is in, the peers they have, the movement of people – this all gets sent your way,” says Refinitiv’s Saunders Calvert.
Nomura’s investment banking arm, for instance, is planning on feeding this kind of information through the bank’s CRM systems, but in such a way that each team has access only to the recommendation on who they should be calling – the data is not pooled together from across different teams due to restrictions around who can see what.
“We obviously have strict information barriers… and we have to be quite protective around our data, so it makes it difficult to pool data together. It’s better for us to find intelligence that can cut across that data,” says Chapman.
Mixing external information – whether market data or news flow – with internal data sources can create a powerful combination. A bank’s CRM database houses details around the client-banker relationships, project information, past deals and call notes. Machine learning algorithms are able to extract the relevant information from the database and combine it with market data to find matches between market conditions and past client interest.
For example, if historically there was client interest in a particular activity and that hits a certain trigger point, it may be time to call up the client.
“One of the key features is your historical call notes, but it will also be looking at email interactions and other trigger points, then extracting that and using the information to scan the market,” says Chapman.
“You can do it two ways. You can do a market scanning and layer that across your infrastructure to try and find matches, or, more intelligently, you can understand what your landscape is, what the call notes you have are, and the information you have within your internal systems, and match those to an external event.”
Quantitative analytics is another area where machine learning can help sales work faster. BNPP’s research division has a team that employs quantitative and analytics tools that scan public market data and identify relevant market factors. The tool scans 75 different assets to come up with factors such as a specific interest rate or a currency that is primarily driving the market.
We obviously have strict information barriers… and we have to be quite protective around our data, so it makes it difficult to pool data together. It’s better for us to find intelligence that can cut across that data
Mark Chapman, Nomura
“We span across the major asset classes and then extract what variables can explain most of the variation across the markets,” says Sneyd. “A person doesn’t have the capability to track every single variable in the markets but by having this quant process we are providing you with a smaller list of variables, which as a qualitative analyst you should be following closely.”
Although this is primarily to help institutional clients with alpha generation, the information feeds through from research to all sales teams, which can act quickly.
“They are our distributional channels so they then promote the publication with clients… [and] with traders so they are aware what variables we think will move the market,” says Sneyd.
BNPP has also developed machine learning applications for the analysis of economic data published by statistical agencies in the US. This aims to discover alternative indicators on the state of the economy.
“We are using APIs from public statistical data… and then using AI-type approaches to look at trends and turning points in the data and really crunch through the details to then provide an aggregated series of what the status of the US economy is at this point,” says Sneyd.
The team also uses machine learning techniques to identify mispricing of assets in the market by running a large number of variations of pricing models and matching that to market prices. If an arbitrage is identified, sales may speak to the client about developing a strategy around that.
“There is a signal that an asset from our process is looking cheap versus what we deem to be the fair value. Then one of the roles of the salesforce is to highlight to clients that have that exposure that we see the asset is cheap and they should perhaps consider a trading or a hedging strategy around that,” adds Sneyd.
For each asset, two sets of fair values are generated depending on which client the dealer thinks would be most interested in the output. There is one fair value for higher-frequency clients and another fair value where the signal tends to last for one to three months for clients who want trades to last a little bit longer. Sneyd says it takes about 10 minutes to provide fair values across 75 assets.
Correlated assets are also flagged up so traders and analysts working in rates, for example, can drive sales in another asset class if there is a correlation.
AI tools of the trade
The most popular AI tools currently being applied by corporate sales teams are clustering, a type of unsupervised machine learning technique that tries to find groupings within datasets based on some similarities, and random forests, a supervised learning method which can classify things using many decision trees.
At SG CIB both techniques are used to group together corporate clients on the basis of their commonalities. One application of this is assessing whether the hedging behaviour of a corporate is in line with that of the group’s average. If not, it could be an opportunity to advise the client on a trade, or series of trades.
“[We are trying] to build on the corporate profile some kind of comparison to put them into a regression to build a sectorial pattern and to see the ones which are different from the general pattern of the sector,” says SG CIB’s Pascale Moreau.
The bank also uses techniques such as web scraping to extract information on corporates from public websites, and sentiment analysis to extract information on the back of M&A deals.
Standard Chartered also uses, among other techniques, advanced clustering based on both internal and external market data.
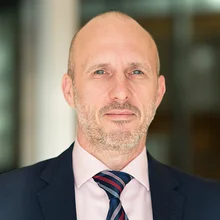
BNP Paribas, on the other hand, uses an optimisation algorithm based on decision trees to provide its market analytics. “In our case we run this on economic data and then set an objective function. We want to look at turning points in economic data, then this optimiser provides you with the output that corresponds with that [function],” says Michael Sneyd.
While existing applications of machine learning at banks revolve around simple techniques to find patterns in data, there are more ambitious projects in the works.
Many dealers are exploring the use of natural language processing to extract meaningful information from financial statements or general news flow, for instance.
“Natural language processing will help us read the financials of corporates so that we can integrate them into some pro forma profiles, then we are trying to keep writing some simple patterns of behaviour. We would like to incorporate AI to answer some simple situations coming from a change in corporate profile. For instance, we would like to provide free advisory on some simple situations,” says SG CIB’s Moreau.
The simple situations would mainly include low-touch clients and smaller exposures, she adds.
Nomura is exploring NLP to extract information from emails. The bank is also considering the option of extracting data from voice-call notes in the future, but there is a risk that the calls are stored and processed outside the bank – a potential data security hazard.
“We are working with a couple of companies as to how we can potentially do that internally,” says Nomura’s Mark Chapman.
Only users who have a paid subscription or are part of a corporate subscription are able to print or copy content.
To access these options, along with all other subscription benefits, please contact info@risk.net or view our subscription options here: http://subscriptions.risk.net/subscribe
You are currently unable to print this content. Please contact info@risk.net to find out more.
You are currently unable to copy this content. Please contact info@risk.net to find out more.
Copyright Infopro Digital Limited. All rights reserved.
You may share this content using our article tools. Printing this content is for the sole use of the Authorised User (named subscriber), as outlined in our terms and conditions - https://www.infopro-insight.com/terms-conditions/insight-subscriptions/
If you would like to purchase additional rights please email info@risk.net
Copyright Infopro Digital Limited. All rights reserved.
You may share this content using our article tools. Copying this content is for the sole use of the Authorised User (named subscriber), as outlined in our terms and conditions - https://www.infopro-insight.com/terms-conditions/insight-subscriptions/
If you would like to purchase additional rights please email info@risk.net
More on Markets
Vanguard stages swaptions comeback
Counterparty Radar: Deutsche grew book to $11 billion in Q1 to become largest non-US swaptions dealer to mutual funds
Data reveals hidden clockwork of FX forwards market
More than 70% of Vanguard’s volumes and nearly half of Pimco’s regularly occur on just four calendar days
RJ O’Brien plots expansion into US Treasury clearing
Chairman and CEO says futures house plans to capitalise on SEC’s new mandate for interdealer trades
Premialab adopts CDM for QIS swap booking
Vendor selects open source data model to power expansion in growing systematic index market
Is JSCC-CFTC stalemate about to be broken?
Japan CCP gains allies in battle to clear yen swaps for US clients, but CFTC shakeup could dash hopes
What T+1 risk? Dealers shake off FX concerns
Predictions of increased settlement risk and later-in-the-day trading have yet to materialise
How steepener trades burned hedge funds, and what happened next
Delays to central bank rate cuts torpedo popular trade, causing funds to pull capital – to the chagrin of sell-side desks
Covid-induced Eurobonds mark step towards EU financial cohesion
Successful issuance points to greater pan-European sharing of risk
Most read
- Harvesting the FX skew premium
- How steepener trades burned hedge funds, and what happened next
- House of cards? The $3 trillion (non-systemic) real estate risk