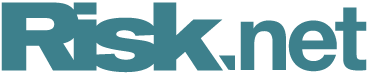
Quants look to image recognition to process alternative data
Funds are using machine learning to screen alternative datasets more quickly
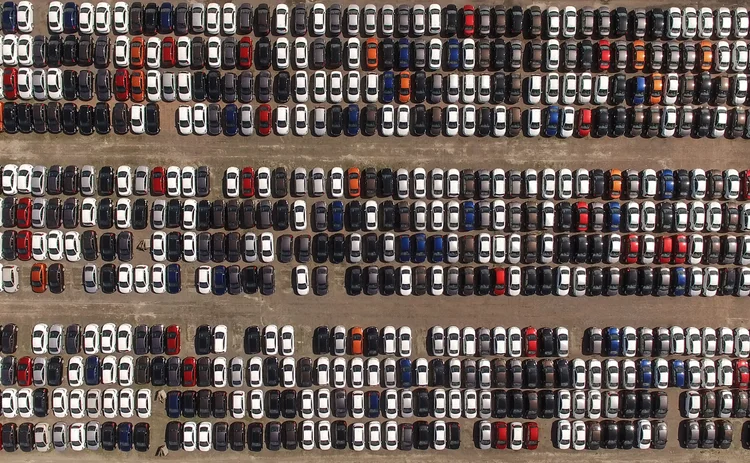
Quant funds are using machine-learning technology such as image recognition in their race to pick datasets for new investment strategies ahead of rivals.
Funds are expected to spend $7 billion a year by 2020 on so-called alternative data, such as satellite imagery and social media information, according to consultancy Opimas. But selecting datasets that contain the seeds of workable investment programmes can take months.
If funds lose time in processing new information, they risk rival funds discovering it too, said Elliot Noma, managing director of Garrett Asset Management, a commodity trading adviser based in New York.
“If someone else also figures out a feed is good, it becomes a race,” Noma said. “Not only [is the question] how quickly can I get the data, but how quickly can I process it and make the trade.”
Increasingly, funds aim to “fail quickly”, Noma said. If machine-learning techniques find nothing in the data, the fund can move on to analysing new datasets.
Noma was speaking at Risk.net’s Quant Summit in New York on July 11.
Four-fifths of investors are looking to buy alternative data, research firm Greenwich Associates says in a recent report. However, quants at JP Morgan have warned of the “blind alleys” awaiting managers if they waste time on datasets that do not contain alpha – either because they generate signals that have too little investment capacity or decay quickly, or because the data is simply too expensive.
As many as eight out of nine efforts to build strategies based on alternative data fail, Wesley Chan, director of stock selection research at Acadian Asset Management, told Risk.net in April.
Machine learning has long been used by firms such as Facebook to speed up the process of analysing and categorising images. For example, if an object has a nose, eyes and a mouth, an algorithm can be trained to recognise it as a face.
The same technology – known as feature extraction – might be applied to satellite images to identify features that can be used in quant investment strategies such as numbers of vehicles in shopping mall car parks or customers entering retail outlets.
When there is no previous model, or the quality of the data is suspect and other people are looking at the data and competing, machine learning is a major advantage in terms of speed
Elliot Noma, Garrett Asset Management
Garrett Asset Management is in the early stages of exploring the applications of machine learning to unstructured data, but estimates it could cut the feature extraction process from years and months to days or even hours.
“Alternative data has certain characteristics, but when there is no previous model, or the quality of the data is suspect and other people are looking at the data and competing, machine learning is a major advantage in terms of speed,” Noma said.
Elsewhere at the summit, Dilip Madan, a professor of mathematical finance at Robert H Smith School of Business at the University of Maryland, said automating data-intensive activities is one of the better applications of machine learning.
That contrasts with the struggle to apply machine learning in predictive models. “Even if a model is successful and learns something, five minutes later, the market changes and you have to learn again,” he said.
Others questioned the value of alternative data for quant funds as well as the potential of machine learning as a processing tool. “I am doubtful machines can be taught to replicate the creativeness of human analysis,” said an equity market neutral portfolio manager at a New York-based quantitative hedge fund.
Satellite imagery could be useful for macro traders, he said, but is “a waste of time” for equities traders and funds with large portfolios. Satellite images tend to focus on a small number of datapoints, so cannot be modelled robustly, he said.
Only users who have a paid subscription or are part of a corporate subscription are able to print or copy content.
To access these options, along with all other subscription benefits, please contact info@risk.net or view our subscription options here: http://subscriptions.risk.net/subscribe
You are currently unable to print this content. Please contact info@risk.net to find out more.
You are currently unable to copy this content. Please contact info@risk.net to find out more.
Copyright Infopro Digital Limited. All rights reserved.
As outlined in our terms and conditions, https://www.infopro-digital.com/terms-and-conditions/subscriptions/ (point 2.4), printing is limited to a single copy.
If you would like to purchase additional rights please email info@risk.net
Copyright Infopro Digital Limited. All rights reserved.
You may share this content using our article tools. As outlined in our terms and conditions, https://www.infopro-digital.com/terms-and-conditions/subscriptions/ (clause 2.4), an Authorised User may only make one copy of the materials for their own personal use. You must also comply with the restrictions in clause 2.5.
If you would like to purchase additional rights please email info@risk.net
More on Asset management
Execs can game sentiment engines, but can they fool LLMs?
Quants are firing up large language models to cut through corporate blather
Pension schemes prep facilities to ‘repo’ fund units
Schroders, State Street and Cardano plan new way to shore up pension portfolios against repeat of 2022 gilt crisis
Fears of runaway risk on offshore reinsurance
Life insurers catch the eye of UK regulator for pension buyout financing trick
Hot topic: SEC climate disclosure rule divides industry
Proposal likely to flounder on First Amendment concerns, lawyers believe
‘Brace, brace’: quants say soft landing is unlikely
Investors should prepare for sticky inflation and volatile asset prices as central banks grapple with turning rates cycle
Trend following struggles to return to vogue
Macro outlook for trend appears to be favourable, but 2023’s performance flop gives would-be investors pause for thought
Start-up bond platform OpenYield prepares to launch
Start-up aims to give retail brokers the same electronic liquidity used by the professionals
Can machine learning help predict recessions? Not really
Artificial intelligence models stumble on noisy data and lack of interpretability