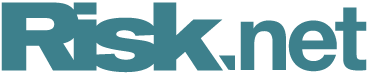
Podcast: Princeton’s Carmona on the future of quant education
Course director discusses machine learning explainability and reclaiming game theory from economists
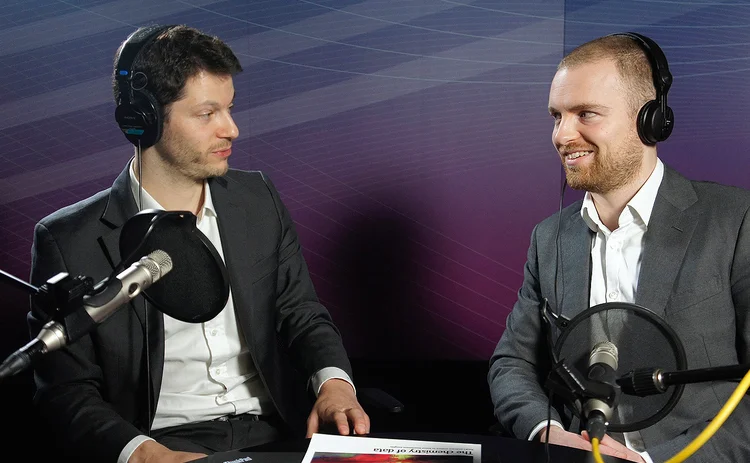
In a wide-ranging Quantcast interview with Risk.net, René Carmona – director of Princeton’s master’s in finance programme – discusses everything from the impact of Trump administration policies on foreign students’ desire to study in the US, to his efforts to reclaim the field of game theory from being the sole preserve of economists.
Carmona, who has spent some five decades in mathematical academia, shares his thoughts on what has helped Princeton’s programme to succeed: the university has topped a number of industry polls, including Risk.net’s 2019 industry guide. He lists three factors: the amount of time core faculty members spend teaching on its courses; the relatively small size of its intake, at 25; and perhaps above all, what he dubs its secret weapon: its rich network of alumni, many of whom, like hedge fund manager Dario Villani, have gone on to build lofty careers in quant finance, before returning to teach, mentor and even help select the graduates who study on the programme.
“The Princeton alumni network is extremely powerful; they’re devoted to this institution. They spend a lot of time and a lot of money on the institution even years after leaving; we take full advantage of their presence and their desire to help,” says Carmona.
Princeton has a head start in this regard as one of the oldest dedicated quant finance master’s programmes, having first opened its doors in 1998. Asked about the biggest change he has seen in quant finance education in the intervening two decades, Carmona points to the impact the crisis had on the assumptions underlying pricing models for many derivatives products – viewed by some as the nadir of the quant profession – such as the Gaussian copula formula, widely blamed for the mispricing of trillions of dollars’ worth of credit derivatives and correlation trades.
“Some of the changes in the industry, we saw coming – and we worked to include these problems in our courses,” he says. “When the first worries about measures of risk occurred in the industry, we started teaching heavy-tailed distribution – various forms of dependencies and copula – even before the credit markets took off, and the copula became so central there.”
In part, he attributes the programme’s adaptiveness to industry trends to the varied input it receives from staff across multiple faculties: it is hosted by Princeton’s Bendheim Center, rather than an individual faculty. Courses thus receive guidance from academics based in a host of departments: mathematics and engineering, but also economics, computer science and operational research.
Turning to one of the fastest-growing areas of research in quant finance – one Princeton and many of its peers have added courses on – the teaching of machine learning, Carmona argues the rapid growth of the field is unlikely to decline any time soon.
In fact, he points to one of the largest areas of debate in the field at present – the challenge of explaining how models based on the self-learning techniques, particularly those plucked from the deep learning family of approaches, have arrived at their conclusion, which is increasingly seen as imposing a natural check on further development in the field – as a natural opportunity for quant academia.
The profession is well placed to provide the research power needed to develop machine learning algorithms that are more transparent, he argues: “I think there is an enormous opportunity for academics and practitioners alike to get involved.”
Turning lastly to his current areas of research, Carmona discusses his recent work on probabilistic theory in mean field games – a branch of game theory that deals with individual decision-making within large groups. Carmona published two 700-page tomes on the subject last year.
“Many colleagues find it absolutely insane,” he jokes, “[but] I thought it was a wonderful way for mathematicians to try and understand the behaviour of large crowds: pedestrians, birds flocking – or herds of traders trying to get into a congested trade,” he says. “I believe there are an enormous number of possible applications. Mathematicians have neglected game theory; John Nash got a Nobel Prize, but he got it in economics, before being recognised by mathematicians. Game theory has basically been handed over to economists, who teach it – very few maths departments do.”
Commentary by Tom Osborn
Index
00:00 Intro
01:20 Drivers of a successful master’s programme in quant finance
06:15 How has education in quant finance changed in the past few years?
11:35 Is there a gap between academia and industry?
15:13 P quants or Q quants? Where do European courses have room for improvement?
21:07 Is Princeton preparing more grads for the buy side?
24:05 The alumni network
26:15 Teaching of ML techniques: a long-lasting trend, or will it fade?
36:35 Brexit and US immigration policy: what is their effect on the influx of students in the US education system?
39:17 Current research on probabilistic theory on mean field games
To hear the full interview, listen in the player above, or download. Future podcasts in our Quantcast series will be uploaded to Risk.net. You can also visit the main page here to access all tracks, or go to the iTunes store or Google Podcasts to listen and subscribe.
Only users who have a paid subscription or are part of a corporate subscription are able to print or copy content.
To access these options, along with all other subscription benefits, please contact info@risk.net or view our subscription options here: http://subscriptions.risk.net/subscribe
You are currently unable to print this content. Please contact info@risk.net to find out more.
You are currently unable to copy this content. Please contact info@risk.net to find out more.
Copyright Infopro Digital Limited. All rights reserved.
As outlined in our terms and conditions, https://www.infopro-digital.com/terms-and-conditions/subscriptions/ (point 2.4), printing is limited to a single copy.
If you would like to purchase additional rights please email info@risk.net
Copyright Infopro Digital Limited. All rights reserved.
You may share this content using our article tools. As outlined in our terms and conditions, https://www.infopro-digital.com/terms-and-conditions/subscriptions/ (clause 2.4), an Authorised User may only make one copy of the materials for their own personal use. You must also comply with the restrictions in clause 2.5.
If you would like to purchase additional rights please email info@risk.net
More on Risk management
The changing shape of risk
S&P Global Market Intelligence’s head of credit and risk solutions reveals how firms are adjusting their strategies and capabilities to embrace a more holistic view of risk
To liquidity and beyond: new funding strategies for UK pensions and insurance
Prompted by policy shifts and macro events, pension funds and insurance firms are seeking alternative solutions around funding and liquidity
More cleared repo sponsors join Eurex ahead of cross-margining
End of TLTROs for banks and pension fund search for liquidity management tools drives uptake
Reimagining model risk management: new tools and approaches for a new era
A collaborative report by Chartis and Evalueserve on how the use of automation can combat the growing complexity of managing model risk due to regulation and market volatility
What Goldman’s appeal victory means for Fed stress tests
Decision could embolden more banks to appeal, analysts say. But others believe result is one-off
Clearing members rattled as CME approved to launch its own FCM
National Futures Association registration sharpens concerns about conflict of interest with CCP
CME files application for US Treasury and repo clearing
New entrant believes direct user access model will avoid accounting problem that hampers rival FICC
UST repo clearing: considerations for ‘done-away’ implementation
Citi’s Mariam Rafi sets out the drivers for sponsored and agent clearing of Treasury repo and reverse repo