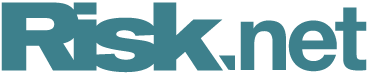
Quant research team of the year: Deutsche Bank
Risk Awards 2019: Deutsche’s quants dig for alpha

Deutsche Bank’s presentations for its newest investment tool – Alpha-Dig – include a Venn diagram showing different types of company information that move stock prices. It looks rather like a drawing from a cell biology textbook.
At one end, shaded blobs represent assets, liabilities, revenues, costs and so on – grouped in a zone titled “hard information”. Overlapping them is a much larger “soft information” area, encircling colourful globules labelled “supply chain”, “regulatory risk” and “reputational risk”.
Deutsche built and launched Alpha-Dig to help its clients put those blobs of intangible value under the microscope. Launched in April, a thousand individuals are trialling the product already.
“It’s the most sophisticated use of artificial intelligence to extract meaning from documents that I’ve seen across the Street,” says one portfolio manager.
Alpha-Dig is perhaps the brightest but not the only highlight of Deutsche’s year. The bank’s quant research team constructed a unique database to understand how exchange-traded fund (ETF) flows affect stock pricing. Deutsche also this year published timely studies on defensive and short volatility strategies that have been well received by clients.
“They produce research I can act on,” says the portfolio manager. Deutsche’s work on ETF flows was the “first concrete empirically based evidence” on how flows are affecting prices – a question that’s been bugging investors for years, he adds.
The bank’s cross-asset quant team, in particular, is “one of the best on the street”, says a second client. He describes Deutsche’s analysts as “pioneers”.
Projects such as Alpha-Dig reflect a broader push by the bank to respond to growing interest in the use of data among investors of all creeds. Increasingly, the quant team works on projects to support the bank’s fundamental analysts, for example.
“We want to build on our strength in quantitative research across asset classes, but also to grow more broadly, particularly through leveraging the power of artificial intelligence and data science in other areas – in company research, in macroeconomic research, in fixed income research,” says Spyros Mesomeris, who heads quant and quantitative investment solutions research.
The bank has built a data science team, DB-Dig, comprising 12 staff so far, including experts in satellite imagery, in nowcasting, specialists in conducting market surveys, even quantitative psychologists – experts in modelling human behaviour.
The rest of the quantitative research and quant investment solutions team is growing too – this at a time when Deutsche more widely is making well-publicised cuts.
Deep reading
Other banks and buy-siders have used algorithms to derive sentiment scores from text data. But where other tools typically do so by counting terms in documents and classifying them as positive or negative, Alpha-Dig uses machine learning techniques including some of the same employed in driverless cars to learn how words – when they appear together – flag problems.
The tool covers 5,000 companies, using the branch of machine learning known as natural language processing to scan and “understand” stories in the financial press, patent filings, company announcements, court and regulatory filings.
Alpha-Dig renders this information into a numerical sentiment score but, critically, also enables users to see how the score was reached.
Mesomeris says it gives clients more nuanced information than just a binary positive or negative rating. “It allows you to look into similar case studies, see how close they are to the event you are interested in and use a score in your own models based on that similarity.”
The tool also scores companies against United Nations sustainable development goals – a set of targets for improving global health, education and sustainability that asset allocators are beginning to use in investment choices; it is also able to quantify a company’s exposure to economic “themes”, such as the growing use of artificial intelligence.
Andrew Moniz, Deutsche’s chief data scientist, likens using Alpha-Dig to a new form of “mosaic investing”, the name used in the past to describe collecting information about companies from multiple sources.
We want to build on our strength in quantitative research across asset classes, but also to grow more broadly, particularly through leveraging the power of artificial intelligence and data science in other areas
Spyros Mesomeris, Deutsche Bank
Today, the information sources have simply become more numerous and harder to comb through, the bank says. In its own work, Deutsche found details of litigation and regulatory risk are typically buried three-quarters of the way through company risk factor disclosures.
Already, Alpha-Dig has unearthed some surprises.
If a materials company experiences supply chain disruption, to give one example, Alpha-Dig says its stock will drop 4% on average. But the losses unfold over the next 40 trading days – much longer than investors might think. Generally it takes three months before the share price will start recovering, the data says.
For a hint of how much alpha is on offer, Deutsche says it can improve the Sharpe ratio of its own existing value factor strategies by 15%, by using the tool to identify and avoid companies whose stock is cheap for good reason.
The year has not been just about clever new machine learning tools, though.
In equity quant research, Deutsche built a proprietary database of ETF composition going back to 2005 and covering more than 97% of Russell 3000 stocks, in order to analyse the impact of the $5 trillion sector on market moves.
The research threw up some counterintuitive findings, around which the bank has been able to construct trading strategies.
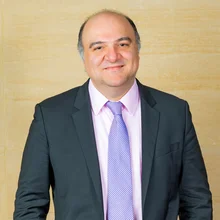
A stock being “promoted” from the Russell 2000 (small cap) index to the Russell 1000 (large cap) index, for example, sees ETF flows turn negative, causing its stock price to fall. Conversely, if the smallest stock in the Russell 1000 gets demoted and becomes a large stock in the Russell 2000, the ETF stake in its stock might increase 100 times or more.
The effect occurs because fewer assets are invested in the large cap index, and because stocks that attract a high weighting at the top of the small cap index get a low weighting when they move to the bottom of the large caps.
On the other hand, stocks moving between S&P indexes, for example, experience less extreme flows because of the relative volume of assets referencing the different indexes.
Deutsche says a dollar invested in companies with the most negative ETF flow in 2006 would have grown to $3.48 by the end of 2017 compared with $1.40 for those with the highest flow. Backtests show the strategy achieved a Sharpe of 1.67 since 2009.
The bank’s cross-asset quant team, meanwhile, put out in February a detailed study of the best way to combine defensive strategies – timely advice for clients in a month during which many such strategies were suffering badly.
Caio Natividade, Deutsche’s head of cross-asset quantitative research, says banks including his own have typically focused on developing return streams that are uncorrelated with wider markets. But clients increasingly are asking for products that are “anti-correlated” to public markets, he says.
He attributes the change in thinking to worries about fixed income portfolios as the era of quantitative easing comes to an end, as well as the recent failure of several common defensive strategies to protect against stock market falls. “In February, Treasuries didn’t work. Trend following didn’t work,” he says.
Awareness among asset allocators of the importance of getting through such episodes unscathed has also grown.
Deutsche traced the impact of the best and worst 5% of months on investors’ compound annual growth rates using proxy data for funds, finding the worst months had the biggest effect, followed by the best months in second place.
“The other 90% of months don’t really matter that much,” Natividade says. “It’s how you performed in the worst and best months that matters.”
The team looked at how to construct portfolios of 11 common defensive strategies so as to achieve the best combination of diversification and capital protection.
Natividade describes the task as a portfolio optimisation problem. Ultimately, the best mix of strategies will depend on an investor’s wider choices. Broadly, though, Deutsche’s work shows that combining both diversifying and insurance-type strategies in a drawdown protection overlay is likely to lead to better long-term growth – with the diversifying strategies reducing the performance drag of the insurance.
At the same time, investors should be willing to include more volatile insurance-type strategies in the mix, as doing so can enhance the capital efficiency of the overall strategy, the team says.
Deutsche also examined ways to enhance short-volatility strategies, saying buy-siders should be copying alternative delta hedging approaches commonly used by market-makers to hedge gamma exposure when selling options.
In simple terms, the idea is to underhedge selectively to allow for intraday momentum in futures markets, which typically leads to futures trading rich into the close.
“There has been a disconnect between those that make markets in options and options users,” Natividade says. “Alternative delta hedging is the bread and butter of profit generation for bank options desks. They do this all the time. Risk premia investors on the other hand tend not to think like that.”
Only users who have a paid subscription or are part of a corporate subscription are able to print or copy content.
To access these options, along with all other subscription benefits, please contact info@risk.net or view our subscription options here: http://subscriptions.risk.net/subscribe
You are currently unable to print this content. Please contact info@risk.net to find out more.
You are currently unable to copy this content. Please contact info@risk.net to find out more.
Copyright Infopro Digital Limited. All rights reserved.
As outlined in our terms and conditions, https://www.infopro-digital.com/terms-and-conditions/subscriptions/ (point 2.4), printing is limited to a single copy.
If you would like to purchase additional rights please email info@risk.net
Copyright Infopro Digital Limited. All rights reserved.
You may share this content using our article tools. As outlined in our terms and conditions, https://www.infopro-digital.com/terms-and-conditions/subscriptions/ (clause 2.4), an Authorised User may only make one copy of the materials for their own personal use. You must also comply with the restrictions in clause 2.5.
If you would like to purchase additional rights please email info@risk.net
More on Awards
Trading systems: structured products/cross-asset – Murex
Murex won the Trading systems: structured products/cross-asset award at the 2025 Risk Markets Technology Awards for its MX.3 platform, praised for its flexibility and advanced analytics
Best vendor for system support and implementation: Murex
Murex has won the Best vendor for system support and implementation at the Risk Markets Technology Awards, recognised for its innovative MX.3 platform, exceptional client support and seamless implementation services
FRTB-IMA product of the year: Murex
Murex wins FRTB-IMA product of the year for its advanced, scalable MX.3 platform enabling seamless regulatory compliance
Pricing and analytics: equities – Finastra
Finastra’s Sophis platform wins the Risk Markets Technology Award for Pricing and analytics in equities, recognised for its robust capabilities in equities and derivatives trading
Best execution product of the year: Tradefeedr
Tradefeedr won Best execution product of the year for its API platform, which standardises and streamlines FX trading data, enabling better performance analysis and collaboration across financial institutions
Collateral management and optimisation product of the year: LSEG Post Trade
LSEG Post Trade wins Collateral management and optimisation product of the year for interconnected services that help mitigate counterparty risk and optimise capital usage
Clearing house of the year: LCH
Risk Awards 2025: LCH outshines rivals in its commitment to innovation and co-operation with clearing members
Driving innovation in risk management and technology
ActiveViam secured three major wins at the Risk Markets Technology Awards 2025 through its commitment to innovation in risk management and technology