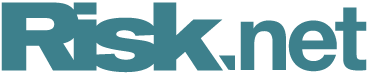
Podcast: Kondratyev and Schwarz on generating data
Market generator models may aid areas of finance where data is limited or sensitive, by generating new data with the same statistical properties.

In this episode of Quantcast, I talk to Alexei Kondratyev, head of the data analytics group at Standard Chartered Bank, and his colleague Christian Schwarz, executive director in the same team.
Kondratyev and Schwarz have co-authored The market generator, in which they introduce a technique to generate synthetic market data that preserves the statistical properties of the original data.
It is a big leap from traditional simulation models, such as those that use Monte Carlo. “The difference between our approach and what was traditionally done in Monte Carlo simulation frameworks is that rather than relying on parametric modelling, we advocate a non-parametric modelling,” Kondratyev explains, implying that the learning process of market scenarios, risk factors and probability are also non-parametric.
The model uses a specific type of generative neural network, called the restricted Boltzmann machine (RBM), to sample from an existing dataset of risk factors and generate a synthetic dataset that replicates the dependence structure of the original data. It is a key achievement that was not possible with parametric models. The ability to capture varying correlations between risk factors is crucial for managing risk and portfolios, and even more so around tail events, when linear correlations break down.
Asked why it is only now possible to implement computationally demanding techniques such as this one, Schwarz says: “There’s a lot more computing power available now that allows us to calibrate this type of machine learning model.
The potential applications of the model are numerous and go beyond the simulation of market data for risk management or other financial purposes.
“You can think of the market generator as a data anonymiser,” says Kondratyev. “For example, data anonymisation in the medical data context is a very important application.”
Schwarz adds that another application is connected to reinforcement learning techniques such as agent-based ones, where the environment where the agent is supposed to learn and act upon is simulated. “In situations where you do not have a lot of data, you can potentially learn the probability distribution of the underlying data and have the agent learn from both real data and synthetic data.”
Index
00:00 Intro
01:45 What is a market generator?
03:15 What are the principles behind this new simulation technique?
08:05 How does your market generator work?
12:22 Application to FX markets and capturing of dependence structures
16:50 Non-financial applications
19:47 Model validation
21:45 Other market generator models
22:43 Model development
To hear the full interview, listen in the player above, or download. Future podcasts in our Quantcast series will be uploaded to Risk.net. You can also visit the main page here to access all tracks, or go to the iTunes store or Google Podcasts to listen and subscribe.
Only users who have a paid subscription or are part of a corporate subscription are able to print or copy content.
To access these options, along with all other subscription benefits, please contact info@risk.net or view our subscription options here: http://subscriptions.risk.net/subscribe
You are currently unable to print this content. Please contact info@risk.net to find out more.
You are currently unable to copy this content. Please contact info@risk.net to find out more.
Copyright Infopro Digital Limited. All rights reserved.
As outlined in our terms and conditions, https://www.infopro-digital.com/terms-and-conditions/subscriptions/ (point 2.4), printing is limited to a single copy.
If you would like to purchase additional rights please email info@risk.net
Copyright Infopro Digital Limited. All rights reserved.
You may share this content using our article tools. As outlined in our terms and conditions, https://www.infopro-digital.com/terms-and-conditions/subscriptions/ (clause 2.4), an Authorised User may only make one copy of the materials for their own personal use. You must also comply with the restrictions in clause 2.5.
If you would like to purchase additional rights please email info@risk.net
More on Cutting Edge
The WWR in the tail: a Monte Carlo framework for CCR stress testing
A methodology to compute stressed exposures based on a Gaussian copula and mixture distributions is introduced
Estimating mean reversions in interest rate models
The speed of factors’ mean reversion in rate models is estimated
Auto-encoding term-structure models
An arbitrage-free low-dimensionality interest rate model is presented
The relativity of the fractional Gamma Clock
Bank of America quant expands his Gamma Clock model with a fractional Brownian motion
A market-making model for an options portfolio
Vladimir Lucic and Alex Tse fill a glaring gap in European-style derivatives modelling
Option market-making and vol arbitrage
The agent’s view is factored in to a realised-vs-implied vol model
Degree of influence 2024: volatility and credit risk keep quants alert
Quantum-based models and machine learning also contributed to Cutting Edge’s output
Overcoming Markowitz’s instability with hierarchical risk parity
Portfolio optimisation via HRP provides stable and robust weight estimates