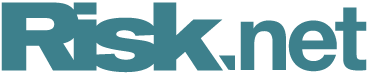
Baselines for applying machine learning to investing
Techniques that worked in the natural sciences may not translate well to financial markets
Buy-siders have been beating the bushes for ways to use machine learning in investing, only to be stymied by the flimsy quality and shortage of data at their disposal. Researchers have warned of the hazards of poorly buttressed efforts.
The newly founded quarterly Journal of Financial Data Science offers some guidance on this score.
The journal, created to address the relatively poor track record of algorithms in forecasting, is edited by Joseph Simonian, director of quant research at Natixis Investment Managers, Frank Fabozzi, professor of finance at the Edhec Business School, and Marcos Lopez de Prado, a principal at AQR Capital Management.
“Many people on the Street think that to do financial data science, you just take a machine learning algorithm and a data science model, and wholesale apply it to finance,” says Simonian. “But our argument, and the basis of this journal, is that financial data, whether it’s structured like time series data or unstructured, has its own peculiarities.”
A paper in the inaugural issue details how machine learning earned its track record in the physical sciences, which offer huge repositories of data. Unlike the natural sciences, where the ground rules do not change, capital markets are ever-moving, and even longstanding patterns can break down.
To work in finance, machine learning algorithms need much more information to spot enduring trends, especially in longer-horizon investing.
The paper, written by Campbell Harvey, a finance professor at Duke University and investment strategy adviser at Man Group, Rob Arnott, founder of smart beta pioneer Research Affiliates, and Harry Markowitz, a Nobel laureate in economics, proposed a checklist for applying machine learning techniques – in particular, determining whether there is enough data for machines to analyse.
Speaking to Risk.net in January, Arnott described using sparse data to train machine learning algorithms as akin to driving a Ferrari on a dirt track.
Among the reasons for the founding of the quarterly journal is to set some baselines. “Right now, everybody is throwing machine learning at any problem because it’s really popular,” says Harvey. “We need more discipline and the journal effectively imposes discipline.”
In another paper, Simonian and his colleagues at Natixis propose a machine learning approach to understanding how risk factors interact with each other.
The defining features of financial markets – mean reversion, momentum, implied volatility – are all connected to human nature, Simonian says, and any algorithm or data science framework applied to investing has to confront this reality.
That is not to say applications of machine learning in finance should be dismissed entirely. Another paper of which Harvey was a co-author explored how an independent Bayesian classifier, a machine learning technique, could be adapted for stock picking. The method has proved successful in categorising supernovas, which fits perfectly in terms of data requirements in investment.
“You’ve got something that’s worked in another area and it ports over very naturally into finance,” says Harvey.
That paper’s other co-authors are David Bew, principal engineer at Man AHL, Anthony Ledford, chief scientist at Man AHL, Sam Radnor, senior vice-president at quantPort, and Andrew Sinclair, senior quant analyst at Realindex Investments.
With enough material to train themselves, machine learning algorithms might master the peculiarities of the data used in finance, which is rooted in human nature. And oddly, some indications on how to do that may come from the gaming world. Google’s DeepMind computer became the top player of the board game Go – after competing against itself tens of millions of times. Investors, in contrast, have just one version of the past to learn from – in other words, only one possible unfolding of events.
But another game presents a more tantalising development for finance. In January, the DeepMind team announced it had built an engine capable of beating the best professional gamers at StarCraft II.
DeepMind describes the game as more like rock-paper-scissors than chess or Go because there is no single best strategy to follow. StarCraft is an strategy game that plays out in a virtual space where competitors are often in the dark about what is happening elsewhere in the game. Players have to make decisions in real time, choosing from an enormous number of available choices.
The artificial intelligence must rely instead on learning from successful strategies in past games and building its own intuition. The best strategy evolves as the game goes on.
And if all that sounded familiar, maybe the way is finally visible to learning algorithms that could master markets in a foreseeable future.
Only users who have a paid subscription or are part of a corporate subscription are able to print or copy content.
To access these options, along with all other subscription benefits, please contact info@risk.net or view our subscription options here: http://subscriptions.risk.net/subscribe
You are currently unable to print this content. Please contact info@risk.net to find out more.
You are currently unable to copy this content. Please contact info@risk.net to find out more.
Copyright Infopro Digital Limited. All rights reserved.
As outlined in our terms and conditions, https://www.infopro-digital.com/terms-and-conditions/subscriptions/ (point 2.4), printing is limited to a single copy.
If you would like to purchase additional rights please email info@risk.net
Copyright Infopro Digital Limited. All rights reserved.
You may share this content using our article tools. As outlined in our terms and conditions, https://www.infopro-digital.com/terms-and-conditions/subscriptions/ (clause 2.4), an Authorised User may only make one copy of the materials for their own personal use. You must also comply with the restrictions in clause 2.5.
If you would like to purchase additional rights please email info@risk.net
More on Our take
Podcast: Alexei Kondratyev on quantum computing
Imperial College London professor updates expectations for future tech
Quants mine gold for new market-making model
Novel approach to modelling cointegrated assets could be applied to FX and potentially even corporate bond pricing
Thin-skinned: are CCPs skimping on capital cover?
Growth of default funds calls into question clearers’ skin in the game
Quants dive into FX fixing windows debate
Longer fixing windows may benefit clients, but predicting how dealers will respond is tough
Talking Heads 2024: All eyes on US equities
How the tech-driven S&P 500 surge has impacted thinking at five market participants
Beware the macro elephant that could stomp on stocks
Macro risks have the potential to shake equities more than investors might be anticipating
Podcast: Piterbarg and Nowaczyk on running better backtests
Quants discuss new way to extract independent samples from correlated datasets
Should trend followers lower their horizons?
August’s volatility blip benefited hedge funds that use short-term trend signals