Risk Technology Awards 2024: AI hopes and holdups
Live AI use-cases are limited, as vendors warn on over-regulation
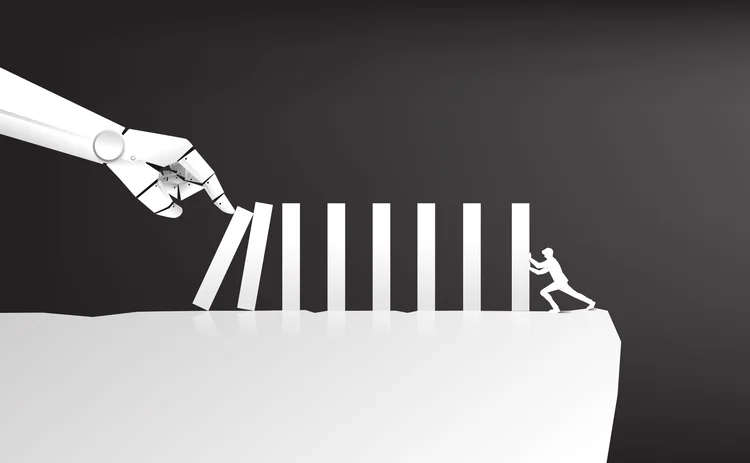
It’s natural to be excited when emerging technology promises to make life better. Often, the excitement continues as the graft begins. The vision of a better future is clear, almost tangible. But focus shifts to the practical reality.
This is where risk managers – and the vendors vying to serve them – are today when it comes to the promise of artificial intelligence.
The vision is compelling. But the reality involves grappling with data, legacy systems, knowledge gaps, computational capacity and spending constraints. Crucially, it also requires users of AI to convince regulators and supervisors, who worry about letting genies out of bottles.
“Staying at the cutting edge of AI is incredibly important for risk management and for threat detection, so it is dangerous to over-regulate AI to the point that innovation is stifled,” says Kristof Horompoly, head of AI risk management at ValidMind, which won the model validation service category in this year’s Risk Technology Awards (RTAs).
The concern is not just about blunting that cutting edge, though. Some vendors worry that mature uses of machine learning may also be caught in the dragnet.
We need institutions to come forward with working models that demonstrate to regulators the clear potential of AI
Kristof Horompoly, ValidMind
“People talk about AI as though it’s something new, that it has just been invented, but some of the really useful techniques for financial crime detection have been around for decades. I think there is a danger that very well-established technology could be made harder to use because of some of the controversy surrounding AI at the moment,” says Gabriel Hopkins, chief product officer at Ripjar, which provides screening solutions for banks in their fight against financial crime.
The tension between the technically possible and the practically permissible could be seen in this year’s Risk Technology Awards (RTAs). The categories cover a mix of domains, so it’s rare to see the same terms and phrases recurring across them – but in this year’s more-than-130 pitches, almost every document included some kind of reference to artificial intelligence, natural language processing, large language models, or machine learning.
If that sounds like evidence of a technological sea-change, it’s not – yet. Among the 19 winners, 15 pitches referred to AI in some shape or form. But only six of those were describing a live instance of the technology, and some of these were tried-and-tested applications, rather than a bold leap forward. Many of the other mentions were aspirational – use-cases where vendors think AI could or should be applied, one day.
The excitement is still palpable – one pitch suggested banks are on a road that will result in far more of their decisions being automated and model-driven, pointing to the example of other industries that have gone before them, such as insurance, healthcare, retail and telecoms. Equally palpable is the awareness of regulatory potholes in this road – another firm highlighted the “incredibly compelling use-cases” that exist in the field of reporting, before warning about “highly sensitive data” and “potentially severe consequences” for non-compliance.
So, how does the industry get past this stage?
The glib answer is that it depends on the regulators. But risk managers may also have a part to play.
“There is great potential for thought leadership within industry. We need institutions to come forward with working models that demonstrate to regulators the clear potential of AI,” says Horompoly at ValidMind.
Chatbots, alert triage, scenario gen
Banks appear to be doing their bit by exploring a wide range of AI applications. This includes: using machine learning to validate balance sheet forecasts; risk control automation; detecting errors in data; code refactoring; and automatic report generation. Some institutions have started looking at how natural language processing can be used to extract environmental, social and governance factors from company reports, in order to inform credit risk models.
Examples of live AI from this year’s winning RTA pitches are similarly diverse. At the mature end of the spectrum are pattern-recognition algorithms that help sift through hordes of automated alerts – the goal is to detect worrying anomalies and reject benign ones, cutting down on the effort required of human analysts.
Others are trying something newer, but which has already been seen to work in other contexts – for example, a proprietary chatbot to help users navigate their way through a product. Another instance of this kind is an auto-summariser – an LLM-powered application that converts large quantities of data into reports that are easy for a human to quickly read and digest.
Elsewhere, there are references to AI-powered behavioural models, to NLP-driven data collection, and to AI-enabled scenario generation.
Scenario design feels like a good use for forms of generative AI, says Sasi Mudigonda, senior director for financial services analytical applications at Oracle Financial Services.
“Gen AI is being tried for developing more realistic adverse scenarios to help with stress testing so banks can prepare for unforeseen risks. This leverages the power of generative AI to connect different external and internal factors to devise ways that bank or insurer balance sheets could come under pressure. For banks, it might look at economic, geopolitical, or supply chain risks. For insurers, climate change might be a factor,” he says.
One common theme, though, is that banks and vendors alike are generally keeping the handbrake on, in an attempt to avoid alarming regulators – that means applications that whir along in the background, in a supporting role.
“In all of these cases, we see financial institutions are leaning into augmenting human capabilities rather than embracing full automation,” says Mudigonda.
A watching brief
To this point, bank watchdogs have been cautious about how they police AI development, preferring private conversations over public confrontation. In part, this may be an attempt to avoid constraining potentially beneficial innovation. It may also be a recognition that the technology is moving so rapidly that it could outpace the rule-making process.
In the US, for example, the country’s framework for model risk management, enshrined within a 13-year old supervisory document known as SR 11-7, has not been updated to reflect the latest developments in AI. The message given privately to banks is that the existing guidelines can and should be applied to AI algorithms in the same way as they are to classical models.
Politicians have been more active. The European Union’s AI Act, which was adopted by the European Parliament and Council earlier this year, applies to all AI applications – regardless of sector – and may affect decisions made by banks and other financial services firms. Among other things, the rules demand that rigorous governance practices be put in place to prevent AI systems discriminating against individuals and to ensure compliance with data protection laws.
Against this background of political scrutiny and regulatory caution, vendors have come up with their own, general rules of the road.
Banks need to make sure they have extended validation frameworks, so models maintain some kind of interpretability
Federico Crecchi, Prometeia
Vikas Agarwal, financial services risk and regulatory leader at PwC US, says the level of regulatory caution will be determined by the scope of any AI application – specifically, how close it is to directly impacting a firm’s customers.
“You can think of three different types of use case. One is in the back office, which will have minimal impact on customers. Two is where use cases start to affect customer decisions. Three is where AI systems are talking directly to the customers. Regulators will be able to get comfortable quite quickly with the first type of use case. It will take a bit longer for them to endorse the use of AI in box two or three,” says Agarwal.
Oracle’s Mudigonda puts it slightly differently, instead focusing on how sophisticated the underlying algorithms are – that is, how easy they are to understand and explain. More traditional AI models – using ‘white box’ machine learning techniques, such as regression, decision trees, Bayesian inference and so forth – will be least affected by new regulatory initiatives “because sufficient model risk management practices already exist for quantifying and managing risks of these models”.
On the other hand, he says, black box models – those based on deep-learning approaches, neural networks, boosting models, random forest models and transformers – will require additional model risk controls to be put in place.
Nonetheless, as the use of AI technology becomes more widespread, banks are likely to rely more and more on AI models to make decisions – and this will make some risks more pronounced. Mudigonda says this includes “model errors that produce inaccurate predictions which are hard to identify” and “model usage errors where a model is applied incorrectly or inappropriately”.
AI-specific model risk
Many jurisdictions already have specific rules surrounding model risk, and this landscape is evolving – a new framework took effect in the UK in May, for example. The UK document does not attempt to craft restrictions specific to artificial intelligence, but it does warn that “model risk increases with model complexity”, and gives the example of models that are “difficult to understand or explain in non-technical terms, or for which it is difficult to anticipate the model output given the input”.
Jos Gheerardyn, chief executive officer of Yields, a model risk management company, says that, as long as banks are adhering to these frameworks – even if they are not AI-specific – they should still be able to cope with the vagaries AI brings.
“While the AI lifecycle may be a bit different from the traditional model lifecycle, AI model risk management shouldn’t be all that different from what banks have in place at the moment. I would assume it will be a question of extending the frameworks banks already have in place, rather than replacing them completely,” says Gheerardyn.
Some of these extensions could be a significant stretch, however. In particular, where the application incorporates some kind of ongoing, self-directed learning, it is possible that the behaviour of a model could change over time. Some risk managers have suggested the model risk management framework for such applications would need some kind of near-real-time monitoring component.
This may sound daunting, but is consistent with the foundations of good AI governance, says Hopkins at Ripjar.
“The same principles underpinning sound machine learning systems have been in place for more than 30 years. This is the need to understand how well the models are performing, even if it is difficult to see how the model is working. Every time the model is changed, questions need to be asked. What difference is the change making? Is it introducing any biases?” he says.
Robust governance frameworks may have been important components of machine learning for decades, but Federico Crecchi, co-head of the data science practice of Prometeia, believes model validation is still a weak spot in many institutions.
“Banks have been building out their modelling functions around machine learning, but in order to make AI really operational they are going to have to sharpen their validation methodologies,” says Crecchi. “At some point in the future we may be able to make deep learning models more explainable and predictable, but we are not there yet. This is why banks need to make sure they have extended validation frameworks, so models maintain some kind of interpretability.”
Oracle’s Mudigonda says that, as various regulations take shape, there will be a need for evolving model risk management policies and practices.
“These efforts will possibly slow down the use of AI, especially within traditionally conservative risk management practices,” he says. “But by the same token, we see unambiguous momentum towards broader adoption of AI.”
Risk Technology Awards 2024: roll of honour
This year’s list is diverse, with Quantifi’s two wins the only example of a participant landing multiple awards. This contrasts with last year, when SAS bagged five wins, and four other firms were double-winners. Successful vendors this year run the gamut from venerable, globe-spanning tech titans to specialist start-ups – and established, mid-sized firms in-between.
In total, there are 19 awards this year, with entries invited for a further six categories. There were either too few entries in the missing categories, or no compelling entrant.
The winners
Bank ALM system of the year: Prometeia
Best vendor for systems support & implementation: Quantifi
Consultancy of the year, reg and compliance: PwC
Counterparty risk innovation of the year: Cumulus9
Credit data provider of the year: SOLVE
Credit risk innovation of the year: Dow Jones
Credit stress-testing product of the year: Quantifi
Cyber risk/security product of the year: Kovrr
Financial crime product of the year: Ripjar
Life and pensions ALM system of the year: Conning
Model validation service of the year: ValidMind
Op risk innovation of the year: Axoni
Op risk scenarios product of the year: Fusion Risk Management
Regulatory capital calculation product of the year: Oracle Financial Services
Regulatory reporting system of the year: Regnology
Risk dashboard software of the year: SS&C Technologies
Third-party risk product of the year: S&P Global
Trade surveillance product of the year: Eventus
Wholesale credit modelling software of the year: Moody’s
Methodology
Technology vendors were invited to pitch in 25 categories by answering a standard set of questions within a maximum word count. More than 130 submissions were received, resulting in over 64 shortlisted entries across the categories.
A panel of 10 industry experts and Risk.net editorial staff reviewed the shortlisted entries, with judges recusing themselves from categories or entries where they had a conflict of interest or no direct experience.
The judges individually scored and commented on the shortlisted entrants before meeting in June to review the scores and – after discussion – make final decisions on the winners.
In all, 19 awards were granted this year. Awards were not granted if a category had not attracted enough entrants or if the judging panel was not convinced by any of the pitches.
The judges
Sidhartha Dash, chief researcher, Chartis Research
Mayank Goel, compliance manager, MUFG
Christian Hasenclever, head of strategic asset and liability management, Norddeutsche Landesbank
Deborah Hrvatin, chief risk officer, CLS Group
Jenny Knott, audit committee chair, British Business Bank
Peter Quell, head of portfolio analytics for market and credit risk, DZ Bank
Andrew Sheen, independent consultant
Jeff Simmons, senior adviser, Alba Partners
Blake Evans Pritchard, Risk Technology Awards manager
Duncan Wood, editorial director, Risk.net
Only users who have a paid subscription or are part of a corporate subscription are able to print or copy content.
To access these options, along with all other subscription benefits, please contact info@risk.net or view our subscription options here: http://subscriptions.risk.net/subscribe
You are currently unable to print this content. Please contact info@risk.net to find out more.
You are currently unable to copy this content. Please contact info@risk.net to find out more.
Copyright Infopro Digital Limited. All rights reserved.
As outlined in our terms and conditions, https://www.infopro-digital.com/terms-and-conditions/subscriptions/ (point 2.4), printing is limited to a single copy.
If you would like to purchase additional rights please email info@risk.net
Copyright Infopro Digital Limited. All rights reserved.
You may share this content using our article tools. As outlined in our terms and conditions, https://www.infopro-digital.com/terms-and-conditions/subscriptions/ (clause 2.4), an Authorised User may only make one copy of the materials for their own personal use. You must also comply with the restrictions in clause 2.5.
If you would like to purchase additional rights please email info@risk.net
More on Risk management
Regionals built first-line defences pre-CrowdStrike
In-business risk teams vary in size and reporting lines, but outage fears are a constant
Op risk data: Santander in car crash of motor-finance fail
Also: Macquarie fined for fake metals trade flaws, Metro makes AML misses, and Invesco red-faced over greenwashing. Data by ORX News
Public enemy number one: the threat to information security
Nearly half of domestic and regional banks report risk appetite breaches amid heightened sense of insecurity
Credit risk transfer, with a derivatives twist
Dealers angle to revive market that enables them to offload counterparty exposures, freeing up capital
Op Risk Benchmarking 2024: the banks
As threats grow and regulators bore down, focus shifts to the first line
Fed stress-testing operational readiness of discount window
Experts say consultation on improved ops should be accompanied by focus on willingness to borrow
Millennium risk manager defends leverage in basis trade
“Gross notional measures don’t equate to market risk,” says Scott Rofey
Banks feel regulatory heat on op resilience
Op Risk Benchmarking: supervisors dial up reporting expectations and on-site inspections