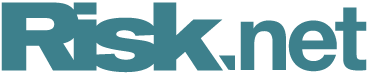
Quant hedge fund of the year: Man AHL
Risk Awards 2018: New roles for machine learning, frontier markets and OTC data give fund an edge
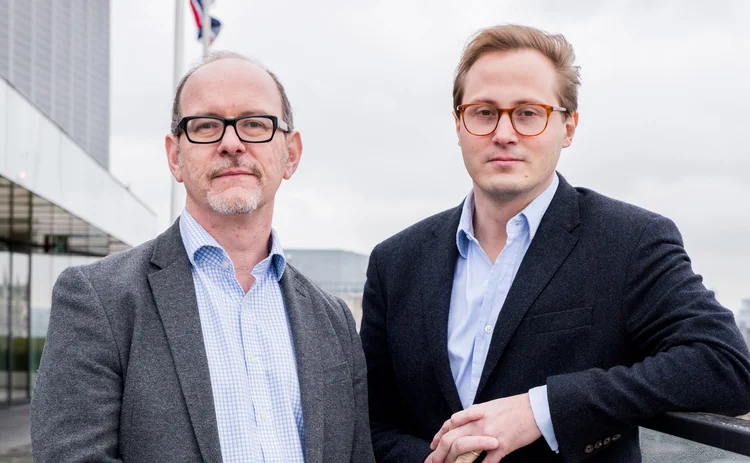
A floor-to-ceiling screen in Man AHL’s London office shows the stream of trades generated by the firm’s computers and how they are being executed – by internal execution algorithms, external dealer algorithms or the firm’s trading desk.
Making the call on which route each trade takes is a machine learning algorithm launched by AHL this year (see box: A problem solved). The better the execution achieved by each route, the more trades get sent that way. Naturally, the human traders like to come out on top when they can.
This setup says much about AHL’s ability to apply machine learning in less obvious ways than rivals. More broadly, it also shows the firm’s zeal to innovate in all corners of the market. “The order router isn’t classic alpha. But it potentially has as much impact for our clients as any of our trading systems,” says Matthew Sargaison, co-chief executive.
At a time when other trend-following funds have struggled, AHL – the quant arm of Man Group – appears to be benefiting from this commitment to innovation. Its trend-following funds are performing strongly, and assets under management were up $2.5 billion for the 12 months to the end of September.
Sargaison says it is too early to quantify how much the order-routing algorithm is saving, but claims the early signs are promising. The algorithm directs trading based on past experience and uses machine learning to rebalance as it goes, recalculating the probability of the outcomes for each execution route as it gathers information.
The task is perfect for machine learning because the algorithm must adapt to a changing live environment, says Sargaison: “As soon as you place an order, the order book changes. Having algorithms learn about the way their execution impacts other people’s behaviour is something we can’t do from simple back testing.”
Elsewhere this year, the firm added momentum strategies using machine learning models – already deployed in its multi-strategy funds – to its main trend funds.
Though the output from these models is highly correlated to existing momentum signals, they are better able to distinguish between market dips that present a buying opportunity versus those that presage a further decline.
The non-linear interactions when you’ve got five or six different types of signals become much harder to explain. That’s where you start relying on machine learning
Matthew Sargaison, Man AHL
It comes down to recognising how multiple signals interrelate. A 2% market fall in the middle of a six-month bull run might be a buying opportunity, Sargaison explains. But if the market has been noisy and flat for six months, it could be the beginning of a significant correction.
With two signals, that’s simple to model. “The non-linear interactions when you’ve got five or six different types of signals become much harder to explain,” he says. “That’s where you start relying on machine learning.”
Sargaison says the keys to making machine learning work are “hiring people with hands-on experience, making your smartest staff really think hard about problems, and being prepared to fail”.
The first of these is tricky, because competition is fierce, he says. But AHL benefits from close relationships with expert practitioners through its endowment of a post at Oxford University and support of the Oxford-Man Institute of Quantitative Finance.
That initiative has helped AHL identify academic studies in different fields that can be applied in investing. One example is a machine learning technique used by astronomers to identify supernovae, which AHL used to classify stock analysts on the forecasting power of their predictions.
The second and third come down to a culture of encouraging ideas but setting a high bar for success. Sargaison says he is a “huge believer” in bottom-up research: “Every year, the best single new alpha idea comes from the least likely source.”
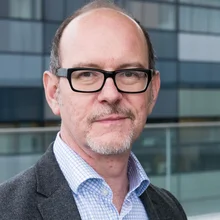
We don’t own a satellite and we aren’t collecting our own satellite images. What we are doing is interacting with the market, and we’ve been meticulous in recording every point at which we’ve done that
Matthew Sargaison, Man AHL
At the same time, fewer than one in 10 ideas make it past test trading. “Recently, we’ve had a higher internal reject rate for research than at any time in our history, and I feel good about that,” he says.
Also important is AHL’s meticulous hoarding of data, especially from over-the-counter markets.
Here the firm benefits from its decision 12 years ago to pursue trend following in markets beyond the managed futures domain common to most commodity trading advisers (CTAs). It was a bold choice, requiring new infrastructure to trade and exposing the firm to new counterparty and liquidity risks.
As a result, at a time when interest in so-called alternative data is exploding, AHL already has a deep pool of proprietary information to draw on.
“We don’t own a satellite and we aren’t collecting our own satellite images. What we are doing is interacting with the market, and we’ve been meticulous in recording every point at which we’ve done that,” Sargaison says.
Streamed pricing
Through the firm’s Evolution funds, it trades credit default swaps, rates in markets outside the G7 and other more esoteric markets. AHL also takes in streamed pricing from more than a dozen different dealers in foreign exchange.
Data similar to this is becoming increasingly available from banks and exchanges, but Sargaison believes the firm has a good lead on competitors because of the way it has captured the information.
Publicly available pricing information typically reflects a consensus at a point in time, he says, but outlying data can be more revealing. “If you only go to Bloomberg for the current price in a particular market, you’re only getting one data point. More data points are good,” he says.
With developed markets trending weakly, AHL’s decision to venture into new markets looks far-sighted for other reasons too.
For CTAs, the past year has been a time when the trend was nobody’s friend. Societe Generale’s CTA index is barely positive for the year to date at the time of writing, while the bank’s trend indicator, based on a simple momentum strategy, is down 13%.
Despite the adverse conditions, AHL’s main trend-following fund was up 6% for the 12 months to October. The standout performance, though, has come from Evolution and Evolution Frontier: up 16.5% and 15.2%.
The price of electricity in Germany over the next week has nothing to do with whether we think rates are going up or down. It has mostly to do with the current price of coal and whether the weather is going to be slightly hotter or colder than expected
Matthew Sargaison, Man AHL
The latter two were an attempt to find less-trodden turf. While central banks have kept rates squashed at low levels in developed markets since the financial crisis, limiting the range potential of trends, emerging market rates have followed a different path. Brazilian central bank rates have been through three cycles in the time it has taken the US Federal Reserve to raise rates by 0.5%, Sargaison points out.
In AHL’s first venture into this space – its Evolution programme – the firm started trading interest rate swaps in less-developed economies. With its second venture – Evolution Frontier – it began trading assets as obscure as Kazakh currency and cheese.
The idea was to diversify, says Sargaison. “Momentum is a relatively weak signal at a single market level, but we see it almost everywhere. In every asset class we’ve ever looked at, we see evidence of price trends being more than just Brownian motion.”
Man AHL’s managers believed the more markets it could trade, the higher its risk-adjusted returns could be. “You are trying to diversify away some of the downside risk,” Sargaison explains.
European power markets are not driven by what’s going on at the Federal Reserve, for example, he says. “The price of electricity in Germany over the next week has nothing to do with whether we think rates are going up or down. It has mostly to do with the current price of coal and whether the weather is going to be slightly hotter or colder than expected.”
AHL has added new markets to these programmes every year since inception, but equally has rejected some, such as Australian electricity, where those markets are dominated by too few active players. To guard against liquidity risk, the firm limits the size of its positions relative to the average daily trading volume in a market. This makes life difficult in smaller markets, and the Evolution funds are now closed to new investors, having reached capacity.
So, what does AHL do next? Its focus on machine learning is a large part of the answer.
Sargaison is aiming for everybody at the firm to be fluent in the use of machine learning techniques. The firm is installing new hardware to do back-propagation training for convolutional neural networks – a type of machine learning used in image recognition and a hot area of exploration for hedge funds right now.
But it’s not all about flashy technology, and the firm’s approach is far from gung-ho. Four years ago, AHL tested its first machine learning-based model with proprietary money for a year before going live and built diagnostic tools to help grasp how the model “chose” to behave in different scenarios, Sargaison says. “We needed to get comfortable that we understood what it was really doing.”
And today he worries that machine learning becomes the assumed solution to every problem. “It’s about knowing which techniques to apply where, which ones are most likely to lead to fruitful analysis,” he says. “It shouldn’t be the hammer for every nail.”
This article has been updated to clarify AHL’s practice relating to position sizes relative to market liquidity.
A problem solved
Like all investors, AHL faces a problem knowing where best to execute its trades. That problem has got more complicated with the development of increasing numbers of execution algorithms.
Firms typically spread trading across multiple execution routes to get a sense of where results are best and send the bulk of their orders that way. But the question is how best to play the trade-off between testing the options and picking the best ones.
“If you see an edge, you should be exploiting it. But you still need to explore to find out if there is a better edge to be found,” Sargaison says.
AHL’s insight was to recognise this as a mathematical problem, and one with a known solution.
The so-called ‘multi-arm bandit’ problem – the name refers to playing multiple slot machines with unknown but differing payoffs – was famously considered by mathematicians during World War II in a bid to deploy resources effectively.
One joke was that it so sapped the minds of Allied analysts it should be dropped over enemy territory as the ultimate instrument of intellectual sabotage.
In fact, the problem had been solved by William Thompson in 1933, but the solution was so computationally intensive it had been ignored. Only in recent years has computer power reached the point where Thompson’s sampling algorithm can be employed.
Thompson’s algorithm creates a distribution of probabilities as to which is the best bandit to play based on the evidence so far. It then allocates cash to each machine in line with those probabilities. Based on the outcome, it updates the probabilities.
Only users who have a paid subscription or are part of a corporate subscription are able to print or copy content.
To access these options, along with all other subscription benefits, please contact info@risk.net or view our subscription options here: http://subscriptions.risk.net/subscribe
You are currently unable to print this content. Please contact info@risk.net to find out more.
You are currently unable to copy this content. Please contact info@risk.net to find out more.
Copyright Infopro Digital Limited. All rights reserved.
As outlined in our terms and conditions, https://www.infopro-digital.com/terms-and-conditions/subscriptions/ (point 2.4), printing is limited to a single copy.
If you would like to purchase additional rights please email info@risk.net
Copyright Infopro Digital Limited. All rights reserved.
You may share this content using our article tools. As outlined in our terms and conditions, https://www.infopro-digital.com/terms-and-conditions/subscriptions/ (clause 2.4), an Authorised User may only make one copy of the materials for their own personal use. You must also comply with the restrictions in clause 2.5.
If you would like to purchase additional rights please email info@risk.net
More on Awards
Clearing house of the year: LCH
Risk Awards 2025: LCH outshines rivals in its commitment to innovation and co-operation with clearing members
Best use of machine learning/AI: CompatibL
CompatibL won Best use of machine learning/AI at the 2025 Risk Markets Technology Awards thanks to its ground-breaking use of LLMs for automated trade entry, redefining speed and reliability in what-if analytics
Markets Technology Awards 2025 winners’ review
Vendors jockeying for position in this year’s MTAs, as banks and regulators take aim at counterparty blind spots
Equity derivatives house of the year: Bank of America
Risk Awards 2025: Bank gains plaudits – and profits – with enhanced product range, including new variants of short-vol structures and equity dispersion
Law firm of the year: Linklaters
Risk Awards 2025: Law firm’s work helped buttress markets for credit derivatives, clearing and digital assets
Derivatives house of the year: UBS
Risk Awards 2025: Mega-merger expected to add $1 billion to markets revenues, via 30 integration projects
Interest rate derivatives house of the year: JP Morgan
Risk Awards 2025: Steepener hedges and Spire novations helped clients navigate shifting rates regime
Currency derivatives house of the year: UBS
Risk Awards 2025: Access to wealth management client base helped Swiss bank to recycle volatility and provide accurate pricing for a range of FX structures