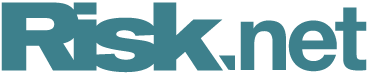
Best AI and machine learning innovation: GBG
Asia Risk Awards 2021
As digitalisation continues to accelerate, so will the volume of financial crime, and online financial crime in particular. Financial Crime 4.0, a term that GBG, a global digital identity expert and technology specialist in fraud and compliance management, location and identity data intelligence, coined to encapsulate emerging financial crime patterns, will continue to exacerbate.
Financial institutions would not only need to detect fraud, but also manage accuracy and efficiency in fraud detection. Fraud detection solutions that can achieve lower false-positive rates will be vital in helping banks minimise the number of investigations and analyses on fraud alerts.
While traditional rules-based detection systems are moderately effective, there are two main shortcomings in detection accuracy. The rules do not detect enough frauds, and they produce too many false positives.
In this area, machine learning can play a part in identifying and mapping out new patterns, tracking highly suspicious activities and known fraud better than a traditional rules-based engine.
Dev Dhiman, managing director for Asia-Pacific at GBG, says machine learning helps financial institutions enhance two fundamental processes – customer experience management and financial crime prevention.
“Reducing false-positive rates is not just about streamlining internal processes and costs, but in enabling legitimate customers to onboard and transact with success. At the same time, with Financial Crime 4.0 taking root and escalating, a rules-based system would eventually be inadequate to detect and keep up with emerging fraud typologies,” Dhiman says.
GBG Machine Learning uses algorithms to uncover inconsistencies in behaviours and activities, analyse them and flag anomalies compared to historical sets of personal data seen within the company or across the industry.
The feature engineering component within GBG Machine Learning creates the predictive analytics capability for the model. It allows the machine to continuously create accounts and customer profiles, the typical behaviour of that customer, and continuously compare the new behaviours to the typical behaviours. Anomalies are tweaked to help the model detect and isolate possible emerging and new fraud patterns.
For example, if an account is taken over using data from breaches, scams or phishing activity, GBG Machine Learning can isolate inconsistencies in the fraudster’s behaviour. If the fraudster started performing daily transfers just below the daily limit, which is a behaviour atypical of the original customer, GBG Machine Learning is able to pick out this anomaly and raise an alert.
GBG Machine Learning creates models that are sequenced to be activated with rules triggered versus no-rules triggered. By doing this, GBG is able to increase accuracy in identifying false positives by concentrating on the bigger pain point for this customer.
Unlike traditional rule-based systems that flag potential fraud because a series of rule conditions are not met, GBG’s goal for using machine learning is to find invisible and implicit correlations in data in real time and automate the detection of new possible fraud scenarios as quickly as possible, and to determine if the anomaly is due to an unusual or suspicious activity, instead of an unwitting human error.
Adam Emslie, head of analytics at GBG, says the most important factor about machine learning models is not that it is workable, but that they meet the efficiency and accuracy standards that customers and industry demands.
“Stemming from a single end-to-end solution, GBG’s Digital Risk Management and Intelligence platform passes data from origination and transactions directly into the machine learning models bypassing the need for data extraction, resulting in faster model training and deployment than what the industry typically offers,” he says.
Fraud and compliance objectives can be achieved through multiple workflows with the GBG Intelligence Center. Fraud analysts are enabled to prioritise and enhance accuracy in detection and prevention of larger fraud challenges.
As fraud instances continue to trend upwards across digital channels, GBG has incorporated device-level information as a feature, which adds significant uplift to the existing feature set.
This means GBG’s machine learning model ingests historic known fraud, data and behaviour, and also data received through GBG Intelligence Center, which includes IP, address, identity, email, social media, phone/SIM, device behaviour and any other third-party data from fraud consortium or third-party vendors.
Looking ahead, GBG plans to add feature profiling and bivariate analysis reporting for feature selection. With feature profiling, GBG analyses which fields are truly useful and can add value to fraud detection without cluttering the model.
Bivariate analysis will allow GBG to analyse the fields of value and discern which ones have a higher fraud rate.
GBG is also providing more information to explain the scores the models produce. It already returns global feature importance – the feature importance ranked the same for all customers and applications – to fraud reviewers. It aims to add local feature importance – specific variables/features that contribute to a particular customer application and fraud score, ranked by local context – to provide more insight as to why the model produced a particular score.
GBG is also providing insights into any potential bias against certain population segments because of the model’s output.
Only users who have a paid subscription or are part of a corporate subscription are able to print or copy content.
To access these options, along with all other subscription benefits, please contact info@risk.net or view our subscription options here: http://subscriptions.risk.net/subscribe
You are currently unable to print this content. Please contact info@risk.net to find out more.
You are currently unable to copy this content. Please contact info@risk.net to find out more.
Copyright Infopro Digital Limited. All rights reserved.
As outlined in our terms and conditions, https://www.infopro-digital.com/terms-and-conditions/subscriptions/ (point 2.4), printing is limited to a single copy.
If you would like to purchase additional rights please email info@risk.net
Copyright Infopro Digital Limited. All rights reserved.
You may share this content using our article tools. As outlined in our terms and conditions, https://www.infopro-digital.com/terms-and-conditions/subscriptions/ (clause 2.4), an Authorised User may only make one copy of the materials for their own personal use. You must also comply with the restrictions in clause 2.5.
If you would like to purchase additional rights please email info@risk.net
More on Awards
Joining the dots: banks leverage tech advancements for the future of regulatory reporting
The continued evolution of regulatory frameworks is creating mounting challenges for capital markets firms in achieving comprehensive and cost-effectiveawa compliance reporting. Regnology discusses how firms are starting to use a synthesis of emerging…
Markets Technology Awards 2024 winners' review
Vendors spy opportunity in demystifying and democratising – opening up markets and methods to new users
Derivatives house of the year: JP Morgan
Risk Awards 2024: Response to regional banking crisis went far beyond First Republic
Risk Awards 2024: The winners
JP Morgan wins derivatives house, lifetime award for El Karoui, Barclays wins rates
Best product for capital markets: Murex
Asia Risk Awards 2023
Technology vendor of the year: Murex
Asia Risk Awards 2023
Best structured products support system: Murex
Asia Risk Awards 2023
Energy Risk Asia Awards 2023: the winners
Winning firms demonstrate resiliency and robust risk management amid testing times