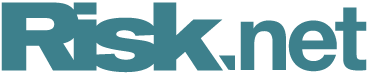
Paper of the year: Peter Mitic and Jiaqi Hu
OpRisk Awards 2020: Quants recognised for VAR approach to modelling conduct risk with scant data
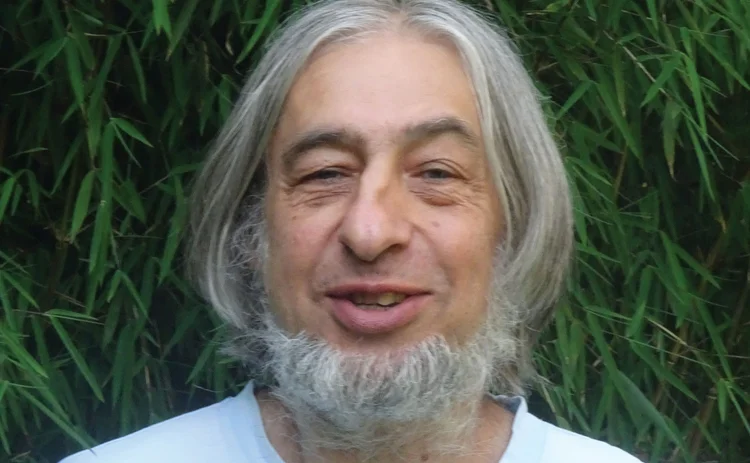
The abandonment of the model-based calculations for operational risk capital does not mean the end of op risk modelling – instead, it seems to have freed up op risk modellers to look at issues other than the narrow problem of capital levels. The result has been an astonishing burst of creativity, as modellers take a fresh look at how op risk behaves – and address some of the less tractable problems it presents.
The advanced measurement approach for calculating operational risk capital, introduced as part of the Basel II capital framework, divided operational risk losses into seven categories. But for op risk quants, the important divide now seems to be into two: conduct losses, and everything else. Non-conduct risk losses have proved more amenable to modelling: dimensional analysis shows that, regardless of their Basel category, all types of non-conduct risk behave in a similar way.
Conduct risk, however, is different.
“We have always been troubled by modelling conduct risk,” says Peter Mitic, head of operational risk methodology at Santander Bank UK, and winner of the Paper of the year award.
“We have been using the same techniques to model conduct risk as we use for the other operational risk classes. In that sense, conduct risk seems to be the same as all the other risk classes. Yet conduct risk is different in the sense that it has been dominated by severe losses, principally due to payment protection insurance [PPI]. Such large losses have resulted in huge VAR values, which we have considered disproportionate.”
The 2020 Operational Risk Paper of the year, published in the Journal of Operational Risk in December 2019, addresses this crucial problem. In Estimation of value-at-risk for conduct risk losses using pseudo-marginal Markov chain Monte Carlo, Mitic and Jiaqi Hu, a graduate student in Oxford University’s statistics department, suggest that banks should break up these massive losses into hundreds or thousands of individual loss events.
Marcelo Cruz, editor of the Journal of Operational Risk, comments: “Measuring and managing conduct risk is difficult, as there are not many loss events, but quite a lot of daily regular tasks performed by client-facing employees can trigger conduct risk events. The authors devised a very elegant mathematical model that uses any little available data to estimate conduct risk in financial institutions.”
Conduct risk is different in the sense that it has been dominated by severe losses, principally due to PPI. Such large losses have resulted in huge VAR values, which we have considered disproportionate
Peter Mitic, Santander Bank UK
Breaking up larger losses is sometimes easy – if the loss is actually made up of a large number of small payouts. But in most cases, it can’t. Basel Committee on Banking Supervision rules require that losses used for modelling should be listed on a balance sheet.
However, Mitic and Hu point out: “In this context, the provisions are the balance sheet items, not the individual payments to customers. Therefore, it is the provisions that should be modelled. In most cases, it is not possible to decompose any given provision into individual payments. Those payments are usually made against a current set of provisions, not against any particular member of the set.”
At the height of the PPI mis-selling scandal in the UK, for example, banks were provisioning against expected payouts at the rate of hundreds of millions of pounds per quarter – the payouts themselves would follow only months or years later.
However, the right artificial breakdown can provide similarly robust results, even when a loss figure cannot be naturally split up, argue Mitic and Hu.
The method they suggest involves using Bayesian methods and a Markov chain approach to determine lognormal parameters for the distribution of the partitioned losses. They suggest experimenting with several different numbers of partitions – the results may converge on a single value of VAR as the number of partitions increases, after varying drastically during the first few partitions.
Almost all the datasets tested – including four sets of internal Santander loss data, data from loss data specialist ORX, and a synthetic sample – showed initial wide swings followed by convergence on a stable value once the number of partitions reached several thousand. The four internal datasets converged on the same value of VAR when the largest loss in each was partitioned – reflecting the fact that all represent the same risk profile, the authors point out. Determining this convergent value, they warn, means assuming that the decline towards it follows an exponential distribution – which may not be the case.
And another pitfall is represented by using the method inappropriately on a dataset that is not dominated by a single extreme loss. They simulated this by using a synthetic distribution – which therefore had a known ‘true’ lognormal distribution – and removing its largest point. Instead of the “correct” VAR of £640 million ($800.1 million), their method produced a much lower £92 million figure. Again, user judgement is the only safeguard against this risk, write Mitic and Hu.
Once their method has produced a suitably rich loss dataset, it allows the use of conventional modelling methods to derive a credible VAR figure. Here, again, op risk modellers will need to rely heavily on their own judgement.
The authors acknowledge that the definition of a ‘reasonable’ VAR is inevitably subjective. They suggest comparing the VAR derived from the unmodified data with figures at other similar institutions, or comparing the VAR result with a minimum acceptable VAR derived from an empirical bootstrap method (although this provides only a minimum and not a maximum).
“There is no objective standard for assessing a maximum value for VAR given a set of losses,” they write, “other than a general idea of what the reserve should be”.
The next step, Mitic says, will be to simplify the method further by focusing on VAR calculations only for the areas towards the tail of the distribution of VAR values – the areas where VAR has already converged on its stable value.
“A provision implies an unknown number of future payouts, each of an unknown amount. Doing a VAR calculation for multiple cases is very time-consuming. However, we have established that there are cases that we should look at preferentially. Effectively, they account for a large number of small payouts, rather than a small number of large payouts … so the way forward is to look at the region corresponding to a high number of payouts, and to make a decision as to what the limiting value should be in cases when it’s not obvious. A simple average of a few cases should suffice,” says Mitic.
Unfortunately, he adds, those cases take the longest time to calculate – “you can’t have everything”.
And, he adds, it’s also possible that conduct risk could become less abnormal in the next few years.
“The technique is appropriate when one or a few huge losses dominate all of the others and is particularly useful if there is a very small number of losses. So, as PPI and similar effects drop out of the calculation window, conduct risk losses resemble other risk classes much more. A special treatment then becomes less relevant. That is, until the next big scandal arrives.”
Only users who have a paid subscription or are part of a corporate subscription are able to print or copy content.
To access these options, along with all other subscription benefits, please contact info@risk.net or view our subscription options here: http://subscriptions.risk.net/subscribe
You are currently unable to print this content. Please contact info@risk.net to find out more.
You are currently unable to copy this content. Please contact info@risk.net to find out more.
Copyright Infopro Digital Limited. All rights reserved.
As outlined in our terms and conditions, https://www.infopro-digital.com/terms-and-conditions/subscriptions/ (point 2.4), printing is limited to a single copy.
If you would like to purchase additional rights please email info@risk.net
Copyright Infopro Digital Limited. All rights reserved.
You may share this content using our article tools. As outlined in our terms and conditions, https://www.infopro-digital.com/terms-and-conditions/subscriptions/ (clause 2.4), an Authorised User may only make one copy of the materials for their own personal use. You must also comply with the restrictions in clause 2.5.
If you would like to purchase additional rights please email info@risk.net
More on Awards
Environmental products house of the year: ENGIE
ENGIE is driving change in energy transition, with a strong focus on renewable energy and the liberalisation of power markets in Apac, which presents significant long-term growth opportunities. In recognition of its efforts, ENGIE GEMS has been named…
Natural gas/LNG house of the year: ENGIE
ENGIE continues to expand its services to better serve firms in Apac dealing with the challenges of energy risk management and supply
FRTB management solution of the year: Bloomberg
Amid the diverging timeframes and complex requirements of FRTB, Bloomberg offers a consistent, comprehensive and customisable solution for Apac banks preparing for implementation
Newcomer of the year: Topaz Technology
Jon Fox and former colleagues formed Topaz Technology in 2015. Having seen many different systems and, in some cases, written and built a few themselves, there was always something missing, leading them to build a system that unifies risk reporting and…
Technology vendor of the year: Murex
As a technology vendor, Murex places adaptability front and centre of everything it does, constantly enriching its MX.3 platform to ensure institutions can respond to new market opportunities as soon as they spot them
Currency derivatives house of the year: Deutsche Bank
Asia Risk Awards 2024
Interest rate derivatives house of the year: Standard Chartered Bank
Asia Risk Awards 2024
Derivatives house of the year, Taiwan: CTBC Bank
Asia Risk Awards 2024