Journal of Computational Finance
ISSN:
1460-1559 (print)
1755-2850 (online)
Editor-in-chief: Christoph Reisinger
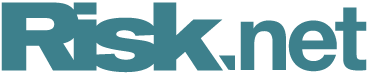
The probability of backtest overfitting
David H. Bailey, Jonathan M. Borwein, Marcos López de Prado and Qiji Jim Zhu
Need to know
- A general framework for modeling the IS and OOS performance using probability is proposed.
- Results indicate that CSCV provides reasonable estimates of PBO, with relatively small errors.
- CSCV provides both a new and powerful tool in the arsenal of an investment and financial researcher.
Abstract
ABSTRACT
Many investment firms and portfolio managers rely on backtests (ie, simulations of performance based on historical market data) to select investment strategies and allocate capital. Standard statistical techniques designed to prevent regression overfitting, such as hold-out, tend to be unreliable and inaccurate in the context of investment backtests. We propose a general framework to assess the probability of backtest overfitting (PBO). We illustrate this framework with specific generic, model-free and nonparametric implementations in the context of investment simulations; we call these implementations combinatorially symmetric cross-validation (CSCV). We show that CSCV produces reasonable estimates of PBO for several useful examples.
Copyright Infopro Digital Limited. All rights reserved.
As outlined in our terms and conditions, https://www.infopro-digital.com/terms-and-conditions/subscriptions/ (point 2.4), printing is limited to a single copy.
If you would like to purchase additional rights please email info@risk.net
Copyright Infopro Digital Limited. All rights reserved.
You may share this content using our article tools. As outlined in our terms and conditions, https://www.infopro-digital.com/terms-and-conditions/subscriptions/ (clause 2.4), an Authorised User may only make one copy of the materials for their own personal use. You must also comply with the restrictions in clause 2.5.
If you would like to purchase additional rights please email info@risk.net