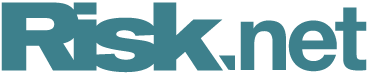
Mifid, machine learning and swaps compression
The week on Risk.net, March 10–16, 2017
MIFID alarms the rest of the world
MACHINE LEARNING attracts quant and sales attention
SWAPS COMPRESSION race heats up
COMMENTARY: The problems with machine learning
The banking industry has not always been the best at adopting new technology. For many years, it was the last redoubt for such items of industrial archaeology as fax machines and telex terminals; even today, the internal networks of many major banks are tangled nightmares of obsolete software and incompatible standards. The reasons for this vary – from regulation and staff turnover to a widespread belief in secrecy and proprietary software over open standards – but in one area, progress is speeding up.
Machine learning, after many false starts under various names, is now flavour of the year in financial technology, promising better regulation, smarter risk management and sharper sales tactics, by providing financial institutions with a tool that can process the immense amounts of information being collected under 'big data' schemes. Automated systems that were intended to detect suspicious transactions and prevent fraud or money laundering can also be used to profile retail customers for marketing purposes. Now derivatives sales teams are using the same approaches to guide their own sales and client relations efforts – either backing up human salespeople, or replacing them altogether.
But risk managers need to be more cautious, delegates at the Quant Europe conference heard this week: training machine-learning software on historical records risks judging the future by the standards of the past, which might make sense in medicine but not in an evolving field like finance, Riccardo Rebonato warned. But, as other delegates noted, in many areas, the volumes of data involved may leave banks no choice but to rely on machine-learning approaches to an ever greater degree. “[Machine learning] is a way for us to find a solution without writing equations, without building the model – we just let our data tell us what the optimal solution is,” one commented.
The crucial consequence of this is that modellers and risk managers must be able to defend the results. If the process is obscured within a black-box machine-learning process such as a neural net, the output must still be comprehensible – to regulators, and to the senior managers who will rely on it for decisions on new products, risk appetite and business strategy generally.
STAT OF THE WEEK
QUOTE OF THE WEEK
“I don’t know how long it takes for CVA to blow up in our faces in the event of a big bank default, but frankly the numbers we get out of this exercise are based on wishful thinking; I’m not sure what they really mean” – Rama Cont, professor of mathematics and chair of mathematical finance at Imperial College London.
Further reading
Only users who have a paid subscription or are part of a corporate subscription are able to print or copy content.
To access these options, along with all other subscription benefits, please contact info@risk.net or view our subscription options here: http://subscriptions.risk.net/subscribe
You are currently unable to print this content. Please contact info@risk.net to find out more.
You are currently unable to copy this content. Please contact info@risk.net to find out more.
Copyright Infopro Digital Limited. All rights reserved.
As outlined in our terms and conditions, https://www.infopro-digital.com/terms-and-conditions/subscriptions/ (point 2.4), printing is limited to a single copy.
If you would like to purchase additional rights please email info@risk.net
Copyright Infopro Digital Limited. All rights reserved.
You may share this content using our article tools. As outlined in our terms and conditions, https://www.infopro-digital.com/terms-and-conditions/subscriptions/ (clause 2.4), an Authorised User may only make one copy of the materials for their own personal use. You must also comply with the restrictions in clause 2.5.
If you would like to purchase additional rights please email info@risk.net
More on 7 days in 60 seconds
Bank capital, margining and the return of FX
The week on Risk.net, December 12–18
Hedge fund losses, CLS and a capital floor
The week on Risk.net, December 5–11
Capital buffers, contingent hedges and USD Libor
The week on Risk.net, November 28–December 4
SA-CCR, SOFR lending and model approval
The week on Risk.net, November 21-27, 2020
Fallbacks, Libor and the cultural risks of lockdown
The week on Risk.net, November 14-20, 2020
Climate risk, fixing Libor and tough times for US G-Sibs
The week on Risk.net, November 7-13, 2020
FVA pain, ethical hedging and a degraded copy of Trace
The week on Risk.net, October 31–November 6, 2020
Basis traders, prime brokers and election risk
The week on Risk.net, October 24-30, 2020