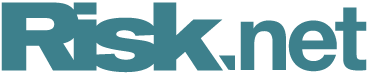
Podcast: Man Group’s Zohren on forecasting prices with DeepLOB
Deep learning model can project prices around 100 ticks into the future
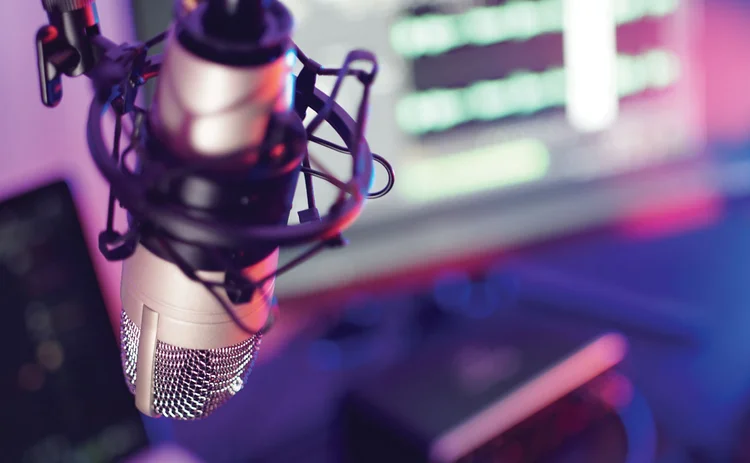
Limit order book (LOB) data has long been a source of fascination and frustration for market makers and high-frequency traders. The log of bids and offers in the market undoubtedly contains pertinent information about the next tick in the price of an asset. But the data is so voluminous that it is cumbersome to analyse using traditional techniques.
Quants at the Oxford-Man Institute are using deep learning to tackle this problem. Our guest for this episode of Quantcast is Stefan Zohren, an associate professor at the Oxford-Man Institute and principle quant in charge of futures and foreign exchange execution research at Man Group’s central trading division. He has been working on the project with Zihao Zhang and Stephen Roberts at the Oxford-Man Institute.
The technique they have developed is called DeepLOB. In essence, it uses ideas borrowed from image recognition and applies them to financial data. The underlying principle is that an algorithm can recognise shapes in the LOB in the same way it identifies images.
“Imbalances [in the LOB] are the kind of features that these models are able to extract,” says Zohren.
DeepLOB can predict forecast a price at a single future point in time and has already been adopted by a number of financial institutions, including Man Group. In their most recent paper, Zohren and Zhang describe how the technique can be adapted to generate multi-period forecasts.
The innovation in their latest work is the implementation of techniques, such as sequence-to-sequence and attention models, that can learn longer-term relationships and extract features with longer memories. This allows forecasts to be projected further into the future.
The forecasting horizon of the adapted model can extend to about 100 ticks into the future, which in a liquid market might translate to around 30 seconds.
Zohren explains this methodology could be combined with reinforcement learning to compute the paths of optimal execution. That might be the subject of a future study.
Index
00:00 LOBs and the problem of unmanageable data
03:00 Using deep learning to manage LOB data
07:07 Sequence-to-sequence, attention models and long memory
15:10 Real-world applications
To hear the full interview, listen in the player above, or download. Future podcasts in our Quantcast series will be uploaded to Risk.net. You can also visit the main page here to access all tracks, or go to the iTunes store or Google Podcasts to listen and subscribe.
Now also available on Spotify.
Only users who have a paid subscription or are part of a corporate subscription are able to print or copy content.
To access these options, along with all other subscription benefits, please contact info@risk.net or view our subscription options here: http://subscriptions.risk.net/subscribe
You are currently unable to print this content. Please contact info@risk.net to find out more.
You are currently unable to copy this content. Please contact info@risk.net to find out more.
Copyright Infopro Digital Limited. All rights reserved.
As outlined in our terms and conditions, https://www.infopro-digital.com/terms-and-conditions/subscriptions/ (point 2.4), printing is limited to a single copy.
If you would like to purchase additional rights please email info@risk.net
Copyright Infopro Digital Limited. All rights reserved.
You may share this content using our article tools. As outlined in our terms and conditions, https://www.infopro-digital.com/terms-and-conditions/subscriptions/ (clause 2.4), an Authorised User may only make one copy of the materials for their own personal use. You must also comply with the restrictions in clause 2.5.
If you would like to purchase additional rights please email info@risk.net
More on Cutting Edge
Overcoming Markowitz’s instability with hierarchical risk parity
Portfolio optimisation via HRP provides stable and robust weight estimates
Funding arbitrages and optimal funding policy
Stochastic control can be used to manage a bank’s net asset income
Quantum two-sample test for investment strategies
Quantum algorithms display high discriminatory power in the classification of probability distributions
Market-making in spot precious metals
A market-making framework is extended to account for metal markets’ liquidity constraints
Choosing trading strategies using importance sampling
The sampling technique is more efficient than A-B testing at comparing decision rules
A comparison of FX fixing methodologies
FX fixing outcomes are mostly driven by length of calculation window
Quantum cognition machine learning: financial forecasting
A new paradigm for training machine learning algorithms based on quantum cognition is presented
Backtesting correlated quantities
A technique to decorrelate samples and reach higher discriminatory power is presented