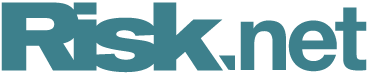
Paper of the year: JD Opdyke
Operational Risk Awards 2015: New technique may help limit errors in AMA capital estimates

Working out the amount of regulatory capital to set aside against operational risk losses can be almost as big a challenge as preventing op risk losses in the first place. Like several previous winners, this year's paper of the year addresses problems relating to the estimation of op risk capital levels. Author JD Opdyke – now head of operational risk modelling at US financial services firm GE Capital – co-wrote a paper on estimating op risk capital that won this award in 2013.
He describes his latest paper, Estimating operational risk capital with greater accuracy, precision and robustness – published in the Journal of Operational Risk in December 2014 – as very much a sequel to his earlier work. "I never like to bemoan challenges without coming up with a solution, so coming up with one was [one] goal of this paper," he says.
Those challenges were three critical flaws in the op risk capital estimates derived by banks using methods such as the maximum likelihood estimation (MLE) technique to comply with the advanced measurement approach (AMA) to Basel rules. In 2011, Opdyke and co-author Alexander Cavallo, head of operational risk and risk analytics at Northern Trust in Chicago, showed that MLE-derived capital figures could be highly inflated, highly variable and fragile in the face of non-standard distributions of data. They traced the upward bias to a statistical phenomenon known as Jensen's inequality, which states that, for a convex function, the expected value of all outputs will be substantially higher than the output when the expected value of the input alone is put through the function.
Because of the way it is calculated, op risk capital is particularly susceptible to Jensen's inequality, Opdyke says. There are three things that determine when the effect becomes material. The first of these is the size of the variance of the severity parameter estimate, which is largely driven by sample size. "If your sample size is 1,000 or greater, it is unlikely that you will see much bias at all," he explains. "Unfortunately most [op risk] units of measure have sample sizes much smaller than 1,000 or even 500. They have 50, 100 or 200, and that is where this bias will really kick in."
The second is the heaviness of the severity distribution tail: the heavier the tail, the more bias there will be, notes Opdyke. Typically, the distributions used for op risk capital estimation are extremely heavy-tailed, making this problem worse. "The heavier the tail, the more bias you will see, because the more convex the quantile function," he says.
The third is the percentile probability of the loss being estimated: a higher percentile means a greater exposure to bias. Op risk models seek to estimate a one-in-a-thousand annual loss, or the 99.9 percentile – a "very high" level, says Opdyke.
Reduced-bias capital estimator
Opdyke's solution to this upward bias in op risk capital estimates is a new method of estimation, the reduced-bias capital estimator (RCE). Starting with an estimate derived using a conventional method such as MLE, the method determines the convexity of the value-at-risk as a function of the severity parameters by systematically perturbing the parameter values; the median of the corresponding capital estimates is divided by the mean, and the original estimate is then scaled down by a function of this ratio to reverse the biasing effects of convexity. The greater the convexity, the greater the upward bias produced by Jensen's inequality, and so the greater the factor by which the original estimate is scaled down.
Basing the RCE on the established loss-distribution approach (LDA), and proving that it worked with MLE – the de facto industry standard for AMA banks – was critical, Opdyke says. "I didn't want this solution to be in conflict with the spirit or the letter of the LDA framework, but rather consistent with this framework, so that use of RCE would not change an LDA implementation at all," he says. "It essentially just adjusts the capital estimate that is generated after the fact, so we right-size the capital estimate to get the true 99.9 percentile on average. Nothing really changes in terms of implementation; it just requires a few additional steps."
RCE is not just applicable to LDA though, and could work with other estimation techniques as well. In fact, Opdyke argued in 2012 that the optimally bias-robust estimation technique could do a better job than MLE in terms of robustness, continuing to produce usable estimates even when the data used as an input was not independent and identically distributed. But the problem of convexity, which causes the upward bias of the eventual capital estimate, is common to every estimation method.
Opdyke says the RCE also reduces the variance of capital estimates – something that is potentially as serious a problem as their upward bias, he argues. Jensen's inequality is also largely to blame here as well: he cites empirical evidence that convex functions such as VAR produce estimates that are not only biased upwards, but far more variable. This means that using RCE has the potential to provide a more precise – as well as a less biased – capital figure. Compared with using MLE alone, Opdyke says variance is usually cut by 30–50% through the use of RCE.
"The empirical 95% confidence intervals, which are embarrassingly large for MLE, are much smaller for RCE, on average only two-thirds the size across both the regulatory and economic capital results," noted Opdyke in his December 2014 paper. "In one-eighth of all cases, they are less than half the size of those of MLE."
Room for improvement
Even though Opdyke describes the improvement in precision as "a pretty big win", he acknowledges that there is still room for improvement. The paper gives the example of a highly variable MLE-based estimation which yields a root-mean-square error of $8.1 billion. That figure is reduced to just $3.3 billion by using the RCE approach. But the paper noted this was "still a very high number ... and decreasing it further is vitally important".
In addition, RCE shows some improvement in robustness – reducing the extent to which capital estimates veer off the mark when the data underlying them is not independent and identically distributed. The textbook assumption is that the entire data-set is made up of points drawn independently from a single distribution, such as a lognormal or generalised Pareto distribution. Yet this does not generally hold true in real life, says Opdyke.
"We do our best to define units of measure so that our data is as homogenous as possible, but ... in reality, everyone realises that it is a necessary but mathematically convenient assumption," he says. "So the issue is: in the real world, how does your capital behave when you contaminate all lognormal data with data from other distributions? On that front the estimator does better, but more modestly so."
Precision and accuracy can be increased by using more data points, with sample sizes in the thousands producing minimal distortion compared with smaller ones – as demonstrated by Opdyke and other researchers. "When the sample size increases to a thousand-plus, the bias disappears, and that's because the variance of the estimator shrinks dramatically, and then there isn't much room for the bias to occur, because we are getting so close to the right answer that we can't have much bias due to the estimator bouncing around," he says. "It's only when sample sizes are small and the estimate bounces around a lot that the mean is pulled up and we can get very large bias."
Unfortunately, though, the individual units of measure used in op risk calculations rarely have such large numbers of data points. Furthermore, very high-quantile estimates (0.99999 and higher) require not thousands but millions of data points to achieve reasonable precision. That would equate to "50,000 to 100,000 years' worth of loss data", says Opdyke – a challenge currently out of reach, even for state-of-the-art applied statistics.
A formal mathematical paper that points to Jensen's inequality as being at the root of the bias in op risk capital estimates, written by Opdyke and former colleague Kirill Mayorov, is intended for publication in 2016. Opdyke hopes that other authors will build on the foundations of the RCE to improve capital estimation techniques still further. The possibility that the Basel Committee on Banking Supervision may carry out a fundamental overhaul of the AMA has opened a "window of opportunity" for methods such as RCE, he says. Neither the AMA nor the LDA should be discarded, he thinks; both represent a great deal of work and constitute "what is generally an impressive, risk-sensitive framework".
Although any reform effort will be challenging, Opdyke believes regulators and practitioners must do what they can to produce a more accurate and risk-sensitive method of estimating op risk capital. "I would love to see the floodgates open, and I hope that this RCE paper – along with my forthcoming paper providing in-depth analytical evidence of Jensen's inequality – will help open them and get people focused on solutions to get the capital distribution right-sized," he says. "Scrutiny of the three characteristics of this distribution – unbiasedness, precision and robustness – must drive the research going forward."
Disclaimer: The views and opinions expressed by JD Opdyke in this article, including any processes and analyses and any assumptions on which they are based, are his own and do not reflect the views of General Electric
Only users who have a paid subscription or are part of a corporate subscription are able to print or copy content.
To access these options, along with all other subscription benefits, please contact info@risk.net or view our subscription options here: http://subscriptions.risk.net/subscribe
You are currently unable to print this content. Please contact info@risk.net to find out more.
You are currently unable to copy this content. Please contact info@risk.net to find out more.
Copyright Infopro Digital Limited. All rights reserved.
As outlined in our terms and conditions, https://www.infopro-digital.com/terms-and-conditions/subscriptions/ (point 2.4), printing is limited to a single copy.
If you would like to purchase additional rights please email info@risk.net
Copyright Infopro Digital Limited. All rights reserved.
You may share this content using our article tools. As outlined in our terms and conditions, https://www.infopro-digital.com/terms-and-conditions/subscriptions/ (clause 2.4), an Authorised User may only make one copy of the materials for their own personal use. You must also comply with the restrictions in clause 2.5.
If you would like to purchase additional rights please email info@risk.net
More on Awards
Trading systems: structured products/cross-asset – Murex
Murex won the Trading systems: structured products/cross-asset award at the 2025 Risk Markets Technology Awards for its MX.3 platform, praised for its flexibility and advanced analytics
Best vendor for system support and implementation: Murex
Murex has won the Best vendor for system support and implementation at the Risk Markets Technology Awards, recognised for its innovative MX.3 platform, exceptional client support and seamless implementation services
FRTB-IMA product of the year: Murex
Murex wins FRTB-IMA product of the year for its advanced, scalable MX.3 platform enabling seamless regulatory compliance
Pricing and analytics: equities – Finastra
Finastra’s Sophis platform wins the Risk Markets Technology Award for Pricing and analytics in equities, recognised for its robust capabilities in equities and derivatives trading
Best execution product of the year: Tradefeedr
Tradefeedr won Best execution product of the year for its API platform, which standardises and streamlines FX trading data, enabling better performance analysis and collaboration across financial institutions
Collateral management and optimisation product of the year: LSEG Post Trade
LSEG Post Trade wins Collateral management and optimisation product of the year for interconnected services that help mitigate counterparty risk and optimise capital usage
Clearing house of the year: LCH
Risk Awards 2025: LCH outshines rivals in its commitment to innovation and co-operation with clearing members
Driving innovation in risk management and technology
ActiveViam secured three major wins at the Risk Markets Technology Awards 2025 through its commitment to innovation in risk management and technology