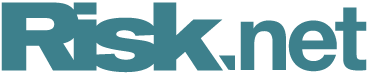
Technology innovation of the year: Scotiabank
Risk Awards 2021: New risk engine can run nearly a billion XVA calculations per second
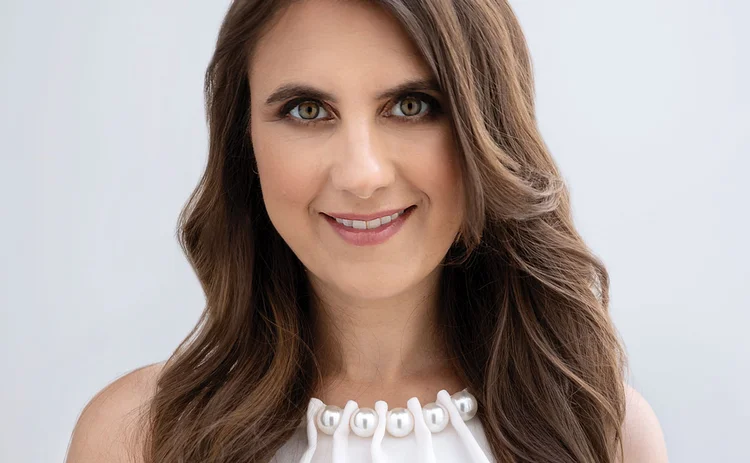
Derivatives clients of Scotiabank may have noticed the bank’s pricing has been tighter of late. They may not know this is due to its new risk engine, which was built to calculate valuation adjustments (XVAs) faster and more accurately.
XVAs reflect the credit, funding and capital costs associated with trading over-the-counter derivatives. Dealers typically incorporate these adjustments into the price of a new trade. But calculating XVAs is one of the largest computational challenges banks face on a daily basis.
Scotiabank’s risk engine combines a mathematical technique known as adjoint algorithmic differentiation, or AAD, with deep neural networks, a branch of artificial intelligence – a mashup the bank claims is a first.
The system is powered by graphics processing units (GPUs) running in the cloud – another first.
The result is a tool that allows traders and risk managers to better understand exposure across asset classes, including rates, credit, foreign exchange and commodities. This insight proved invaluable for Scotiabank during the coronavirus chaos earlier in 2020, particularly as liquidity dried up in some markets.
When markets started to dislocate at the end of March and into April, the risk engine enabled the bank to run multiple scenario analyses to calculate, on-the fly, the impact to its book.
“We tested different flavours of correlation and used these to guide our hedging strategy as we saw which scenario unfolded in the markets on any given day. The migration to this new platform has given us greater visibility into our risk,” says Karin Bergeron, head of XVA trading at Scotiabank.

The risk engine gave the bank a better steer for how to respond to market gyrations when volatility was at its most unpredictable, she adds: “We ran scenario analysis on large rapid moves in credit, interest rates and FX. Although it was impossible to predict the exact scenario, this warned us ahead of time that if we saw a 100 basis point move in credit, we would need to put on large hedges in the rates and FX markets.”
The bank’s fears of outsized swings in credit prices were realised. The CDX index of investment grade credit default swaps blew out from mid-40 basis points in February to around 140bp in March before retracing to 84bp in mid-April.
A surge in credit risk during that period, coupled with a jump in funding costs, contributed to billions in losses from XVAs for major investment banks. JP Morgan, for example, took a $951 million XVA hit in the three months to March 31, although it also saw a partial reversal the next quarter, booking a $510 million gain from XVAs.
Scotiabank did not report an XVA charge on its balance sheet during the quarter ending April 30, although it took a one-off C$116 million ($88 million) charge the previous quarter as a result of a methodology change.
The bank did record a C$150 million rise in the amount of capital it set aside to cover credit valuation adjustments in the three months ending April 30, 2020, but this figure fell by roughly the same amount the following quarter.
John Gjata, global head of fixed income solutions at Scotiabank, says the quicker pricing produced by the engine’s souped-up computation is having a big impact on the bank’s business with large institutional clients, including bank treasuries. Trade flows have increased, he says, as it is able to better exploit fleeting dislocations in the market, especially in highly liquid G7 currencies.
Gjata adds that the system also shows its advantages in calculating risk on trades in longer tenors and in more esoteric currencies.
Scotiabank reported a 33% year-on-year rise in revenues for its wholesale banking business to end-October 2020. These results included a 38% increase in capital markets revenues and an 87% increase in rates and credit trading revenues.
Engine’s combined tech
The standard way of calculating XVAs is to run numerous Monte Carlo simulations of a derivatives portfolio under different scenarios. For this, banks generally rely on vast computing grids of central processing units, or CPUs.
In 2016, Scotiabank began experimenting with AAD, a whizzier mathematical trick for calculating sensitivities at high speed. Other banks had adopted the technique a year or two before, but Scotiabank started a project to combine AAD with GPUs, which are said to be around 10 to 50 times faster than their CPU cousins. This pairing was described in 2015 by vendor Numerical Algorithms Group as “the holy grail”.
The bank also opted to deploy GPUs on the cloud, which it claims is a more efficient way of operating. Cloud hosting means the bank does away with owning a large amount of expensive hardware that is difficult to manage and under-utilised during parts of the week.
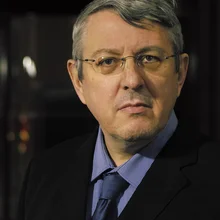
“We can go out and buy a GPU machine but it will be obsolete in a year’s time. We’ve already gone through one complete machine refresh on this, to keep us at the leading edge of GPU performance,” says Tom Pickering, technology fellow at Scotiabank. Using cloud GPUs enables the bank to “turn fixed costs to floating costs”.
The cloud GPU platform, hosted on Microsoft Azure, was first deployed in March 2019. The bank says it allows for more detailed scenario modelling, assessing more than 10 times the number of previous scenarios.
In 2020, Scotiabank was finally able to switch on the AAD function of its cloud-hosted GPU platform, having used a less efficient but easier to implement mode until then. The bank teamed up with Numerical Algorithms Group to develop the AAD algorithm library.
The result is a tool that can evaluate large numbers of first and second order sensitivities in around 30 minutes during end-of-day batch processing. On a normal day the platform is available on demand for XVA pricing, which it takes in the order of 20 seconds to calculate. And, during periods of market stress, the system can be scaled up to provide intraday risk and scenario calculations. For portfolios with 100,000 trades, the XVA engine can execute 915 million valuations per second.
Another landmark was to add artificial intelligence-based technology to the engine, which Scotiabank did in 2020. In partnership with tech vendor Riskfuel, the bank has trained deep neural networks (DNNs) as a valuation methodology within the framework. Riskfuel takes Scotiabank’s models then trains its DNNs to replicate them.
The DNNs replace a hand-coding model and are built on a common infrastructure that uses linear algebra. This limits the need to code lots of models, as once the DNN infrastructure is built it does not need to be built again. After training, the DNNs are co-located with the Monte Carlo simulation on the Azure GPU cards. They currently cover a range of moderately exotic payoffs including Asian options and barrier options.
Andrew Green, lead XVA quant at Scotiabank, and Riskfuel CEO Ryan Ferguson described how deep learning could be used to value derivatives in a 2018 paper called Deeply Learning Derivatives.

Green says the method used is similar to the Longstaff-Schwartz approach, which allows a portfolio to be compressed to a single regression function so that it need not be revalued at each time point.
Put into practice, the platform’s web interface allows the bank’s front office to run XVA analysis with real-time data on either existing active trades or ‘what-if’ trades with potential clients. The system is available for calculation 24/7 globally.
The atypical market conditions experienced in March and April caused problems for the trading desk, which the risk engine helped address, Gjata says: “Market movements create sensitivities and those sensitivities create axes, for example in a cross-currency basis for a pair that we want to trade. That pair could trade on average $50 million a day, but we have an axe for four times that, which is difficult to get executed in the market, especially in a period where liquidity has dropped to zero.”
So, the bank would seek clients who need to issue debt in a foreign currency and swap it back to Canadian dollars. The risk engine would help the desk price 50 to 100 new issue hedge permutations of the same trade to find the client that would benefit most from the transaction.
“You run all the different scenarios and then, voilà, you find one issuer for whom the idea suits that day,” Gjata says.
The new engine has borne fruit particularly with the flow segment of the business, which requires sharp and instantaneous pricing.
“So now you’ve got a happy issuer, because they’re able to raise debt, you’ve got a happy investor that was able to buy debt cheaply in their local currency. And Scotiabank is able to get all its risk done at a decent market level,” Gjata adds.
Only users who have a paid subscription or are part of a corporate subscription are able to print or copy content.
To access these options, along with all other subscription benefits, please contact info@risk.net or view our subscription options here: http://subscriptions.risk.net/subscribe
You are currently unable to print this content. Please contact info@risk.net to find out more.
You are currently unable to copy this content. Please contact info@risk.net to find out more.
Copyright Infopro Digital Limited. All rights reserved.
As outlined in our terms and conditions, https://www.infopro-digital.com/terms-and-conditions/subscriptions/ (point 2.4), printing is limited to a single copy.
If you would like to purchase additional rights please email info@risk.net
Copyright Infopro Digital Limited. All rights reserved.
You may share this content using our article tools. As outlined in our terms and conditions, https://www.infopro-digital.com/terms-and-conditions/subscriptions/ (clause 2.4), an Authorised User may only make one copy of the materials for their own personal use. You must also comply with the restrictions in clause 2.5.
If you would like to purchase additional rights please email info@risk.net
More on Awards
Clearing house of the year: LCH
Risk Awards 2025: LCH outshines rivals in its commitment to innovation and co-operation with clearing members
Best use of machine learning/AI: CompatibL
CompatibL won Best use of machine learning/AI at the 2025 Risk Markets Technology Awards for its use of LLMs for automated trade entry, redefining speed and reliability in what-if analytics
Markets Technology Awards 2025 winners’ review
Vendors jockeying for position in this year’s MTAs, as banks and regulators take aim at counterparty blind spots
Equity derivatives house of the year: Bank of America
Risk Awards 2025: Bank gains plaudits – and profits – with enhanced product range, including new variants of short-vol structures and equity dispersion
Law firm of the year: Linklaters
Risk Awards 2025: Law firm’s work helped buttress markets for credit derivatives, clearing and digital assets
Derivatives house of the year: UBS
Risk Awards 2025: Mega-merger expected to add $1 billion to markets revenues, via 30 integration projects
Interest rate derivatives house of the year: JP Morgan
Risk Awards 2025: Steepener hedges and Spire novations helped clients navigate shifting rates regime
Currency derivatives house of the year: UBS
Risk Awards 2025: Access to wealth management client base helped Swiss bank to recycle volatility and provide accurate pricing for a range of FX structures