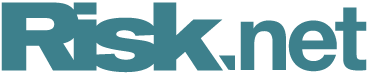
Rising star in quant finance: Silvana Pesenti
Risk Awards 2022: New approach allows portfolios to be optimised and aligned with benchmarks
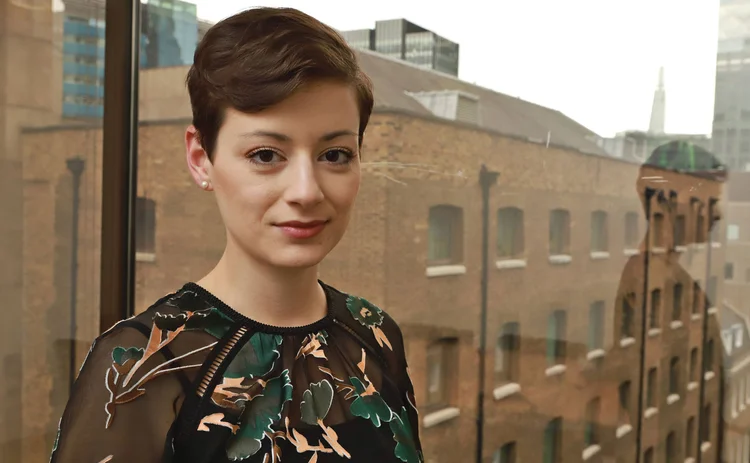
For active managers of many pensions or mutual funds, portfolio optimisation comes with the additional challenge of managing the closeness between a portfolio and the benchmark against which its performance will be measured.
In the vast literature examining the problem, researchers have shared a multitude of ideas. These include adapting Harry Markovitz’s mean-variance optimisation, measuring the tracking error between the benchmark and the portfolio, and building models in which investors have varying utility functions with respect to a benchmark.
In 2020, Silvana Pesenti, assistant professor of insurance risk management at the University of Toronto, decided to tackle the problem from a different perspective and redefine the closeness to a benchmark in terms of the Wasserstein distance. This tool, which allows managers to assess the difference between two distributions, has been used for a variety of financial applications, such as verifying the robustness of risk measures or identifying economic or financial regimes.
To develop her framework, Pesenti collaborated with a professor from the same department, Sebastian Jaimungal. Their paper, Portfolio Optimisation within a Wasserstein Ball, explains the technical aspects of their methods and provides a simulation technique to estimate the terminal wealth resulting from the optimal strategy.
Pesenti wins the Rising star in quant finance award for the originality and mathematical solidity of her work. This resulted in the highest score assigned by the selection committee, who reviewed the submissions of all this year’s candidates.
The proposed solution is designed to deliver a portfolio with a better risk-adjusted return than the benchmark while, at the same time satisfying a budget constraint, being distributionally close to the benchmark and obeying a given dependence structure with it.
The last two conditions are determined by the investor, who chooses how closely the portfolio strategy should follow the benchmark by setting the parameter of the Wasserstein ball and the dependence structure by setting a dependence via a copula.
That might sound complex, but Pesenti is adamant that although mastering the theory of this approach might take some effort, its application is straightforward and the full code is available on Github for everybody to try.
“There is an active academic literature investigating the tracking of benchmarks,” she says. “What is different in our proposal is the general class of risk measures we look at – which are distortion risk measures – and the deviation from the benchmark in the Wasserstein distance. We look at the distribution and the risks of the benchmark, and how it can be improved upon while not going too far away. It’s about inheriting the structure of the benchmark but improving upon it.”
Pesenti describes the approach as a “trade-off” between maintaining some benchmark characteristics, such as riskiness, and improving others, such as profits.
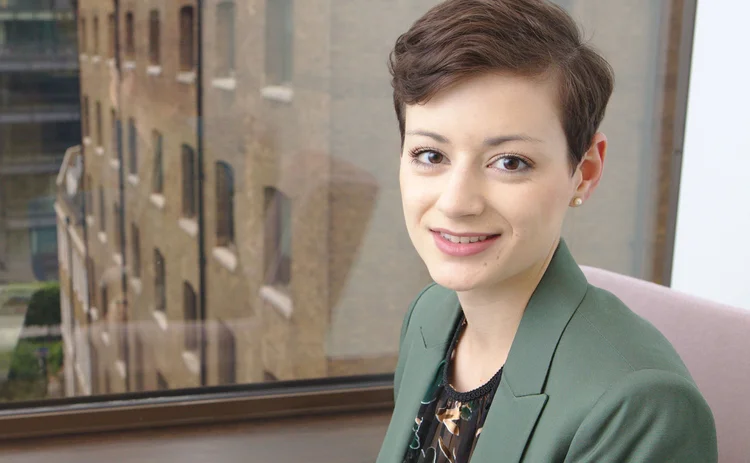
Jaimungal, who met Pesenti at a panel on reverse sensitivity testing in 2018, says the work combines his interest in stochastic control problems and Pesenti’s research into risk measures and risk management. He adds that institutional investors are the current target audience, and says pension and mutual funds are among those that could benefit. He hopes an approach to benchmark tracking that allows for greater control will prove both popular and useful.
“Investors will have a benchmark in mind, and will want to track it in some manner,” he explains. “But there are certain characteristics of the return that they’re not terribly happy with. So, they have some preferences, which can be represented in an economically precise manner as a risk measure.”
An investor tracking the S&P 500, for example, could opt for a co-monotonic dependence structure, which would produce lockstep movements in line with the benchmark while accounting for the investor’s chosen risk measures. Alternatively, Jaimungal says the investor could go for a “coin copular” dependence: the portfolio would move in lockstep with the benchmark in circumstances where the index was performing well; but if the benchmark fell, the portfolio would decouple and become independent.
“You may also want to use, for example, a Gumbel-type copula,” Jaimungal continues, “where you want to have strong dependence in tail events where the benchmark is doing exceedingly well but have less dependence in more typical or negative scenarios. It’s up to the investor to decide. The formulation is very general, and you can choose whatever copula you wish.”
Before beginning work on the paper, Pesenti had spent time investigating the Wasserstein metric and distortion risk measures for model uncertainty problems as part of her PhD in actuarial science and insurance at London’s Cass Business School (now Bayes). She says her future projects could involve a return to model uncertainty topics, in which she believes there are plenty of problems still to be tackled. She also hopes to take her portfolio optimisation work with Jaimungal further and perform case studies on real market data.
“People have found the idea of using the Wasserstein distance in a novel way – tying [a portfolio] to a benchmark – very interesting,” she says of the feedback she has had from her peers in finance and mathematics. “People found it relevant for practice. Our solution can be represented in a clean and neat way. It’s not a complex formula with many variables, and it doesn’t depend on a specific market model.”
Only users who have a paid subscription or are part of a corporate subscription are able to print or copy content.
To access these options, along with all other subscription benefits, please contact info@risk.net or view our subscription options here: http://subscriptions.risk.net/subscribe
You are currently unable to print this content. Please contact info@risk.net to find out more.
You are currently unable to copy this content. Please contact info@risk.net to find out more.
Copyright Infopro Digital Limited. All rights reserved.
As outlined in our terms and conditions, https://www.infopro-digital.com/terms-and-conditions/subscriptions/ (point 2.4), printing is limited to a single copy.
If you would like to purchase additional rights please email info@risk.net
Copyright Infopro Digital Limited. All rights reserved.
You may share this content using our article tools. As outlined in our terms and conditions, https://www.infopro-digital.com/terms-and-conditions/subscriptions/ (clause 2.4), an Authorised User may only make one copy of the materials for their own personal use. You must also comply with the restrictions in clause 2.5.
If you would like to purchase additional rights please email info@risk.net
More on Awards
Clearing house of the year: LCH
Risk Awards 2025: LCH outshines rivals in its commitment to innovation and co-operation with clearing members
Best use of machine learning/AI: CompatibL
CompatibL won Best use of machine learning/AI at the 2025 Risk Markets Technology Awards for its use of LLMs for automated trade entry, redefining speed and reliability in what-if analytics
Markets Technology Awards 2025 winners’ review
Vendors jockeying for position in this year’s MTAs, as banks and regulators take aim at counterparty blind spots
Equity derivatives house of the year: Bank of America
Risk Awards 2025: Bank gains plaudits – and profits – with enhanced product range, including new variants of short-vol structures and equity dispersion
Law firm of the year: Linklaters
Risk Awards 2025: Law firm’s work helped buttress markets for credit derivatives, clearing and digital assets
Derivatives house of the year: UBS
Risk Awards 2025: Mega-merger expected to add $1 billion to markets revenues, via 30 integration projects
Interest rate derivatives house of the year: JP Morgan
Risk Awards 2025: Steepener hedges and Spire novations helped clients navigate shifting rates regime
Currency derivatives house of the year: UBS
Risk Awards 2025: Access to wealth management client base helped Swiss bank to recycle volatility and provide accurate pricing for a range of FX structures