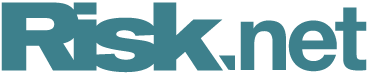
Data mining, machine learning and rival discount rates
The week on Risk.net, June 30-July 6, 2018

Quants are building statistical toolkits to avoid the pitfalls of data mining
OK, computer: hurdles remain for machine learning in credit risk
Concerns over cost, applicability and oversight give pause to banks’ use of ML techniques in credit risk
Clearers diverge on SOFR swaps discounting
CME switches to new rate for clearing; rival LCH stays with Fed funds
COMMENTARY: Torturing the data
Eating too much cheese causes Americans to strangle themselves with their own bedsheets. Buying margarine makes the people of Maine leave their spouses. Every new Nicolas Cage film brings in its wake an epidemic of drowning in swimming pools. It must be true – the datasets correlate almost perfectly.
These and other spurious correlations (collected by the author and consultant Tyler Vigen) point to one of the biggest problems of big data – with a large enough universe of data to pick from, you can produce a convincing statistical story to explain pretty much any series of points. But, of course, that isn’t the same as a reliable story. Banning margarine would not lead to a sudden outbreak of marital harmony among the pine trees and lobsters. It’s unlikely that even The Wicker Man caused any Americans to fling themselves despairing into the nearest body of water.
Big-data partisans in the financial sector are coming to a very similar conclusion. Too many of those promising big data and machine learning projects in quantitative finance have repaid their backers only with fool’s gold; they have dredged through enormous data sets in search of a profitable discovery and found only a spurious correlation with no predictive power. Many more have only rediscovered an already-known factor.
Some of the most heated arguments centre around the role of human judgement. Many quants argue data mining without forming a hypothesis first is unscientific – that way lie swimming pools, Nicolas Cage and other irrational conclusions. And those cautious about the use of these technologies in credit risk modelling point out that regulators will be sceptical of models developed without human intervention. Their scepticism is justified – blind trust in a black box model is a very serious risk.
But there are arguments on the other side too: humans are biased and blinkered reasoners, and basing models on human intuition risks enshrining human bias in code, or missing unorthodox profit opportunities, argue machine learning supporters.
A more productive area for quants would be encouraging a more sophisticated understanding of probability and statistical robustness. Many academics are already arguing in this direction – including those pushing for more widespread use of Bayesian statistics. It will mean abandoning many expensively developed and dearly held beliefs; but that’s what science is all about.
STAT OF THE WEEK
Figures published by the Fed as part of this year’s Comprehensive Capital Analysis and Review show the 35 participating banks would suffer total losses of $578 billion in a severe recession – $98 billion more than last year’s result.
QUOTE OF THE WEEK
Boards have gone from turning up once a quarter for a prawn sandwich to being down in the weeds of what you do – Stephen Creese, Citi
Further reading
Only users who have a paid subscription or are part of a corporate subscription are able to print or copy content.
To access these options, along with all other subscription benefits, please contact info@risk.net or view our subscription options here: http://subscriptions.risk.net/subscribe
You are currently unable to print this content. Please contact info@risk.net to find out more.
You are currently unable to copy this content. Please contact info@risk.net to find out more.
Copyright Infopro Digital Limited. All rights reserved.
As outlined in our terms and conditions, https://www.infopro-digital.com/terms-and-conditions/subscriptions/ (point 2.4), printing is limited to a single copy.
If you would like to purchase additional rights please email info@risk.net
Copyright Infopro Digital Limited. All rights reserved.
You may share this content using our article tools. As outlined in our terms and conditions, https://www.infopro-digital.com/terms-and-conditions/subscriptions/ (clause 2.4), an Authorised User may only make one copy of the materials for their own personal use. You must also comply with the restrictions in clause 2.5.
If you would like to purchase additional rights please email info@risk.net
More on 7 days in 60 seconds
Bank capital, margining and the return of FX
The week on Risk.net, December 12–18
Hedge fund losses, CLS and a capital floor
The week on Risk.net, December 5–11
Capital buffers, contingent hedges and USD Libor
The week on Risk.net, November 28–December 4
SA-CCR, SOFR lending and model approval
The week on Risk.net, November 21-27, 2020
Fallbacks, Libor and the cultural risks of lockdown
The week on Risk.net, November 14-20, 2020
Climate risk, fixing Libor and tough times for US G-Sibs
The week on Risk.net, November 7-13, 2020
FVA pain, ethical hedging and a degraded copy of Trace
The week on Risk.net, October 31–November 6, 2020
Basis traders, prime brokers and election risk
The week on Risk.net, October 24-30, 2020